10 High-throughput Root Phenotyping: Opportunities and Challenges for the Adaptation of Arid and Semi-arid Crops to Future Climates
Abstract
Emerging constraints associated with climate change are posing an increasing threat to crop yields. In the arid and semiarid regions of Africa, crop failure, due to extreme events such as drought, is predicted to cause major food security issues. Root traits can improve crop resilience to drought and poor soil conditions by increasing the crop’s ability to acquire water and soil nutrients. However, selection based on root traits in agronomically meaningful contexts poses practical challenges. In fact, phenotyping represents a major bottleneck for root traits breeding, with trade-offs between precision and throughput. In this chapter, we will describe advances in low- and high-throughput root observation and sampling methods that can be easily deployed to measure root traits in field conditions. We will further describe a high-throughput root phenotyping pipeline that has recently been implemented to phenotype root architectural and anatomical traits in pearl millet grown in West African Sahelian conditions. These new methods can facilitate the selection of next generation crops with improved root systems that are more adapted to future climates.
Keywords: root, field, drought, pearl millet, shovelomics, laser ablation tomography
Introduction
Changing climatic conditions are challenging crop production worldwide. Therefore, it is important to develop crop varieties that are more resilient to extreme weather conditions (e.g., temperature, floods, or drought). Such efforts are particularly required for crops grown in low income Sahelian areas, where guaranteeing crop yields is of major importance for food security. Crops with improved root systems that better capture water and nutrients have recently demonstrated utility in adapting to future climates (Lynch, 2018, 2019; Rogers & Benfey, 2015; Uga et al., 2013).
Several root architectural and anatomical traits (see Figure 1) have been described as beneficial for improving drought tolerance. In maize, plants developing fewer crown roots with steeper growth angles developed more roots at depth (more carbon is invested in growth of individual roots) and showed increased drought tolerance (Gao & Lynch, 2016; Lynch, 2018). In crops growing in arid and semiarid environments—such as sorghum, pearl millet or chickpea—deep rooting may improve access to water and grain production, especially when drought occurs at reproductive and grain-filling stages (Lynch, 2013; Thorup-Kristensen et al., 2020; Vadez et al., 2013). Reduced xylem diameter may also improve transpiration efficiency (biomass produced/water used) and grain production under drought in dryland cereals (Vadez, 2014; Vadez et al., 2014; Zhang et al., 2018). Breeding programs specifically targeting reduction in xylem diameter in wheat led to new varieties having more parsimonious use of water that yielded 11% more grains under drought conditions (Richards & Passioura, 1989).
Figure 1
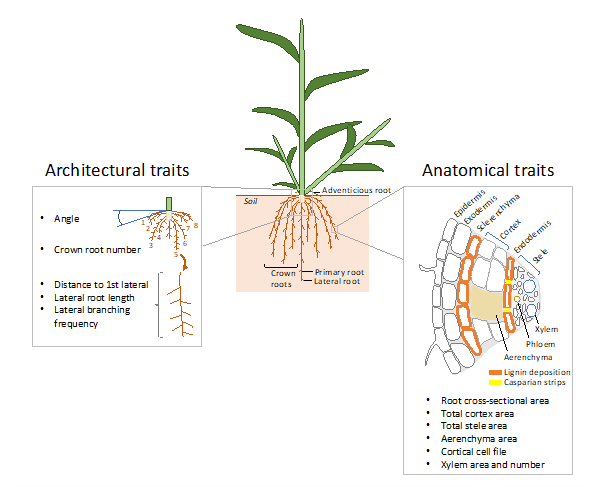
Note. The main architectural and anatomical root traits measured in high-throughput root phenotyping field studies are represented in the left and right boxes, respectively.
However, roots are complex organs constantly developing and adapting to the spatio-temporal heterogeneity in soil properties and resources (Maqbool et al., 2022). Although single traits have shown to improve drought tolerance in specific contexts, it is likely that more significant progress will be accomplished when considering root trait synergisms and integrated phenotypes (Lynch, 2022). Recently phenotyping multiple root traits, along with yield and yield-component related traits in field conditions, revealed that clusters of root phenotypes allowing greater soil exploration, restriction of water uptake, and greater soil penetrability could be considered breeding ideotypes for drought tolerance in maize (Klein et al., 2020).
Identification of markers controlling these root traits through genetic approaches rely on robust high-throughput root phenotyping techniques. For pre-breeding approaches, ideally these techniques should be deployed in agronomical conditions (Lynch, 2022). Still, the high dependence of root development on the environment does not guarantee the successful use of these markers in breeding programs (Lynch et al., 2021). For that matter, identification of easy to measure root phenotypes that could represent a proxy for integrated phenotypes, and development of easy to deploy phenotyping techniques to measure them, may facilitate the integration of root traits in breeding schemes.
A large number of root phenotyping techniques in laboratory, greenhouse, or field conditions have been developed recently (for reviews see Atkinson et al., 2019; Tracy et al., 2020; Wasaya et al., 2018; Watt et al., 2020). Here, we specifically describe recent advances in low- and high-throughput techniques that can be easily deployed in field conditions for root observation, imaging, sampling, and further trait characterization. Some of these techniques can be used to identify the genetic determinants controlling root traits that are beneficial for crop adaptation to drought, as illustrated in a case study describing high-throughput phenotyping of pearl millet root architectural and anatomical traits in field trials in Senegal. These techniques may also be of interest for breeders seeking direct selection of desirable root traits in their breeding program.
Root Phenotyping in Agronomic Conditions
1. Architectural Traits
Trenches – The trench method predicts root length density from root intersections counted on a vertical soil surface (trench). This method requires a calibration step where the relationship between root intersection density (RID) and root length density (RLD) is modeled (Chopart & Siband, 1999; Tennant, 1975). The model is relatively robust within one species grown in one soil type; new calibration steps would be required when phenotyping in other soil composition and organization (Faye et al., 2019b). Once the model is created, the software Racine 2.2 calculates RLD and generates 2D maps along a soil profile (Faye et al., 2019a). These maps provide agronomically meaningful information to estimate water or nutrient acquisition in different soil horizons.
This method is not high-throughput but allows easy and low-cost analysis of root system response to management practices or environmental factors. Models for RID conversion to RLD exist for maize, sorghum, sugarcane, rice, and pearl millet (Chopart & Siband, 1999; Chopart et al., 2008a, 2008b; Dusserre et al., 2009; Faye et al., 2019b). In pearl millet, the model was used to study root drought-responses of a drought-sensitive dual-purpose variety and a less drought-sensitive inbred line in typical Sahelian soils (Faye et al., 2019b). This approach revealed a reallocation of carbon for root growth, combined with a reduction of RLD in topsoil layers under drought, which is hypothesized to be beneficial for water capture (Faye et al., 2019b).
Minirhizotron imaging – Field minirhizotron imaging is a nondestructive method for dynamic visualization of root growth in situ (Vamerali et al., 2012). It involves installing a transparent glass or plastic tube inside the rooting zone of a plant and inserting a camera inside the tube to visualize the roots coming into contact with the tube. Tubes can be of different lenghts and installed within the soil horizontally, vertically, or at an angle (usually between 30° and 60°) without excessive soil disturbance and with good soil-to-tube contact (Vamerali et al., 2012). Angled tubes are useful to obtain information about root vertical distribution; horizontal tubes installed at different depths provide information about root depth distribution (Johnson et al., 2001). Recently, an ingenious minirhizotron imaging facility, where plants were sown directly on top of 5.5 m tubes inserted into the soil with an angle close to the horizontal (15.8° and 23.5°), allowed a larger observation area per unit of soil depth covered (Svane et al., 2019b). Root images are usually performed using commercially available digital cameras (Arnaud et al., 2019; Ohashi et al., 2015), although improved root detection in soil was recently obtained using visible to near-infrared images (Svane et al., 2019a). Images are used to extract root traits, such as root intensity by counting root intersections on a grid superimposed on the image (Chen et al., 2019). Open-source or commercially available sofware such as RootFly (Zeng et al., 2008) or RootSnap (Ohashi et al., 2015) can also be used to extract root length, root diameter, and root area from the images.
Minirhizotron imaging could be considered high-throughput because imaging can take from 90 seconds to 5 minutes depending on the imaging system and image resolution (Arnaud et al., 2019), although to date it has not been used in such fashion in field experiments. In semifield experiments (i.e., recontructed field rather than open field), minirhizotron were used to study root depth in spring barley genotypes (Svane et al., 2019b) and the relationship between root depth and nitrate uptake in winter wheat (Chen et al., 2019; Wacker et al., 2022). The later studies demontrated that deep root traits can predict deep nitrate uptake, which could be exploited to measure deep root growth and function using 15N tracer.
Soil coring – Soil coring involves the insertion of a core sampler to a defined depth at the proximity of a plant, followed by soil core breaks and soil collection at different depths for root washing, scanning, and RLD calculation (Böhm, 1979; Schroth & Kolbe, 1994; Shashidhar et al., 2012). The core sampler is usually made of steel pipes (10 cm to 45 cm in diameter and 60 cm to 2 m in length), with a sharpened end that enters the soil and a hinged opening to aid soil core removal (Henry et al., 2015; Wasson et al., 2014). The core size, number of cores taken, and location of core sampling varies between studies (Bengough et al., 2000). This technique is intrinsically prone to error and the significance of the results much depends on the location and the number of samples (Rossi & Nuutinen, 2004). Indeed, significant variations among soil coring locations within a plot have been observed. It is generally accepted that RLD variance decreases as the sample number increases. Virtual RLD measurements using the CRootBox model estimated that between 10 and 50 core samples per plot would be required to reach a 10% standard error in wheat and maize, respectively (Morandage et al., 2019; Schnepf et al., 2018). More recently, similar approaches comparing virtual soil coring using the OpenSimRoot model and actual field soil coring clarified the location (5 cm from the plant base between rows) and the sampling number (five) to best estimate RLD in maize and common bean (Burridge et al., 2020; Postma et al., 2017). Nonetheless, sampling at three locations around the plant within a plot, within four different plots, showed differences in RLD along the soil profile in rice (Grondin et al., 2018), while a single sample per plot, within four plots, differentiated lines for RLD and maximum depth in wheat (Wasson et al., 2014).
Although soil coring cannot be considered high-throughput because of its labor-intensive characteristics, it was deployed to identify Quantitative Trait Loci (QTL) controlling root mass at different depths in a BC2F3-population, derived from a cross between Moroberekan and Swarna rice cultivars. Some of these QTL collocated with QTL controlling yield component-related traits (Dixit et al., 2015). However, the validity of these QTL remains to be demonstrated. To increase the throughput of soil coring for QTL identification, a new methodology was developed where coring was facilitated using a tractor-mounted hydraulic soil corer (Wasson et al., 2016). Root washing and scanning were replaced by automated counts of root impacts on both sides of soil-core breaks using UV illumination and fluorescence spectroscopy, allowing for RLD calculation directly in the field (Wasson et al., 2016).
Shovelomics – Shovelomics consists of manually excavating the crown root system using a shovel. The soil around the root system is then gently cleaned to manually measure root angle; to count crown roots number; or to take images to automatically extract numerous architectural traits, such as root angle, root width, lateral root length, and branching frequency using image analysis software such as Digital Imaging of Root Traits (DIRT) or Root Estimator for Shovelomics Traits (REST; Bucksch et al., 2014; Colombi et al., 2015). This method was developed on maize and used to successfully quantify root architectural traits in other crops, including common bean, cowpea, and wheat (Burridge et al., 2016; Das et al., 2015; Trachsel et al., 2011; York et al., 2018).
When combined with digital imaging, shovelomics is high-throughput and has been used to identify QTL controlling root length density or width in cowpea (Burridge et al., 2017). In maize, the deployment of this method on a panel of 400 sequenced genotypes, grown in different environments under irrigated and drought-stress conditions, allowed for better understanding of the interactions between the architectural traits associated with drought tolerance and the genetic determinants controlling architectural plasticity (Klein et al., 2020; Schneider et al., 2020b).
2. Anatomical Traits
Hand & microtome sectioning – Root anatomy is traditionally studied using histological approaches consisting in transverse sections of fixed or fresh tissue that can be observed under an optical microscope (usually 50x to 100x magnification) equipped with a digital camera for image capture (Ruzin, 1999). Fresh materials are often conserved in ethanol or methanol (usually 50% v/v or above), and sections are obtained by hand or by using a vibrating microtome. Hand sectioning requires a high level of skill obtaining fine sections without damaging samples, especially in the case of thin roots (Lhotáková et al., 2008). Microtome sectioning requires a prior time-consuming step of paraffin wax or resin embedding, although simpler root embedding protocols in agarose without fixation have been successfully developed (Zelko et al., 2012). Recently, an updated protocol increasing throughput of sectioning using a vibrating microtome on fresh, nonfixed root samples conserved in ethanol and embedded in agarose has been developed (Atkinson & Wells, 2017). The main upgrade in the method consists of using custom designed, 3D-printed molds to embed five to 15 roots in a block that can be sectioned in a single cut. It should be noted, however, that fixation or embedding can cause deformations in the tissue structure due to softening or shrinking, which requires taking multiple images per sample to increase the likelihood of quality images.
Anatomical features can be quantified from the acquired image by using simple software image analyses such as ImageJ (Schneider et al., 2012), or more automated software such as RootScan (Burton et al., 2012), PHIV-RootCell (Lartaud et al., 2015), or RootAnalyzer (Chopin et al., 2015). Although these methods are not considered high-throughput, sectioning was used to study the anatomical features in a panel of sequenced 274 rice genotypes under both irrigated and drought-stress conditions to identify genetic determinants controlling six anatomical traits (root diameter; cortex diameter; stele diameter; metaxylem diameter; and number and stele diameter in proportion of root diameter) and their plasticity (Kadam et al., 2017).
Laser ablation tomography (LAT) – LAT is a novel imaging technology used for rapid and simultaneous sectioning and imaging of roots at spatial scales, (e.g., from 0.1 mm to 1 cm) with micron-level resolution (Hall & Lanba, 2019; Strock et al., 2019). The fresh root segments are often preserved in ethanol (50% v/v or above), dehydrated using graded ethanol series (e.g., 75%, 85%, 95% and 100%), and then dried in a critical point dryer to preserve their cellular structure. Methanol can also be used for preservation before transfer to 100% ethanol prior to critical drying point. LAT also is frequently conducted on fresh samples without the critical drying point step.
The LAT imaging system consists of three components: an ultrafast pulsed UV laser source, a motorized linear stage to which the root sample is mounted, and a DSLR camera or machine vision camera with infinity-corrected long working distance objectives. The beam from the laser source is deflected through a galvanometer along a rapidly scanned line, which is used as a cutting sheet to ablate the root sample by moving it into the beam path using a high-precision motorized linear stage. Autofluorescence from the ablation of the sample by UV wavelengths is simultaneously captured using the camera system. A 3D stack of the cellular structure of the sample can be generated by feeding the sample into the ablation beam at fixed increments using the motorized sample stage.
Anatomical parameters can be qualified from LAT image stacks using semiautomated software such as RootScan or MiPAR (Burton et al., 2012; Schneider et al., 2020a; Strock et al., 2019) or highly automated, next generation machine-learning and deep-learning imaging software (Hall & Lanba, 2019). This method is high-throughput (each root sample takes between 2 minutes and 5 minutes to process). LAT has been recently used to measure root anatomical traits such as root cortical aerenchyma area, cortical cell file number, and cortical cell size in 641 maize genotypes grown in the field under irrigated and water-deficit conditions in different environments (Schneider et al., 2020a). The heritability of these traits was compatible with genetic association analyses and allowed the identification of QTLs and genes possibly involved in the control of root anatomical plasticity in response to drought (Schneider et al., 2020a). LAT has also been used to visualize root colonization by arbuscular mycorrhizal fungi (Strock et al., 2019) or more recently lignification in maize (Schneider et al., 2021b).
Case Study: High-throughput Pearl Millet Root Architectural and Anatomical Phenotyping in West African Soils
To better identify the root architectural and anatomical traits that are beneficial for drought tolerance in pearl millet and the genetic determinants that control these traits, an experiment was set up where 160 genotypes from the Pearl Millet inbred Germplasm Association Panel (PMiGAP) were grown in field conditions in the Centre National de la Recherche Agronomique (CNRA) of the Institut Sénégalais des Recherches Agricoles (ISRA) in Bambey, Senegal (14.42°N, 16.28°W). The PMiGAP panel includes inbred lines from Africa and Asia that are fully sequenced, allowing the identification of 29,542,173 Single-Nucleotide Polymorphisms (SNPs) that can be used for association genetics (Varshney et al., 2017). In our study, plants were grown during the dry season (March to June, which allowed for full irrigation control) under two treatments: irrigated, where plants received 30 mm of water twice a week; and drought stressed, where irrigation was stopped from 21 days to 42 days after sowing (DAS) to allow field dry down. The experiment was set up in an alpha-lattice design with four replications in each treatment, one plot being comprised of three rows of 10 plants with 90 cm between rows and 30 cm between plants within the row. At 42 DAS, three plants per plot with their main tiller tagged were harvested for root phenotyping (shovelomics and anatomics using LAT; see Figure 2) while the remaining plants were kept intact for morphological, yield, and yield components measurements at maturity.
Figure 2
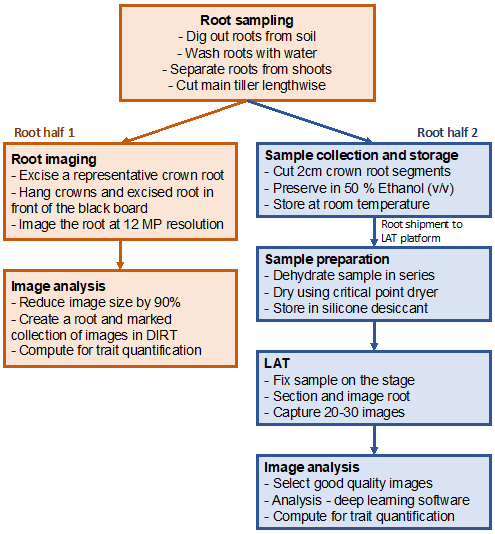
3. Shovelomics
Root sampling and washing – At 42 DAS, the crown root system over a depth of 15 cm to 20 cm was sampled on three plants from the middle row using a shovel (see Figure 3a). The root system was initially washed in buckets filled with water and the shoot part was separated from the root system at around 10 cm above the main stem (see Figure 3b). The number of tillers per plant was counted and the shoots were placed in bags for measurement of aerial dry weight. The root system was further separated in half by cutting the main stem lengthwise. Half of the root system was used for imaging while the other was used for root sampling and anatomical measurements.
Root imaging – One representative crown root was excised from the half root system used for imaging and both were installed in the imaging chamber. The chamber consists of a black board in front of which the roots were hung, protected from direct sunlight by a white cloth. All hanging elements were of dark color so as not to be recognized as root material. Only a scale marker and the tag identifier were white in color. A digital camera was installed on a tripod approximately 1 m away from the black board and images were taken at a resolution of 12 megapixels. The shutter speed of the camera can be adjusted in case of root movement (due to wind, for instance) as well as the focal length in case of large changes in light intensity across the day. Low and homogenous light reflectance from the black board is important for further correct root segmentation by image analyses software.
Figure 3
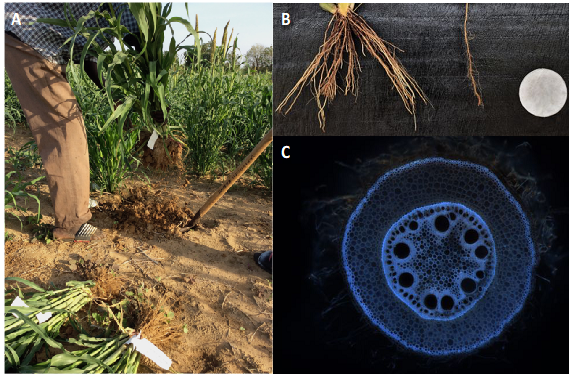
Notes. (A) Root sampling using the shovelomics method (photo by J. Burridge). (B) Image of the shallow root system from a single plant after washing. The root system was cut in half and one crown root was excised (photo by A. Grondin). (C) Image of the anatomy of a root emerging from the fourth whorl using laser ablation tomography (photo by D. Wells).
Architectural traits extraction – The automated DIRT software was used to measure the architectural traits according to Bucksch et al. (2014) and Das et al. (2015). Briefly, before being submitted to DIRT, the size of the images was reduced by 90% and bulk analyses of more than 100 images were performed at once. Although DIRT output provides 78 traits in total (among which 28 traits are specifically for monocotyledons roots), data analysis focused on the following traits:
- crown roots: root angle (top and bottom), root width (top, bottom, and accumulated over 10% to 90% depth), projected root area and averaged root density.
- excised roots: average lateral root length, distance to the first lateral root from the root apex, lateral branching frequency.
The presence of tillers makes more difficult the homogeneous root cutting into half and root hanging in a perfectly vertical position. Therefore, for tillering plants, root angle measurements using DIRT may be prone to errors. Alternatively, manual root angle measurements using the ObjectJ plugin in ImageJ can be performed (methods can be found at https://plantscience.psu.edu/research/labs/roots/methods/computer/rsaj).
Materials and equipment needed for shovelomics:
- Shovels for root sampling
- Buckets for root washing
- Paper bags for shoot harvesting and drying
- Table and cutter for stem dissection
- Black board, nails, and clamps to hang the roots
- White cloth to cover the black board
- Digital camera (Nikon D5600—other models are available) and tripod
- Hard drives for images storing
- Computer with internet access for DIRT analyses
4. Anatomics
Root sampling and storage – On the root half that was conserved for anatomical sampling (see section Shovelomics/Root sampling and washing), roots emerging from whorl four (of the layer of crown roots 1 and 8 in Figure 1) were identified. Two root segments of around 2 cm from the stem base were sampled and placed in a labeled 2 ml tube containing 50% ethanol (v/v) for conservation (roots can be conserved in higher ethanol concentration for several years) and transported to the analysis facility. Samples were then taken through an ethanol dehydration series from 50% to 100% (v/v) in 10% increments. Roots were left to rest at each dehydration stage for a minimum of 60 minutes. Following ethanol dehydration, samples were dried using a critical point dryer. This process removes all liquid from the sample while keeping the cellular structure intact, allowing samples to be stored indefinitely in sealed containers with silica gel desiccant.
Laser ablation tomography – LAT was conducted on a single sample at a time. Each dried sample is fixed in position on the sample stage using a magnetic clamp. Anatomical sections were then captured using the LAT at 30 μm intervals from the middle 1 cm of each root sample. In most cases, between 10 images and 20 images were captured per sample (see Figure 3c). However, when the section of sample being imaged was damaged, either from initial harvesting in the field or during the drying process, further images were captured. Anatomical traits were extracted using a deep-learning approach trained on ground truth sections that were hand annotated using CellSeT software (Pound et al., 2012).
Materials and equipment needed for anatomics:
- Razor blades for root sampling on the fourth whorl
- 2 ml plastic tubes labeled with ethanol resistant stickers for storing root samples
- Ethanol 50 % v/v for conserving root samples
- Boxes for storing the 2 ml tubes
- Absolute ethanol for sample dehydration in series
- Critical point dryer (Leica EM CPD300—other models are available)
- Sealed containers and silicone desiccant for dry sample storage
- Laser ablation tomograph (LatScan 2.0, L4IS)
- Data storage device and computer for image analysis
Prospects/Opportunities
Recent developments in image-based root phenotyping techniques now allow quantification of a number of root traits in field conditions with a throughput that is compatible with genetic approaches (Atkinson et al., 2019; Tracy et al., 2020). In fact, an increasing number of studies using these techniques (especially shovelomics and LAT) in genetic association approaches to identify QTLs controlling root traits have been published recently. Characterization of root diversity in large panels opens opportunities for breeding as it accelerates the identification of heterotic parents that can be used in conventional breeding approaches to validate and introgress these QTLs in popular varieties. In common bean for instance, germplasm identified with increased root length density in shallow soil were crossed with traditional varieties with deeper root system (Burridge et al., 2016; Burridge et al., 2019). This approach allowed the release of new varieties with shallower root systems that are more tolerant to low P stress in Mozambique (Lynch, 2022; Ndoye et al., 2022).
Large-scale phenotyping leads to the identification of haplotypes at the QTL regions of interest and ultimately of the genes underlying these QTLs. Candidate genes can be found through transcriptomics for the identification of differentially expressed genes between contrasted haplotypes and validated through reverse genetics. In maize, such approaches allowed the identification of a CBL-interacting serine/threonine-protein kinase 15 (ZmCIPK15) controlling root angle (Schneider et al., 2021a). The maize cipk mutant showed a 10° steeper root angle in some nodal specific positions, 18% greater shoot biomass, and 29% greater shoot nitrogen accumulation under suboptimal nitrogen availability as compared to the wild type. Haplotypes can also be used for functional analyses aimed at characterizing the physiological significance of the considered root trait. The use of contrasted maize genotypes for root multiseriate cortical sclerenchyma (lignin accumulation in walls of outer cortex cells) showed that roots with larger sclerenchyma had increased penetration ability in compacted soils (Schneider et al., 2021b).
The architectural, anatomical, and functional characterization of specific root traits is useful for improving root structural and functional models that provide a better view of root growth, development, and functional complexity in the soil. For instance, OpenSimRoot simulates how root traits dynamically interact with the soil environment and how this interaction influences resources acquisition in the soil and plant growth (Postma et al., 2017). The integration of root models into crop and climatic models will be important to define the right root system for the right environment, and the inclusion of root traits into the definition of breeding product profiles for development of future varieties best adapted to the conditions they will be subjected to (Lynch, 2018; Windhausen et al., 2012).
Despite these recent intense prebreeding efforts, examples of deployment of root traits in breeding schemes remain scarce. In this context, continuing the efforts to develop root phenotyping in field conditions and making these techniques usable by breeders remain of major importance. Identifying shoot traits that represent proxies for the expression of root traits are of particular interest too. However, providing breeders with genetic markers for root traits and phenotyping tools may not be enough to ensure the benefits of root traits because of their high degree of plasticity (Dwivedi et al., 2020). When a root trait is identified as beneficial in one environment, it is important to better apprehend how the expression of this trait, and its benefits, may change in other environments (Schneider & Lynch, 2020). Controlling the genotype by environment interaction affecting root traits is arguably the next biggest challenge in root research.
References
Arnaud, M., Baird, A. J., Morris, P. J., Harris, A., & Huck, J. J. (2019). EnRoot: A narrow-diameter, inexpensive and partially 3D-printable minirhizotron for imaging fine root production. Plant Methods. 15, 1–9.
Atkinson, J. A., Pound, M. P., Bennett, M. J., & Wells, D. M. (2019). Uncovering the hidden half of plants using new advances in root phenotyping. Current Opinion in Biotechnology, 55, 1–8.
Atkinson, J. A., & Wells, D. M. (2017). An updated protocol for high throughput plant tissue sectioning. Frontiers in Plant Science, 8, 1–8.
Bengough, A. G., Castrignano, A., Pagès, L., & van Noordwijk, M. (2000). Sampling strategies, scaling, and statistics. In A. L. Smit, A. G. Bengough, & C. Engels (Eds.), Root Methods (pp 147–174). Springer-Verlag.
Böhm, W. (1979). Methods of studying root systems Vol. 33: Ecological Studies. Springer. https://doi.org/10.1007/978-3-642-67282-8
Bucksch, A., Burridge, J., York, L. M., Das, A., Nord, E., Weitz, J. S., & Lynch, J. P. (2014). Image-based high-throughput field phenotyping of crop roots. Plant Physiology, 166(2), 470–486.
Burridge, J., Jochua, C. N., Bucksch, A., & Lynch, J. P. (2016). Legume shovelomics: High-throughput phenotyping of common bean (Phaseolus vulgaris L.) and cowpea (Vigna unguiculata subsp, unguiculata) root architecture in the field. Field Crops Research, 192, 21–32.
Burridge, J. D., Black, C. K., Nord, E. A., Postma, J. A., Sidhu, J. S., York, L. M., & Lynch, J. P. (2020). An analysis of soil coring strategies to estimate root depth in maize (Zea mays) and common bean (Phaseolus vulgaris). Plant Phenomics, 2020, 1–20.
Burridge, J. D., Findeis, J. L., Jochua, C. N., Miguel, M. A., Mubichi-Kut, F. M., Quinhentos, M. L., Xerinda, S. A., & Lynch, J. P. (2019). A case study on the efficacy of root phenotypic selection for edaphic stress tolerance in low-input agriculture: common bean breeding in Mozambique. Field Crops Research, 244, Article 107612.
Burridge, J. D., Schneider, H. M., Huynh, B.-L., Roberts, P. A., Bucksch, A., & Lynch, J. P. (2017). Genome-wide association mapping and agronomic impact of cowpea root architecture. Theoretical and Applied Genetetics, 130, 419–431.
Burton, A. L., Williams, M., Lynch J. P., & Brown, K. M. (2012). RootScan: Software for high-throughput analysis of root anatomical traits. Plant and Soil, 357, 189–203.
Chen, S., Svane, S. F., & Thorup-Kristensen, K. (2019). Testing deep placement of an 15N tracer as a method for in situ deep root phenotyping of wheat, barley and ryegrass. Plant Methods, 15, 1–12.
Chopart, J.-L., Rodrigues, S. R., Carvalhoe Azevedo, M., & de Conti Medina, C. (2008a). Estimating sugarcane root length density through root mapping and orientation modelling. Plant and Soil, 313, 101–112.
Chopart, J. L., & Siband, P. (1999). Development and validation of a model to describe root length density of maize from root counts on soil profiles. Plant and Soil, 214, 61–74.
Chopart, J.-L., Sine, B., Dao, A., & Muller, B. (2008b). Root orientation of four sorghum cultivars: application to estimate root length density from root counts in soil profiles. Plant Root, 2, 67–75.
Chopin, J., Laga, H., Huang, C. Y., Heuer, S., Miklavcic, S. J. (2015). RootAnalyzer: A cross-section image analysis tool for automated characterization of root cells and tissues. PLOS ONE, 10(11), 1–22.
Colombi, T., Kirchgessner, N., Le Marié, C. A., York, L. M., Lynch, J. P., & Hund, A. (2015). Next generation shovelomics: set up a tent and REST. Plant and Soil, 388, 1–20.
Das, A., Schneider, H., Burridge, J., Ascanio, A. K. M., Wojciechowski, T., Topp, C. N., Lynch, J. P., Weitz, J. S., & Bucksch, A. (2015). Digital imaging of root traits (DIRT): a high-throughput computing and collaboration platform for field-based root phenomics. Plant Methods, 11, 1–12.
Dixit, S., Grondin, A., Lee, C.-R., Henry, A., Olds, T.-M., & Kumar, A. (2015). Understanding rice adaptation to varying agro-ecosystems: trait interactions and quantitative trait loci. BMC Genetics, 16, 86.
Dusserre, J., Audebert, A., Radanielson, A., & Chopart, J.-L. (2009). Towards a simple generic model for upland rice root length density estimation from root intersections on soil profile. Plant and Soil, 325, 277.
Dwivedi, S. L., Stoddard, F. L., & Ortiz, R. (2020). Genomic-based root plasticity to enhance abiotic stress adaptation and edible yield in grain crops. Plant Science, 295, Article 110365.
Faye, A., Chopart, J.-L., Sine, B., Grondin, A., & Laplaze, L. (2019a). Racine2.2 (Version 2) [Application]. DataSuds. https://doi.org/10.23708/CKRDBW
Faye, A., Sine, B., Chopart, J.-L., Grondin, A., Lucas, M., Diedhiou, A. G., Gantet, P., Cournac, L., Min, D., Audebert, A., Kane, A., & Laplaze, L. (2019b). Development of a model estimating root length density from root impacts on a soil profile in pearl millet (Pennisetum glaucum (L.) R. Br). Application to measure root system response to water stress in field conditions. PLOS ONE, 14(7), Article e0214182.
Gao, Y., & Lynch, J. P. (2016). Reduced crown root number improves water acquisition under water deficit stress in maize (Zea mays L.). Journal of Experimental Botany, 67(15), 4545–4557.
Grondin, A., Dixit, S., Torres, R., Venkateshwarlu, C., Rogers, E., Mitchell-Olds, T., Benfey, P. N., Kumar, A., & Henry, A. (2018). Physiological mechanisms contributing to the QTL qDTY3.2 effects on improved performance of rice Moroberekan x Swarna BC2F3:4 lines under drought. Rice, 11, 1–17.
Hall, B., & Lanba, A. (2019). Three-dimensional analysis of biological systems via a novel laser ablation technique. Journal of Laser Applications, 31, Article 022602.
Henry, A., Mallikarjuna Swamy, B. P., Dixit, S., Torres, R. D., Batoto, T. C., Manalili, M., Anantha, M. S., Mandal, N. P., & Kumar, A. (2015). Physiological mechanisms contributing to the QTL-combination effects on improved performance of IR64 rice NILs under drought. Journal of Experimental Botany, 67, 1787–1799.
Johnson, M. G., Tingey, D. T., Phillips, D. L., & Storm, M. J. (2001). Advancing fine root research with minirhizotrons. Environmental and Experimental Botany, 45(3), 263–289.
Kadam, N. N., Tamilselvan, A., Lawas, L. M. F., Quiñones, C., Bahuguna, R. N., Thomson, M. J., Dingkuhn, M., Raveendran, M., Struik, P. C., Yin, X., & Jagadish, K. S. V. (2017). Genetic control of plasticity in root morphology and anatomy of rice in response to water deficit. Plant Physiology, 174(4), 2302–2315.
Klein, S. P., Schneider, H. M., Perkins, A. C., Brown, K. M., & Lynch, J. P. (2020). Multiple integrated root phenotypes are associated with improved drought tolerance. Plant Physiology, 183(3), 1011–1025.
Lartaud, M., Perin, C., Courtois, B., Thomas, E., Henry, S., Bettembourg, M., Divol, F., Lanau, N., Artus, F., Bureau, C., Verdeil, J.-L., Sarah, G., Guiderdone, E., & Dievart, A. (2015). PHIV-RootCell: A supervised image analysis tool for rice root anatomical parameter quantification. Frontiers in Plant Science, 5, 1–7.
Lhotáková, Z., Albrechtová, J., Janáček, J., & Kubínová, L. (2008). Advantages and pitfalls of using free-hand sections of frozen needles for three-dimensional analysis of mesophyll by stereology and confocal microscopy. Journal of Microscopy, 232(1), 56–63.
Lynch, J. P. (2013). Steep, cheap and deep: an ideotype to optimize water and N acquisition by maize root systems. Annals of Botany, 112(2), 347–357.
Lynch, J. P. (2018). Rightsizing root phenotypes for drought resistance. Journal of Experimental Botany, 69(13), 3279–3292.
Lynch, J. P. (2019). Root phenotypes for improved nutrient capture: an underexploited opportunity for global agriculture. New Phytologist, 223(2), 548–564.
Lynch, J. P. (2022). Harnessing root architecture to address global challenges. The Plant Journal, 109(2), 415–431.
Lynch, J. P., Mooney, S. J., Strock, C. F., & Schneider, H. M. (2021). Future roots for future soils. Plant, Cell & Environment, 45(3), 620-636.
Maqbool, S., Hassan, M. A., Xia, X., York, L. M., Rasheed, A., & He, Z. (2022). Root system architecture in cereals: progress, challenges and perspective. The Plant Journal, 110(1), 23–42.
Morandage, S., Schnepf, A., Leitner, D., Javaux, M., Vereecken, H., & Vanderborght, J. (2019). Parameter sensitivity analysis of a root system architecture model based on virtual field sampling. Plant and Soil, 438, 101–126.
Ndoye, M. S., Burridge, J., Bhosale, R., Grondin, A., & Laplaze, L. (2022). Root traits for low input agroecosystems in Africa: Lessons from three case studies. Plant, Cell & Environment, 45(3), 637–649.
Ohashi, A. Y. P., de Matos Pires, R. C., Ribeiro, R. V., & de Oliveira Silva, A. L. B. (2015). Root growth and distribution in sugarcane cultivars fertigated by a subsurface drip system. Bragantia, 74(2), 131–138.
Postma, J. A., Kuppe, C., Owen, M. R., Mellor, N., Griffiths, M., Bennett, M. J., Lynch, J. P., & Watt, M. (2017). OpenSimRoot: widening the scope and application of root architectural models. New Phytologist, 215(3), 1274–1286.
Pound, M. P., French, A. P., Wells, D. M., Bennett, M. J., & Pridmore, T. P. (2012). CellSeT: novel software to extract and analyze structured networks of plant cells from confocal images. The Plant Cell, 24(4), 1353–1361.
Richards, R. A., & Passioura, J. B. (1989). A breeding program to reduce the diameter of the major xylem vessel in the seminal roots of wheat and its effect on grain yield in rain-fed environments. Austrilian Journal of Agricultural Research, 40(5), 943–950.
Rogers, E. D., & Benfey, P. N. (2015). Regulation of plant root system architecture: implications for crop advancement. Current Opinion in Biotechnology, 32, 93–98.
Rossi, J.-P., & Nuutinen, V. (2004). The effect of sampling unit size on the perception of the spatial pattern of earthworm (Lumbricus terrestris L.) middens. Applied Soil Ecology, 27, 189–196.
Ruzin, S. E. (1999). Plant microtechnique and microscopy. In R. Schmid (Ed.), Taxon (Vol. 48). Wiley.
Schneider, C. A., Rasband, W. S., & Eliceiri, K. W. (2012). NIH Image to ImageJ: 25 years of image analysis. Nature Methods, 9, 671–675.
Schneider, H. M., Klein, S. P., Hanlon, M. T., Kaeppler, S., Brown, K. M., & Lynch, J. P. (2020a). Genetic control of root anatomical plasticity in maize. The Plant Genome, 13(1), 1–14.
Schneider, H. M., Klein, S. P., Hanlon, M. T., Nord, E. A., Kaeppler, S., Brown, K. M., Warry, A., Bhosale, R., Lynch, J. P. (2020b). Genetic control of root architectural plasticity in maize. Journal of Experimental Botany, 71(10), 3185–3197.
Schneider, H. M., Lor, V. S. N., Hanlon, M. T., Perkins, A., Kaeppler, S. M., Borkar, A. N., Bhosale, R., Zhang, X., Rodriguez, J., Bucksch, A., Bennett, M. J., Brown, K. M., & Lynch, J. P. (2021a). Root angle in maize influences nitrogen capture and is regulated by calcineurin B-like protein (CBL)-interacting serine/threonine-protein kinase 15 (ZmCIPK15). Plant, Cell & Environment, 45(3) 837-853.
Schneider, H. M., & Lynch, J. P. (2020). Should root plasticity be a crop breeding target? Frontiers in Plant Science, 11, 1–16.
Schneider, H. M., Strock, C. F., Hanlon, M. T., Vanhees, D. J., Perkins, A. C., Ajmera, I. B., Sidhu, J. S., Mooney, S. J., Brown, K. M., & Lynch, J. P. (2021b). Multiseriate cortical sclerenchyma enhance root penetration in compacted soils. Proceedings of the National Academy of Sciences, 118(6), Article e2012087118.
Schnepf, A., Leitner, D., Landl, M., Lobet, G., Mai, T. H., Morandage, S., Sheng, C., Zörner, M., Vanderborght, J., & Vereecken, H. (2018). CRootBox: A structural-functional modelling framework for root systems. Annals of Botany, 121(5), 1033–1053.
Schroth, G., & Kolbe, D. (1994). A method of processing soil core samples for root studies by subsampling. Biology and Fertility of Soils, 18, 60–62.
Shashidhar, H. E., Henry, A., & Hardy, B. (Eds.) (2012). Methodologies for root drought studies in rice. International Rice Research Institute.
Strock, C. F., Schneider, H. M., Galindo-Castañeda, T., Hall, B. T., Van Gansbeke, B., Mather, D. E., Roth, M. G., Chilvers, M. I., Guo, X., Brown, K., & Lynch, J. P. (2019). Laser ablation tomography for visualization of root colonization by edaphic organisms. Journal of Experimental Botany, 70(19), 5327–5342.
Svane, S. F., Dam, E. B., Carstensen, J. M., & Thorup-Kristensen, K. (2019a). A multispectral camera system for automated minirhizotron image analysis. Plant and Soil, 441, 657–672.
Svane, S. F., Jensen, C. S., & Thorup-Kristensen, K. (2019b). Construction of a large-scale semi-field facility to study genotypic differences in deep root growth and resources acquisition. Plant Methods, 15, 1–16.
Tennant, D. (1975). A test of a modified line intersect method of estimating root length. Journal of Ecology, 63(3), 995–1001.
Thorup-Kristensen, K., Halberg ,N., Nicolaisen, M., Olesen, J. E., Crews, T. E., Hinsinger, P., Kirkegaard, J., Pierret, A., & Dresbøll, D. B. (2020). Digging deeper for agricultural resources, the value of deep rooting. Trends in Plant Science, 25(4), 406–417.
Trachsel, S., Kaeppler, S. M., Brown, K. M., & Lynch, J. P. (2011). Shovelomics: High throughput phenotyping of maize (Zea mays L.) root architecture in the field. Plant and Soil, 341, 75–87.
Tracy, S. R., Nagel, K. A., Postma, J. A., Fassbender, H., Wasson, A., & Watt, M. (2020). Crop improvement from phenotyping roots: Highlights reveal expanding opportunities. Trends in Plant Science, 25(1), 105–118.
Uga, Y., Sugimoto, K., Ogawa, S., Rane, J., Ishitani, M., Hara, N., Kitomi, Y., Inukai, Y., Ono, K., Kanno, N., Inoue, H., Takehisa, H., Motoyama, R., Nagamura, Y., Wu, J., Matsumoto, T., Takai, T., Okuno, K., & Yano, Y. (2013). Control of root system architecture by DEEPER ROOTING 1 increases rice yield under drought conditions. Nature Genetics, 45, 1097–1102.
Vadez, V. (2014). Root hydraulics: The forgotten side of roots in drought adaptation. Field Crops Research, 165, 15–24.
Vadez, V., Kholova, J., Medina, S., Kakkera, A., & Anderberg, H. (2014). Transpiration efficiency: New insights into an old story. Journal of Experimental Botany, 65(21), 6141–6153.
Vadez, V., Kholová, J., Yadav, R. S., & Hash, C. T. (2013). Small temporal differences in water uptake among varieties of pearl millet (Pennisetum glaucum (L.) R. Br.) are critical for grain yield under terminal drought. Plant and Soil, 371, 447–462.
Vamerali, T., Bandiera, M., & Mosca, G. (2012). Minirhizotrons in modern root studies. In S. Mancuso (Ed.), Measuring Roots as Update Approach (pp. 341-361). Springer.
Varshney, R. K., Shi, C., Thudi, M., Mariac, C., Wallace, J., Qi, P., Zhang, H., Zhao, Y., Wang, X., Rathore, A., Srivastava, R., Chitikineni, A., Fan, G., Baja, P., Punnuri, S., Gupta, S. K., Want, H., Jiang, Y., Couderc, M., … Xu., X.(2017). Pearl millet genome sequence provides a resource to improve agronomic traits in arid environments. Nature Biotechnology, 35, 969–976.
Wacker, T., Popovic, O., Olsen, N. A. F., Markussen, B., Smith, A. G., Svane, S. F., & Thorup-Kristensen, K. (2022). Semifield root phenotyping: Root traits for deep nitrate uptake. Plant, Cell & Environment, 45(3), 823-836. https://doi.org/10.1111/pce.14227
Wasaya, A., Zhang, X., Fang, Q., & Yan, Z. (2018). Root phenotyping for drought tolerance: A review. Agronomy, 8(11), 1–19.
Wasson, A., Bischof, L., Zwart, A., & Watt, M. (2016). A portable fluorescence spectroscopy imaging system for automated root phenotyping in soil cores in the field. Journal of Experimental Botany, 67(4), 1033–1043.
Wasson, A. P., Rebetzke, G. J., Kirkegaard, J. A., Christopher, J., Richards, R. A., & Watt, M. (2014). Soil coring at multiple field environments can directly quantify variation in deep root traits to select wheat genotypes for breeding. Journal of Experimental Botany, 65(21), 6231–6249.
Watt, M., Fiorani, F., Usadel, B., Rascher, U., Muller, O., & Schurr, U. (2020). Phenotyping: New windows into the plant for breeders. Annual Review of Plant Biology, 71, 689–712.
Windhausen, V.S., Wagener, S., Magorokosho, C., Makumbi, D., Vivek, B., Piepho, H.-P., Melchinger, A. E., & Atlin, G. N. (2012). Strategies to subdivide a target population of environments: Results from the CIMMYT-led maize hybrid testing programs in Africa. Crop Science, 52(5), 2143–2152.
York, L. M., Slack, S., Bennett, M. J., & Foulkes, M. J. (2018). Wheat shovelomics I: A field phenotyping approach for characterising the structure and function of root systems in tillering species. bioRxiv. http://doi.org/10.1101/280875
Zelko, I., Lux, A., Sterckeman, T., Martinka, M., Kollárová, K., & Lisková, D. (2012). An easy method for cutting and fluorescent staining of thin roots. Annals of Botany, 110, 475–478.
Zeng ,G., Birchfield, S. T., & Wells, C. E. (2008). Automatic discrimination of fine roots in minirhizotron images. New Phytologist, 177(2), 549–557.
Zhang, J., Zhang, H., Srivastava, A. K., Pan, Y., Bai, J., Fang, J., Shi, H., & Zhu, J.-K. (2018). Knockdown of rice microRNA166 confers drought resistance by causing leaf rolling and altering stem xylem development. Plant Physiology, 176(3), 2082–2094.