9 Toward a Regional Field Phenotyping Network in West Africa
Alain Audebert, CIRAD, France & ISRA/CERAAS, Senegal
Delphine Luquet, CIRAD, France
Vincent Vadez, IRD, Senegal & ISRA/CERAAS, Senegal
Daniel Foncéka, CIRAD & ISRA/CERAAS, Senegal
Ndjido Ardo Kane, ISRA/CERAAS, Senegal
Abstract
Phenotyping plays an important role in crop science and breeding programs. The accurate, rapid acquisition of phenotypic information on plants in different environments helps researchers explore the genomes’ inheritance and expression patterns and determine the association of genomic and phenotypic information and ultimately increase crop yields. Traditional methods for acquiring crop traits (e.g., plant height, biomass, yield, etc.) rely on manual sampling, which is laborious and time-consuming. Fortunately, technological advances are resolving the high-throughput phenotyping bottleneck. To optimize the benefit of breeding programs in West Africa (WA) on the sustainability and performance of cropping systems (considering climate change and agroecological transition) developing high throughput field phenotyping methods is essential. However, the establishment of this type of activity requires infrastructure, equipment, management, and most importantly, dedicated and trained staff. Also, new technologies like Unmanned Aerial Vehicles (UAV) have recently become an important tool for fast and non-destructive high throughput phenotyping. This technology is flexible, practical, and provides easy access to data and high spatial resolution. UAVs are powerful tools for phenomics and genomics studies. As such, CERAAS and its partners are developing a field phenotyping network in the West African sub-region—the general scheme of which is presented below.
Keywords: Breeding, Field phenotyping, High-throughput, Regional network, West Africa
Introduction
Crop production must double by 2050 to respond to predicted demands of the global population (Ray et al., 2013). To meet this demand, crop yields should be increased by 2.4% annually, but currently, the average rate of yield increase is only 1.3%, with yields stagnating in up to 40% of the land under cereal production (Fischer & Edmeades, 2010). To ensure improved agricultural productivity, developing new varieties that are well adapted to specific environments is one of the main avenues for agricultural research centers. An effective approach to achieve this objective is the design of marker-assisted breeding programs which requires scientists to better understand the connection between a plant’s observable characteristics (phenotype) and its genetic makeup (genotype). By establishing the connection between genotype and phenotype, it is possible to select high-yielding stress-tolerant plants and improve agricultural production to satisfy the requirements of the growing human population (Li et al., 2014; Thorp et al., 2015, 2018; White et al., 2012).
In the last two decades, genome sequencing of crops has proceeded at a rapid pace, but the translation of these data into the identification of desirable traits has been constrained by the lack of knowledge of the associated phenotypes (Furbank & Tester, 2011; Zaman-Allah et al., 2015). Besides, marker assisted breeding programs often require working with large mapping populations (more than 250 lines in most cases). Depending on the experimental design used or the number of treatments applied, phenotyping may require thousands of individual plots to be measured. To relieve this bottleneck and to fully benefit from the available genomic information, reliable, automatic, multifunctional, and high-throughput phenotyping platforms should be developed to offer plant scientists new insight into all the aspects of living plants. In recent years, high-throughput phenotyping platforms (HTPPs) have been developed (Araus & Cairns, 2014; Yang et al., 2013). However, most of these are fully automated facilities in greenhouses or growth chambers, equipped with a precise environmental control. Although HTPPs enable the capture of detailed, non-invasive information throughout the plant life cycle, the results from controlled environments are distinct from the actual situations that plants will experience in the field, making it difficult to extrapolate data from controlled environments to the field. As a regional center of excellence that focuses on the genetic improvement of dryland crops (e.g., sorghum, pearl millet, fonio, groundnut, cowpea and sesame), CERAAS/ISRA proposes to engage the breeding community in the development of a field phenotyping network of testing sites. The goal is to provide a rich ground for interactions across disciplines in order to develop improved varieties and management packages adapted to specific stress scenarios and sensitive to socio-cultural contexts, and hence address the multiple complex challenges facing plant breeding in West Africa. Family farming is essential, and it must meet an increasing food demand in diverse and changing agro-climatic environments and in connection with changing agricultural sectors (i.e., food, non-food agricultural products, seeds). This chapter’s objective is to propose a roadmap to associate different technologies—varying from manual measurements to image analysis and UAV onboard sensors—in a field phenotyping network in the Sahelian sub-region.
1. Institutional Positioning
CERAAS, Centre d’Études Régional pour l’Amélioration de l’Adaptation à la Sécheresse, is a research center of ISRA (Institut Sénégalais de Recherche Agronomique) specialized in drought adaptation studies. In 2018, CERAAS was elevated from a National Center of Specialization to an ECOWAS Regional Centre of Excellence (RCE) on dryland cereals (e.g., millet, sorghum and fonio) and associated crops (e.g., peanut, cowpea and sesame). The RCE is a joint entity between CERAAS, two other centers of ISRA (the Bureau d’Analyse Macroéconomique – BAME and the Centre National de Recherche Agronomique – CNRA), the Institut de Technologie Alimentaire (ITA) and the Ecole Nationale Supérieure d’Agronomie (ENSA-University of Thies). The primary mandate of RCE is to lead research on suitable drylands-cereal technologies and varieties in the West and Central African region.
With its historical partner, CIRAD (Centre de Coopération Internationale pour la Recherche en Agronomie pour le Développement), CERAAS is running a partnership network dedicated to innovation in crop improvement in Western Africa (dP-IAVAO which stands for dispositif en partenariat-Innovation et Amélioration Variétale en Afrique de l’Ouest, in French). The proposal of the dP-IAVAO is to rethink varietal improvement that has so far focused mainly on specialized agricultural and social systems. The aim is to recognize the plurality of agricultural systems and corresponding varietal demands, and to mobilize more disciplines and advanced tools towards more agile breeding programs that respond to the diversity and complexity of these agricultural systems.
Interdisciplinarity is key to this proposal, and it needs to be effectively integrated into innovative breeding programs. The scientific vision of the dP-IAVAO is thus structured around four research domains involving multiple disciplines and constitutes a platform fostering dialogue and interactions between its components:
– Understand the crop improvement system. This first domain aims at better describing the different components of the agri-food system in which crop improvement operates.
– Genetic analysis and crop improvement. This domain, which constitutes the historical heart of crop improvement, aims to mobilize modern genetic tools to explore the diversity and complexity of agricultural systems.
– Variety adoption and innovation management. The effective implementation of redefined breeding objectives and the creation of corresponding varieties provides the subject of an iterative analysis on the adoption and dissemination of this new diversity within traditional diversity.
– Partnership modalities and research practices. The context in which life sciences research operates has become significantly more complex over the past 30 years: international regulations on access to and sharing of genetic resources have evolved considerably, and at the same time, research products are increasingly subject to intellectual property constraints related, for example, to the development of the seed sector and biotechnology.
The dP-IAVAO was also part of an evolving West African partnership context with a new research structure led by CORAF and supported by WAAPP/PPAAO, which aims at the emergence of Regional Centers of Excellence (RCE). The dP-IAVAO, which is based on existing research facilities and projects, is improving coordination at the regional level, but also guiding the design of new flagship projects and the development of infrastructures and technical capacity. Education and training are also an essential component of the dP-IAVAO as it contributes to the training of a new generation of plant breeders.
The geographical boundaries of the dP-IAVAO include the Sahelian and Sudanese (200 – 1200 mm) areas of West Africa where the cultivation of dry cereals such as millet, sorghum, fonio, and their associated crops such as cowpea, groundnut, and sesame is predominant. In this area, several breeding programs working on these species, notably from Senegal, Mali, Burkina Faso, and Niger, are involved in projects focused on innovative approaches for crop improvement (participatory selection, marker-assisted selection, etc.). However, other countries located in these areas, (Chad, Guinea, Cameroon, Côte d’Ivoire, Ghana, Togo, and Benin) and with research activities on one or more of the crops listed above, are also associated with the dP to develop collaborations on specific topics, in particular field phenotyping and training activities. The central theme of the dP-IAVAO is therefore the integration of a multidisciplinary approach in the design and effective implementation of innovative varietal breeding programs in response to the complexity of the agro-ecological systems encountered in West Africa. In terms of design, the breeding program is considered an integration place enabling the production of genetic materials responding to the demand.
2. High-throughput Phenotyping Methods
The crop phenotype is an expression of the genotype (G) and the environment (E) in which it grows. Crop phenotyping generally includes agro-morphological traits that provide indications on the dynamics of yield establishment and its genotypic and environmental variability (e.g., plant height, counting LAI, lodging, crop canopy cover), canopy spectral texture (i.e., spectral features), physiological traits (e.g., chlorophyll, biomass, pigment content, photosynthesis), abiotic/biotic stress indicators (e.g., stomatal conductance, canopy temperature difference, leaf water potential, senescence index), nutrients (e.g., nitrogen concentration, protein content), and yield. Field phenotyping is a critical component of plant breeding programs, as the ultimate expression of the genetic factors, environmental factors, and their interaction on critical production traits, such as yield potential and tolerance to abiotic/biotic stresses (Araus & Cairns, 2014; Neilson et al., 2015). Field phenotyping is increasingly recognized as a key approach capable of delivering the required throughput and an accurate description of trait expression in real-world cropping systems. However, for a higher precision, the performances of breeding materials must be evaluated through a wide range of environmental conditions (González-Dugo et al., 2015; González-Recio et al., 2014; Rahaman et al., 2015).
Traditionally, phenotyping was done manually, and it was time consuming and expensive. To move towards high throughput methods, new phenotyping approaches relied largely on imaging technologies. Different methodological approaches that use spectral reflectance, canopy temperature by thermal imaging, and visible light imaging have been proposed to evaluate phenotypic traits in the field (Araus & Cairns, 2014). The agro-morphological traits of a crop can be estimated using an RGB camera (visible) by building the digital surface model (DSM) or the digital elevation model (DEM) and by conducting image classification analysis, which can be used to estimate the plant height, the proportion of lodging area, seedling emergence, etc. (Bendig et al., 2015; Hunt et al., 2005, 2010; Li et al., 2015). The absorption and reflectance characteristics of crops can be used to calculate vegetation indices related to agro-morpho-physiological traits (Nigon et al., 2015; Øvergaard et al., 2010; Swain et al., 2010). For instance, the temperature of the canopy is closely related to the cooling capacity of the plant, which depends on the transpiration of the crop, itself reflecting the plant water potential, stomatal conductance, etc., under stress or non-stress conditions. Crop yield can be predicted using proxies based on the combination of hyperspectral and thermal infrared data (Berni et al., 2009; González-Dugo et al., 2015).
Currently, advanced field-phenotyping platforms use high throughput ground wheeled or aerial vehicles deploying multiple types of sensors to measure plant traits on a timescale of a few seconds per plot. Although phenotyping with ground vehicles such as phenomobiles is extremely precise, the process can be time-consuming if there are too many plots to phenotype (Cobb et al., 2013; Zhang and Kovacs, 2012). Therefore, this approach would be unsuitable for an African cross-regional network due to the cost, heavy maintenance, transport, and lack of maneuverability.
Satellite imaging technologies have become extremely useful when collecting data for various agricultural applications (Li et al., 2014; Sankaran et al., 2015). However, the major limitations of using the currently available satellite sensors are the high cost, the lack of spatial resolution for the identification of desirable traits, the risk of cloud cover, and long revisit periods (Gevaert et al., 2015; Han-Ya et al., 2010). For breeding programs, the resolution of the satellite sensor is still not enough to compare individual lines or plants. In contrast, UAVs have demonstrated capabilities for large-scale monitoring of crop condition due to the high spatial and spectral resolutions of the sensors.
In recent years, the use of UAVs has increased considerably in agricultural research programs due to the drop in prices, the miniaturization, the higher resolution of sensors, the ease of use (piloting, programming of flight plans), the improvement of computing (image analysis) and its strong potential for applications in breeding programs (Ballesteros et al., 2014; Berni et al., 2009; Candiago et al., 2015; Chapman et al., 2014; Gómez-Candón et al., 2013; Hunt et al., 2005; Liebisch et al., 2015; Zhang & Kovacs, 2012). This technology provides a low-cost approach to meet the critical requirements of spatial, spectral, and temporal resolutions. To assess the precision and efficiency for field-based phenotyping in small plots by different remote sensing techniques, three remote sensing approaches including UAV, proximal sensing, and satellite-based imagery, were compared. This study demonstrated that the UAV-based remote sensing was the most suitable approach for acquiring canopy temperature and normalized difference vegetation index (NDVI) in breeding populations (Tattaris et al., 2016). Therefore, UAVs are becoming critical in the high-throughput phenotyping of many plots and field trials in a near real-time and dynamic manner. UAVs can be used to execute autonomous tasks through the use of radio remote control equipment and an auto-control system, which can be divided into several types according to the flight mode (Sankaran et al., 2015). Field phenotyping platforms that use UAV tools have a higher potential thanks to the development and use of adapted sensors such as digital cameras, multispectral cameras, hyperspectral sensors, and infrared thermal imagers, which are commonly deployed UAV-RSP sensors. With advances in miniaturization, new sensors based on light detection and ranging (LiIDAR) are upcoming. The main applications of these sensors for breeding programs include the use of visible imaging for canopy surface modeling, crop height, and biomass estimation (Diaz-Varela et al., 2014; Mathews & Jensen, 2013; Zarco-Tejada et al., 2014); the use of visible–near-infrared spectroscopy to identify physiological status (Nigon et al., 2015; Overgaard et al., 2010; Sugiura et al., 2005; Swain et al., 2010); thermal imaging to detect water stress (González-Dugo et al., 2013, 2014); LIDAR cloud point to measure plant’s fine-scale geometric parameters with high precision (Wallace et al., 2012); and microwave imaging to estimate soil moisture and canopy structure parameters by combining different spectral bands (Acevo-Herrera et al., 2010; Han-Ya et al., 2010).
3. Network Construction
At the national level, the field phenotyping network relies on three levels. The first level is centered on fine phenotyping. The second level is focused on varietal evaluation in different environments, while the third level consists of evaluations in farmers’ fields. In addition to the breeding aspect, the network is also of significant interest for all research and development activities in agronomy. The diagram presented in Figure 1 lists the possible research actions at the different levels.
Figure 1
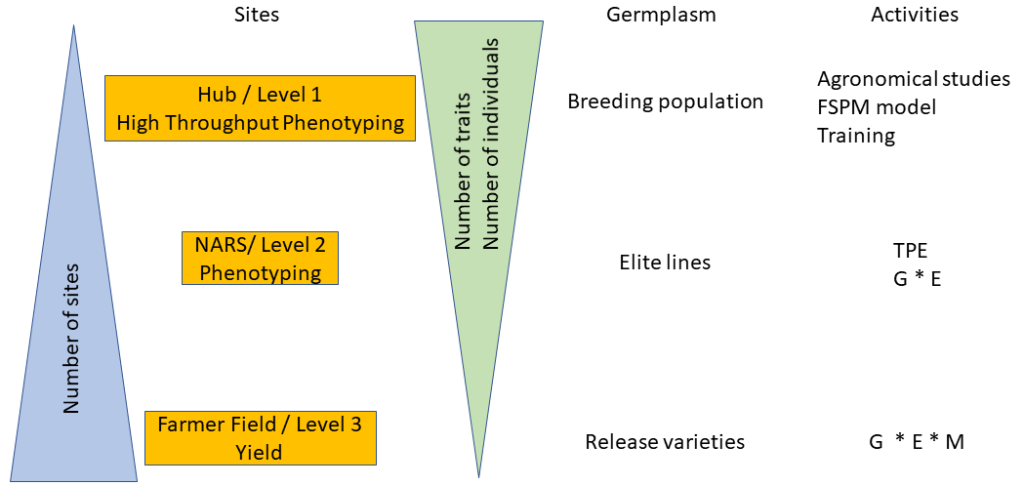
Level 1: The fine phenotyping is done at a hub that is fully equipped and highly specialized to meet the needs of high throughput (i.e., the ability to conduct experiments with a large number of germplasms tested and to carry out precise measurements in a short time step). These representative high throughput phenotyping locations must also present all the guarantees to manage experiments with different agronomic treatments and should be selected based on environmental characterization, build on existing experimental sites that are functional, and back up the most advanced NARS (e.g., ISRA in Senegal, IER in Mali). This may require the development or rehabilitation of infrastructures like weather stations, drip irrigation systems, fencing, and modern data capture tools. There might be a scope for working in collaboration with the private sector at certain sites (e.g., Adventa or Corteva). The necessary equipment to carry out high-throughput phenotyping measurements should be available at these sites. Occasionally and depending on the resources, this equipment can be deployed to other sites (levels 2 and 3). These fine high-throughput phenotyping sites should also have all the advantages to conduct studies that aim to understand better crop adaptation mechanisms using functional-structural plant models. The development of infrastructures needs to be paired with a massive long-term training of students, technicians, and scientists/breeders (“multi-purpose breeders,” capable of doing both breeding and reliable phenotyping) in order to develop a sense of ownership. Hence, three sites were chosen as potential high throughput phenotyping hubs across the subregion. Each hub is representative of the diversity of climate and soil conditions in the region (Figure 2): Bambey (ISRA research station, Senegal; 14.710825° N; -16.483797° E), Sotouba (IER research station, Bamako, Mali; 12.659558° N; -7.924943° E) and Farako-ba (INERA research Station, Bobo Dioulasso, Burkina Faso; 11.094251° N; -4.332719° E).
Figure 2
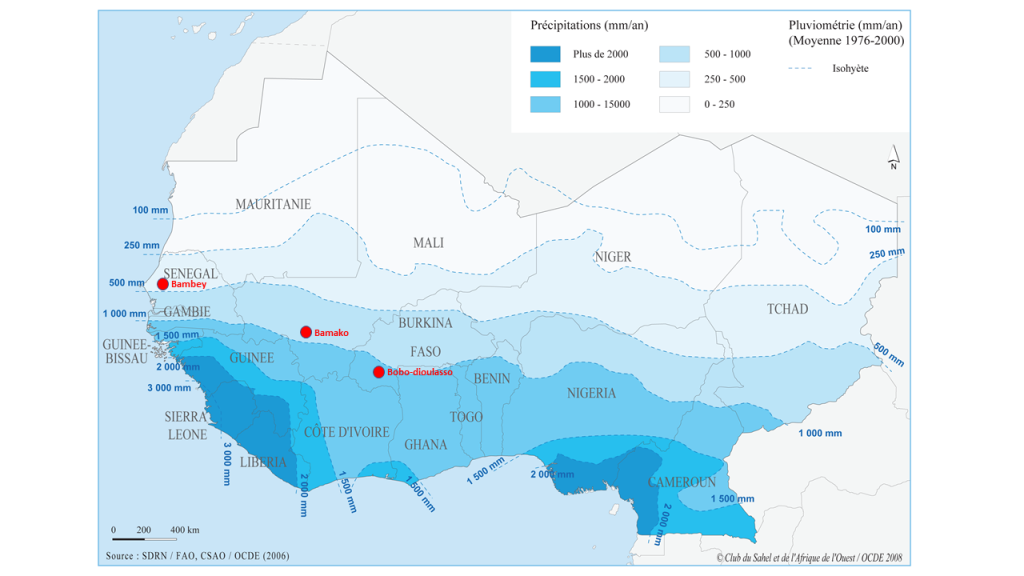
Level 2: This level is based on the national agricultural research sites, which generally form a good network throughout the country. The main objective of this network is to evaluate the performances of the breeding lines in different environments, including hot-spots for specific stresses and then to study genotype-environment interactions. Studies carried out at this level are of great importance to define the target population environment (TPE) at the national and regional levels. Robust crop simulation models exist that can be used to: (i) characterize climatic scenarios in all target regions (main TPE) and (ii) guide the choice of trait-management packages to optimize productivity locally, even in the context of different socio-cultural contexts. This work requires good quality agronomic data from the network of Level 2 sites. The proposed work would engage breeders in generating input data and knowledge for crop models in order to guide breeding decisions. Breeding programs would then have greater insight on breeding targets (i.e., an area with known and prevalent stress type, required traits and management options, as well as appropriate breeding approaches). Besides, enriching the analysis of TPE with a socio-cultural analysis of potential cropping system variants within the TPE is essential to better design technical solutions, in particular to target cultivar/management improvement, to local constraints.
Level 3: Farmers’ fields are used to test promising breeding lines that are foreseen for release in this production system. At this level, only yield and yield components will be measured. This level is characterized by uncontrolled crop management. Therefore, to have a good estimation of varieties’ performances, the number of farmer fields should be as large as possible to integrate the diversity of cropping conditions.
4. Data Management
Much of the power of this field phenotyping network is in the way the data it generates are connected across sites and years. An efficient data management system needs to be established to ensure that evaluation and breeding data are integrated and shared across sites, network levels, and breeding programs. CERAAS, as a partner of IBP (Integrated Breeding Platform), benefits from logistic support for the use of the BMS (Breeding Management System). BMS is a complete software suite designed to boost the efficiency of plant breeding programs. This database combines crop information management, data analysis and decision-support tools to conduct most routine breeding activities, including the integration of different levels of molecular marker use. In other words, BMS represents an ideal tool to integrate data across the regional field phenotyping network.
5. Field Phenotyping Methodologies Developed in the Field Phenotyping Network
Based on the capacities of each partner/country and their financial possibilities, the development of the field phenotyping network is not synchronous among participating West African countries. The fine phenotyping hubs (level 1) and the evaluation sites (level 2) are generally located in national agricultural research stations. These sites have been well characterized previously and soil maps are usually available. They are equipped with a functional weather station, agricultural equipment, and have local manpower familiar with the requirements of research activities. For some sites, a renewal of the equipment is in progress. The field phenotyping network is expected to double in the next few years with the integration of a near infrared spectroscopy (NIRS) network that will enable high-speed phenotyping in the field of biochemistry.
Currently, Senegal is the most advanced in setting up the network since level 1 (hub) and level 2 sites are operational. For Mali, the phenotyping equipment is missing. The purchase of the UAV, pilot training, as well as the use of the image processing pipeline, are all under consideration. Burkina-Faso is lagging behind mostly due to funding reasons. Its level 1 site meets all criteria, but it still needs to be equipped with an irrigation system and UAV equipment, while in the level 2 sites, some weather stations are missing.
Across the different projects that allowed the start of West African field phenotyping network, UAVs technical solution has been chosen as an optimal solution to deploy across sites and trials. In Senegal the equipment consists of a FeHexaCopterV2 hexaCopter UAV system (Flying Eye Ltd., Sophia Antipolis, France). This UAV can carry three cameras fixed on a two-axis gimbal to point vertically downward. The first camera was an RGB ILCE-6000 digital camera (Sony Corporation, New York, NY, USA) with a 6000×4000 pixels sensor equipped with a 60 millimeter (mm) focal length lens. To minimize the blurring effect and noise in the images, the camera was set on speed priority (1/1250sec) and fixed on 100 ISO. The second camera is the AIRPHEN multispectral camera (Hiphen, Avignon, France) equipped with a 4.2 mm focal length lens and acquiring 1280×960 pixels images. The AIRPHEN includes six individual sensors equipped with filters centered on 450, 530, 560, 675, 730 and 850 nm, with a spectral resolution of 10 nm. The third camera is an infrared thermographic camera Tau 2 (Flir system, Oregon, USA) with 19 mm focal length.
The flight plan is defined with MikroKopter-Tools software (MikroKopter, Germany) to cover the entire area to be mapped and ensure an 80% frontal and lateral overlap along the track. Flight speed and image triggering depend on the flight altitude.
Figure 3
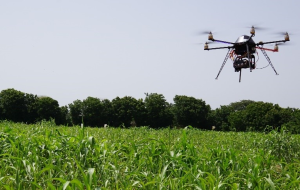
A semi-automatic image-processing pipeline developed by ISRA/CERAAS and CIRAD generates radiometrically calibrated and geometrically corrected multiband orthoimages using Agisoft PhotoScan digital photogrammetric software (PhotoScan Professional 1.4, Agisoft LLC, Russia) (See Part 2-Chapter 1). Real reflectance is computed using a radiometric reference target positioned to the ground and imaged at every UAV flight. This reference target is previously spectrally characterized in controlled conditions. Geometric correction is done using ground control points (GCPs) that have already been georeferenced. Orthorectification is then performed using GCPs to increase the accuracy of the generated orthoimages; vegetation indices can be extracted from the orthoimages. Using this UAV image processing pipeline, a good correlation between field measurements and proxies from the UAV images was observed in a study conducted during the 2019 cold dry season in Senegal which involved a sorghum panel (African population) of 202 entries (Gano et al., 2021) (Figure 4 and Figure 5). Similar correlation levels were observed on pearl millet and peanut populations (Diop et al., 2021) and calibration work is currently underway for cowpea.
Figure 4
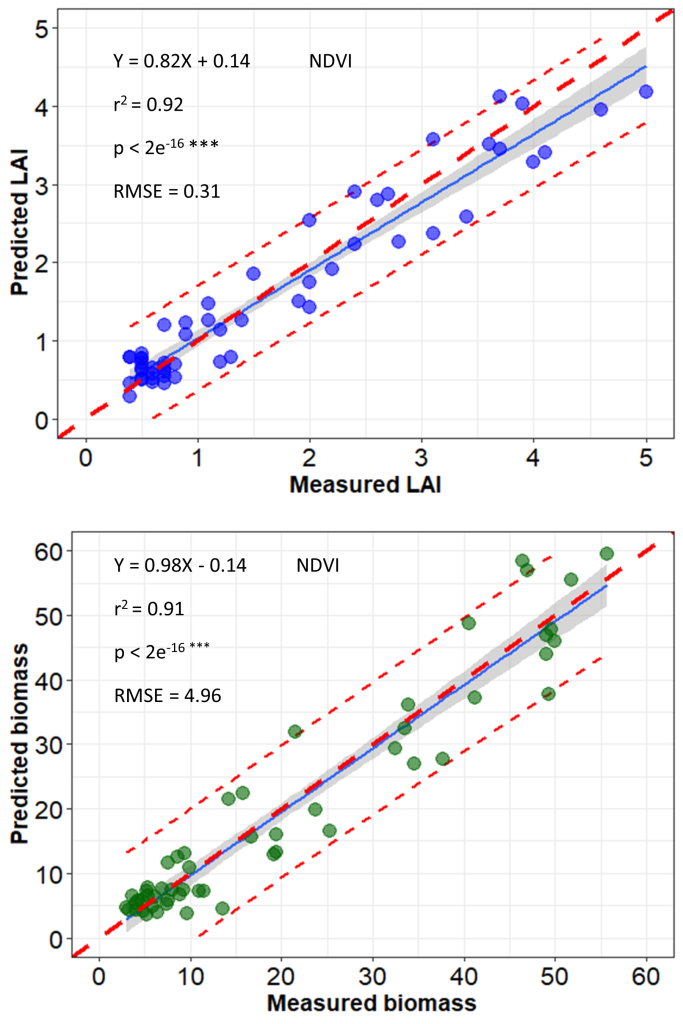
6. Training
The training activities are already underway in different countries of the phenotyping network. A significant number of students have carried out their internships in the field with the network researchers. Two postdocs worked on the UAV phenotyping platform in order to develop the image analysis pipeline. Two PhD students are finalizing their thesis, and since the onset of the project a total of 15 MSc students have been supervised. A training session on eco-physiological and biochemical phenotyping as well as crop modeling were organized within the network or by the network’s partners for PhD students, postdocs, and young scientists. It is expected that such training will be repeated over time in the framework of various projects. A sub-regional pilot contingent is already being built with two graduated pilots in Senegal.
Conclusion
The field phenotyping network initiated in 2016 is now in its second phase of construction. The activities in Senegal are ahead of schedule, but there is little doubt that other countries participating in the network will catch up. The drone-borne imaging phenotyping methodology is now functional for sorghum and millet culture but still under construction for peanut. The existing capacities meet the requirements of field high-throughput phenotyping and for training. The field phenotyping network is being rapidly strengthened by a biochemical phenotyping network with the deployment of NIRS equipment. Accordingly, West African crop improvement programs will become autonomous for the acquisition and analysis of phenotypic data in the fields of biochemical and morpho-physiological traits, thus meeting the requirements to better support breeding programs in their goal of contributing to the food security of future generations.
References
Acevo-Herrera, R., Aguasca, A., Bosch-Lluis, X., Camps, A., Martínez-Fernández, J., Sánchez-Martín, N., & Pérez-Gutiérrez, C. (2010). Design and first results of an UAV-borne L-band radiometer for multiple monitoring purposes. Remote Sensing, 2(7), 1662–1679.
Araus, J. L., & Cairns, J. E. (2014). Field high-throughput phenotyping: The new crop breeding frontier. Trends in Plant Science, 19(1), 52–61.
Ballesteros, R., Ortega, J. F., Hernández, D., & Moreno, M. A. (2014). Applications of georeferenced high-resolution images obtained with unmanned aerial vehicles. Part I: Description of image acquisition and processing. Precision Agriculture, 15(6), 579–592.
Bendig, J., Yu, K., Aasen, H., Bolten, A., Bennertz, S., Broscheit, J., Gnyp, M. L., & Bareth, G. (2015). Combining UAV-based plant height from crop surface models, visible, and near infrared vegetation indices for biomass monitoring in barley. ITC Journal, 39, 79–87.
Berni, J., Zarco-Tejada, P. J., Suárez, L., & Fereres, E. (2009). Thermal and narrowband multispectral remote sensing for vegetation monitoring from an unmanned aerial vehicle. IEEE Transactions on Geoscience and Remote Sensing, 47(3), 722–738.
Candiago, S., Remondino, F., De Giglio, M., Dubbini, M., & Gattelli, M. (2015). Evaluating multispectral images and vegetation indices for precision farming applications from UAV images. Remote Sensing, 7(4), 4026–4047.
Chapman, S., Merz, T., Chan, A., Jackway, P., Hrabar, S., Dreccer, M., Holland, E., Zheng, B., Ling, T., & Jimenez-Berni, J. (2014). Pheno-copter: A low-altitude, autonomous remote-sensing robotic helicopter for high-throughput field-based phenotyping. Agronomy, 4(2), 279–301.
Cobb, J. N., DeClerck, G., Greenberg, A., Clark, R., & McCouch, S. (2013). Next-generation phenotyping: Requirements and strategies for enhancing our understanding of genotype–phenotype relationships and its relevance to crop improvement. Theoretical and Applied Genetics, 126(4), 867–887.
Diaz-Varela, R. A., Zarco-Tejada, P. J., Angileri, V., & Loudjani, P. (2014). Automatic identification of agricultural terraces through object-oriented analysis of very high-resolution DSMs and multispectral imagery obtained from an unmanned aerial vehicle. Journal of Environmental Management, 134, 117–126.
Diop, A., Sakho, M., Audebert, A., Mbaye, M., Sine, B., Faye, I., Balota, M., Hoisington, D., & Rhoads, J.l. (2021). High throughput phenotyping methods on peanuts fields. 53rd Annual Meeting of the American Peanut Research and Education Society (APRES), Dallas, USA.
Fischer, R. A., & Edmeades, G. O. (2010). Breeding and cereal yield progress. Crop Science, 50(2), S-85–S-98.
Furbank, R. T., & Tester, M. (2011). Phenomics–technologies to relieve the phenotyping bottleneck. Trends in Plant Science, 16(12), 635–644.
Gano, B, Dembele, J. S. B., Ndour, A., Luquet, D., Beurier, G., Diouf, D., & Audebert, A. (2021). Using UAV borne, multi-spectral imaging for the field phenotyping of shoot biomass, leaf area index and height of West African sorghum varieties under two contrasted water conditions. Agronomy, 11(5), 850.
Gevaert, A. I., Parinussa, R. M., Renzullo, L. J., van Dijk, A. I. J. M., & de Jeu, R. A. M. (2016). Spatio-temporal evaluation of resolution enhancement for passive microwave soil moisture and vegetation optical depth. International Journal of Applied Earth Observation and Geoinformation, 45, 235–244.
Gómez-Candón, D., De Castro, A. I., & López-Granados, F. (2013). Assessing the accuracy of mosaics from unmanned aerial vehicle (UAV) imagery for precision agriculture purposes in wheat. Precision Agriculture, 15(1), 44–56.
González-Dugo, M. P., Escuin, S., Cano, F., Cifuentes, V., Padilla, F. L. M., Tirado, J. L., Oyonarte, N., Ferandez, P., & Mateos, L. (2013). Monitoring evapotranspiration of irrigated crops using crop coefficients derived from time series of satellite images. II. Application on basin scale. Agricultural Water Management, 125, 92–104.
Gonzalez-Dugo, V., Hernandez, P., Solis, I., & Zarco-Tejada, P. (2015). Using high-resolution hyperspectral and thermal airborne imagery to assess physiological condition in the context of wheat phenotyping. Remote Sensing, 7(10), 13586–13605.
González-Recio, O., Rosa, G. J., & Gianola, D. (2014). Machine learning methods and predictive ability metrics for genome-wide prediction of complex traits. Livestock Science, 166, 217–231.
Han-Ya, I., Ishii, K., & Noguchi, N. (2010). Monitoring rice growth environment by low-altitude remote sensing using spectroradiometer. IFAC Proceedings Volumes, 43(26), 184–189.
Hunt, E. R., Cavigelli, M., Daughtry, C. S. T., Mcmurtrey, J. E., & Walthall, C. L. (2005). Evaluation of digital photography from model aircraft for remote sensing of crop biomass and nitrogen status. Precision Agriculture, 6(4), 359–378.
Li, J., Zhang, F., Qian, X., Zhu, Y., & Shen, G. (2015). Quantification of rice canopy nitrogen balance index with digital imagery from unmanned aerial vehicle. Remote Sensing Letters, 6(3), 183–189.
Li, L., Zhang, Q., & Huang, D. (2014). A review of imaging techniques for plant phenotyping. Sensors, 14(11), 20078–20111.
Liebisch, F., Kirchgessner, N., Schneider, D., Walter, A., & Hund, A. (2015). Remote, aerial phenotyping of maize traits with a mobile multi-sensor approach. Plant Methods, 11(1), 9.
Mathews, A., & Jensen, J. (2013). Visualizing and quantifying vineyard canopy LAI using an unmanned aerial vehicle (UAV) collected high density structure from motion point cloud. Remote Sensing, 5(5), 2164–2183.
Neilson, E. H., Edwards, A. M., Blomstedt, C. K., Berger, B., Møller, B. L., & Gleadow, R. M. (2015). Utilization of a high-throughput shoot imaging system to examine the dynamic phenotypic responses of a C4 cereal crop plant to nitrogen and water deficiency over time. Journal of Experimental Botany, 66(7), 1817–1832.
Nigon, T. J., Mulla, D. J., Rosen, C. J., Cohen, Y., Alchanatis, V., Knight, J., & Rud, R. (2015). Hyperspectral aerial imagery for detecting nitrogen stress in two potato cultivars. Computers and Electronics in Agriculture, 112, 36–46.
Øvergaard, S. I., Isaksson, T., Kvaal, K., & Korsaeth, A. (2010). Comparisons of two hand-held, multispectral field radiometers and a hyperspectral airborne imager in terms of predicting spring wheat grain yield and quality by means of powered partial least squares regression. Journal of Near Infrared Spectroscopy, 18(4), 247–261.
Rahaman, M., Chen, D., Gillani, Z., Klukas, C., & Chen, M. (2015). Advanced phenotyping and phenotype data analysis for the study of plant growth and development. Frontiers in Plant Science, 6, 619–619.
Ray, D. K., Mueller, N. D., West, P. C., & Foley, J. A. (2013). Yield trends are insufficient to double global crop production by 2050. PloS One, 8(6), e66428.
Sankaran, S., Khot, L. R., Espinoza, C. Z., Jarolmasjed, S., Sathuvalli, V. R., Vandemark, G. J., Miklas, P. N., Carter, A. H., Pumphrey, M. O., Knowles, N. R., & Pavek, M. J. (2015). Low-altitude, high-resolution aerial imaging systems for row and field crop phenotyping: A review. European Journal of Agronomy, 70, 112–123.
Sugiura, R., Noguchi, N., & Ishii, K. (2005). Remote-sensing technology for vegetation monitoring using an unmanned helicopter. Biosystems Engineering, 90(4), 369–379.
Swain, K. C., Thomson, S. J., & Jayasuriya, H. P. (2010). Adoption of an unmanned helicopter for low-altitude remote sensing to estimate yield and total biomass of a rice crop. Transactions of the ASABE, 53(1), 21–27.
Tattaris, M., Reynolds, M. P., & Chapman, S. C. (2016). A direct comparison of remote sensing approaches for high-throughput phenotyping in plant breeding. Frontiers in Plant Science, 7, 1131.
Thorp, K. R., Gore, M. A., Andrade-Sanchez, P., Carmo-Silva, A. E., Welch, S. M., White, J. W., & French, A. N. (2015). Proximal hyperspectral sensing and data analysis approaches for field-based plant phenomics. Computers and Electronics in Agriculture, 118, 225–236.
Thorp, K., Thompson, A., Harders, S., French, A., & Ward, R. (2018). High-throughput phenotyping of crop water use efficiency via multispectral drone imagery and a daily soil water balance model. Remote Sensing, 10(11), 1682.
Wallace, L., Lucieer, A., Watson, C., & Turner, D. (2012). Development of a UAV-LiDAR system with application to forest inventory. Remote Sensing, 4(6), 1519–1543.
White, J. A., Ryley, M. J., George, D. L., Kong, G. A., & White, S. C. (2012). Yield losses in grain sorghum due to rust infection. Australasian Plant Pathology, 41(1), 85–91.
Yang, W., Duan, L., Chen, G., Xiong, L., & Liu, Q. (2013). Plant phenomics and high-throughput phenotyping: Accelerating rice functional genomics using multidisciplinary technologies. Current Opinion in Plant Biology, 16(2), 180–187.
Zaman-Allah, M., Vergara, O., Araus, J. L., Tarekegne, A., Magorokosho, C., Zarco-Tejada, P. J., Hornero, A., Alba, A. H., Das, B., Craufurd, P., Olsen, M., Prasanna, B. M., & Cairns, J. (2015). Unmanned aerial platform-based multi-spectral imaging for field phenotyping of maize. Plant Methods, 11(1), 35.
Zarco-Tejada, P. J., Diaz-Varela, R., Angileri, V., & Loudjani, P. (2014). Tree height quantification using very high-resolution imagery acquired from an unmanned aerial vehicle (UAV) and automatic 3D photo-reconstruction methods. European Journal of Agronomy, 55, 89–99.
Zhang, C., & Kovacs, J. M. (2012). The application of small unmanned aerial systems for precision agriculture: A review. Precision Agriculture, 13(6), 693–712.