12 Designing Dual-purpose Sorghum Ideotypes for High Grain and Biomass Yields Suitable for Various Target Environments in Senegal
Abstract
Sorghum is a water-efficient crop and an important cereal in semiarid and arid environments where water availability limits production. Providing farmers with sorghum genotypes with specific agro-morphological characteristics of high double production (grains and biomass) would help to maintain or obtain high yields in these environments. The SAMARA model was used to define dual-purpose sorghum ideotypes for different target environments. Model calibration and evaluation were carried out with experimental data collected in Nioro du Rip in 2015 and Sinthiou Malem from 2013 to 2016 on 10 contrasting sorghum genotypes. Daily weather conditions from 1950 to 2016 were used for the simulations. Also considered were the different environmental (soil and climate) conditions of Bambey, Nioro du Rip, and Sinthiou Malem (Senegal). Simulations for the identification of optimal genotypic parameters in these target environments highlighted three ideotypes showing performances for a dual-purpose (grain and fodder) production superior to the reference genotype Nieleni. This was thanks to their strong capacity to colonize the soil (long roots) and to maintain green leaves (stay-green) throughout the cycle. Hence, long roots and stay-green trait should be strongly considered while selecting or developing dual-purpose genotypes of sorghum for the target environments, especially in less-watered areas like Bambey.
Keywords: Sorghum, dual-purpose, SAMARA, ideotype, target environment, Senegal
Introduction
Food security has become a major issue given the need to increase the global food supply by about 70% in 2050 (FAO, 2009). However, given the increasing limits to the expansion of cultivated areas in developing countries (land saturation linked to demographic changes), a significant increase in crop productivity seems necessary to achieve this objective. Sorghum is the fifth most important cereal crop in the world and is the staple food of more than 500 million people in more than 30 countries (ICRISAT, 2009). It is a water-efficient crop, making it an important cereal in semiarid and arid environments where water is the main limiting factor in production. Despite its high-yield potential in terms of grains and biomass, sorghum production is very sensitive to environmental conditions. In Senegal, the average yield of sorghum, the third most important rainfed cereal after millet and maize, remains low (1020 Kg.ha-1; ANSD, 2018) with an estimated total area of more than 221,329 ha for a national production of 225,865 tons. Indeed, its cultivation faces several constraints including low soil fertility (Vanlauwe et al., 2010) and the lack of or low use of agricultural inputs (i.e., fertilizers, seeds of improved varieties; Gerstenmier & Choho, 2015). In addition, rainfall has inter- and intra-annual variabilities (Hansen, 2002).
To boost sorghum and other cereal crop production, farmers must be provided with genotypes that are more efficient and better adapted to their growing conditions. Thus, new sorghum genotypes with specific agro-morphological characteristics that result in a high double production (grains and biomass) in the target environments should be prioritized given the emergency to increase sorghum yields. Several traits have been identified because of their potential to develop sorghum ideotypes with high grain and biomass yields: internode length, stem diameter, leaf number and size, and number of grains per panicle (Ndiaye, 2019).
Donald (1968) was one of the first to propose an “ideotype selection” approach to underpin varietal improvement programs, which until then had been based on two selection philosophies: “elimination of defects” and “selection for yield” (Chantereau & Nicou, 1991). He defined varietal ideotype as an idealized plant, or plant model (not yet in the sense of a mathematical model), that should yield a greater quantity or quality when grown as a cultivar. On the other hand, selection for yield is limited by the fact that the desirable combination of traits is usually achieved by chance.
Reynolds et al. (2011) emphasized the importance of mathematical modeling as a powerful tool for elucidating optimal combinations including tradeoffs between traits for the creation of varietal ideotypes. Cultivation models based on ecophysiological processes are commonly used in basic and applied research in plant science and natural resource management (Hammer et al., 2002; Passioura, 1996; Rötter et al., 2011; Sinclair & Seligman, 1996; White et al., 2011) because they provide the best-known framework for understanding complex plant processes and their interactions with climate and the environment. These models play an increasing role in guiding basic research by providing quantitative predictions and highlighting knowledge gaps (Hammer et al., 2006, 2010; Semenov & Halford, 2009; Semenov & Shewry, 2011; Tardieu, 2003). Modeling can thus provide a rational approach to design and test in silico new sorghum ideotypes optimized for given environments (Hammer et al., 2006; Quilot-Turion et al., 2012; Semenov & Halford, 2009; Semenov & Shewry, 2011; Tardieu & Tuberosa, 2010).
Therefore, this study aims to illustrate the capacity of modeling in the design of sorghum ideotypes with high yields of both grain and biomass for target environments in Senegal using the SAMARA crop model that simulates crop growth and development at a daily time step.
Materials and Methods
1. Model Description
The SAMARA model (Simulator of crop trait Assembly, MAnagement Response and Adaptation) was initially implemented on the EcoTrop platform (programmed in Delphi language, Kouressy et al., 2008), a model development and simulation platform. This platform integrates a series of tools and interfaces: a library of simulation modules, a simulation engine, a user interface with graphical and numerical outputs, simulation scenarios that can be run in bulk, and a database for observed and simulated data among them. A description and commented source code of the model, the definition of all parameters (e.g., crop, plot, soil, and cropping practices), and all input and output variables are available at https://umr-agap.cirad.fr/nos-recherches/equipes-scientifiques/modele-samara. Only the principles and processes relevant to this study are described here. The version used for this study has been recoded under R version 3.2 (R Development Core Team, 2015) to facilitate the link with parameter optimization software and to make it more flexible within the developer community.
SAMARA is a deterministic crop model. Its objective is to simulate the growth of monocotyledons such as tropical cereals, rice, and sorghum. SAMARA simulates—according to radiation, temperature, water, and CO2—the growth and yield of cereals at the canopy scale at daily time steps. Input data for the model includes:
- Standard daily meteorological data (i.e., solar radiation or possibly insolation, minimum and maximum temperatures, mean wind speed, minimum and maximum relative humidity, potential evapotranspiration [Food and Agriculture Organization, FAO], and precipitation);
- Hydrodynamic properties of the soil (i.e., volumetric water content at wilting point, field capacity, and saturation); and
- Technical itineraries (especially sowing date and density).
Simple concepts described by Kouressy et al. (2008) are used to simulate biomass gain using the Big-Leaf approach, Lambert-Beer’s law for the interception of photosynthetically active radiation, and radiation use efficiency for carbon assimilation.
2. Model Calibration and Evaluation
2.1 Experimental Data
The modeling work (calibration and evaluation) was based on data from 11 trials conducted during the rainy seasons of 20132016 at three research stations of the Institut Sénégalais de Recherches Agricoles (ISRA), namely Sinthiou Malem (in 2013, 2014, 2015, and 2016), Bambey (in 2013), and Nioro du Rip (in 2015; see Table 1). The environmental conditions at the different sites are given in Table 2. The main characteristics of these trials (soil type, agronomic management, and standard daily climate data—minimum, maximum, and average temperature; minimum, maximum, and average relative humidity; global radiation; average wind speeds at 2 m from the ground; and rainfall height) that served as inputs for the model were described in detail by Ndiaye et al. (2019).
Trial | Zone | Code | Soil Type* | Sowing Date | Previous Crop | Calibration | Evaluation |
Sowing 1/2013 | BBY | B13D1 | Sandy | 17/07/2013 | Fallow | ||
Sowing 2/2013 | BBY | B13D2 | Sandy | 31/07/2013 | Fallow | ||
Sowing 1/2013 | SIN | S13D1 | Sandy loamy | 25/07/2013 | Fallow | 1 | |
Sowing 2/2013 | SIN | S13D2 | Sandy loamy | 6/8/2013 | Fallow | 1 | |
Sowing 1/2014 | SIN | S14D1 | Sandy | 17/07/2014 | Peanut | 1 | |
Sowing 2/2014 | SIN | S14D2 | Sandy | 6/8/2014 | Peanut | 1 | |
Sowing 1/2015 | SIN | S15D1 | Sandy | 9/7/2015 | Peanut | ||
Sowing 2/2015 | SIN | S15D2 | Sandy | 8/8/2015 | Peanut | 1 | |
Sowing 1/2015 | SIN | S16D1 | Sandy loamy | 25/07/2016 | Fallow | 1 | |
Sowing 1/2015 | NIO | N15D1 | Sandy | 16/07/2015 | Cowpea | ||
Sowing 2/2015 | NIO | N15D2 | Sandy loamy | 13/08/2015 | Fallow | 1 |
Note. BBY = Bambey; SIN = Sinthiou Malem; NIO = Nioro du Rip. *Classification according to the USDA method based on average data over the 0–30 cm horizon.
Code | Coordinates | Alt (m) | *SAN (%) | CS (%) | N (%) | OM (%) | Rain (mm) | R0-30 (mm) |
B13D1 | 14°42’N | 20 | 94.2 | 6.6 | 0.15 | 3.1 | 644 | 180 |
B13D2 | 16°29’W | 94.2 | 6.6 | 0.15 | 3.1 | 566 | 253 | |
S13D1 | 13°49′ N | 23 | 89.4 | 11.6 | 0.21 | 4.3 | 575 | 146 |
S13D2 | 13°55′ W | 89.4 | 11.6 | 0.21 | 4.3 | 536 | 183 | |
S14D1 | 91.2 | 10.2 | 0.17 | 5.7 | 488 | 158 | ||
S14D2 | 90.9 | 9.7 | 0.17 | 4.5 | 377 | 190 | ||
S15D1 | 93.7 | 6.3 | 0.32 | 3.5 | 505 | 52 | ||
S15D2 | 93.2 | 6.8 | 0.33 | 3.8 | 455 | 259 | ||
S16D1 | 84.1 | 15.9 | 0.55 | 10.6 | 447 | 230 | ||
N15D1 | 13°45′ N | 45 | 92.4 | 7.6 | 0.31 | 3.5 | 943 | 196 |
N15D2 | 15°45′ W | 87.0 | 13.0 | 0.43 | 6.1 | 747 | 329 |
Code | Coordinates | Alt (m) | R30-60 (mm) | R60-90 (mm) | R90-120 (mm) | Tmin (°C) | Tmax (°C) | **Healthy |
B13D1 | 14°42’N | 20 | 352 | 110 | 3 | 23 | 33.9 | 2 |
B13D2 | 16°29’W | 256 | 56 | 1 | 22.8 | 33.9 | 3 | |
S13D1 | 13°49′ N | 23 | 365 | 59 | 6 | 21.4 | 35.3 | 5 |
S13D2 | 13°55′ W | 306 | 46 | 1 | 21.2 | 35.4 | 5 | |
S14D1 | 213 | 88 | 31 | 22.2 | 35.7 | 4 | ||
S14D2 | 156 | 31 | 1 | 22.1 | 35.6 | 5 | ||
S15D1 | 259 | 153 | 43 | 21.8 | 34.7 | 2 | ||
S15D2 | 155 | 44 | 2 | 21.2 | 34.9 | 4 | ||
S16D1 | 155 | 24 | 38 | 22.5 | 35.6 | 5 | ||
N15D1 | 13°45′ N | 45 | 361 | 261 | 126 | 20.6 | 33.8 | 3 |
N15D2 | 15°45′ W | 273 | 145 | 0 | 19.7 | 33.8 | 4 |
Notes: BBY = Bambey, SIN = Sinthiou Malem, NIO = Nioro du Rip, Alt = Altitude, SAN = Sand, CS = Clay + Silt, * = classification according to the USDA method based on average data over the 0–30 cm horizon, R030 = total rainfall between 0 and 30 days after sowing, R3060 total rainfall between 30 and 60 days after sowing, R6090 = total rainfall between 60 and 90 days after sowing, R90120 = total rainfall between 90 and 120 days after sowing, ** = score given to a given environment according to disease level: The favorable situation takes the score 5 (absence of disease) and the unfavorable situation the score 1 (strong presence of disease), Rain = total rainfall during the trial.
The plant material consisted of 10 genotypes from various regions of West and Central Africa that are known to perform well in their respective zones of distribution: Fadda, Nieleni, Pablo, Soumba, F2-20, 621B, SK5912, IS15401, Grinkan, and CSM63E. These genotypes have been selected to constitute a contrasting sample in terms of cycle duration, plant architecture (height, stem diameter in particular), structural characteristics (lignin, cellulose), and grain and biomass production. The characteristics of these 10 genotypes were described by Ndiaye et al. (2019). In these trials, observations on flowering and maturity dates, leaf area indices (LAI), biomass and plant height dynamics, yields (grain and biomass), and yield components were used for model calibration and evaluation. Total aboveground biomass and its distribution among stems, leaves and panicles, LAI, and leaf area numbers were monitored weekly in 2013 and 2014 and every 12 days in 2015 and 2016 for up to 10 days after flowering.
2.2 Calibration and Evaluation
The calibration of a model should in principle be carried out using data from trials conducted in well-controlled agronomic situations so that factors not considered by the model (e.g., diseases and weeds) do not sway data. Then the calibration of the model must be evaluated using data that are different and independent from those of the calibration and that come from trials also conducted under good agronomic conditions, if possible. Thus, the data from the three trials conducted in 2014 and 2016 at Sinthiou Malem (S14D1, S14D2, and S16D1) were used to calibrate the phenology, the dynamics of plant biomass, the height, the number of leaves appeared, and the final yields. The LAI was only calibrated on trial S16D1 because of the lack of LAI data in 2014 and because S16D1 was the best trial of all of the experiments with a very good soil fertility, an absence of disease, and low overall rainfall. Therefore, the LAI data from this trial are assumed to be the best. In addition to their good agronomic conditions, S14D1 and S14D2 had a 20-day delay between sowing dates, which is important for the calibration of the phenology. The evaluation of the model was done with trials conducted in 2013 and 2015 at Sinthiou Malem (S13D1, S13D2, and S15D2) and in 2015 at Nioro du Rip (N15D2; see Table 1). These trials had relatively good agronomic conditions (i.e., absence of diseases for S13D1 and S13D2; and less diseases for S15D2 and N15D2; overall more or less good fertility; see Table 2). The S13D1 and S13D2 assays were used for the evaluation of phenology, dynamics of plant biomass, height, number of leaves, yield, and yield components; S15D2 and N15D2 were used only for phenology, yield, and yield components. The dynamic measurements on these trials had been abandoned because of a weak development and heterogeneities observed on some plots. Parameterization was done as described by Ndiaye (2019). Details of further model parameters and their function and default values are provided in https://umr-agap.cirad.fr/nos-recherches/equipes-scientifiques/modele-samara.
2.3 Choice of Traits of Interest and Genotypic Parameters Used for Optimization
SAMARA, like most crop models, involves many parameters whose values need to be known or estimated so that the model can function correctly. There are 76 such parameters in SAMARA (Dingkuhn et al., 2013). Some have little influence; others are relatively well-known and not very variable, so that it is not necessary to optimize all of them. A choice must therefore be made for ideotype design based on the characteristics of interest (i.e., the output variables of the model) to be optimized and the sensitivity of these variables to the parameters that influence them. Thus, the choice of the parameters on which optimization for ideotype design must be done is determined either by expert opinion or based on field and modeling studies carried out beforehand for the identification of the model parameters to which the traits of interest are sensitive, in this case, grain and biomass yields.
Our previous study on the associations and plasticity of traits contributing to both grain and biomass yields (Ndiaye, 2019) highlighted four traits that could improve the yield potential of dual-purpose sorghum thanks to their strong correlation with grain and biomass yields. These traits are the number of green leaves, stem diameter, internode length, and number of grains per panicle (strongly correlated to grain yield). We defined the parameters of the SAMARA model that correspond to these traits of interest and whose values affect the output variables of interest (i.e., grain yield and biomass yield). For the optimization, the genotypic parameters of the model used were similar to the traits explained above. They are coefficient of sensitivity to leaf mortality (CoeffLeafDeath) for the number of green leaves, structural mass of the internode (CoeffInternodeMass) for the stem diameter, maximum length of the internode (InternodeLengthMax) for the internode length, and structural mass of the panicle (CoeffPanicleMass) for the number of grains per panicle. Additionally, the maximum root length (RootFrontMax) was considered because of its importance in the studied environments, which are often confronted with water stress. The range of values of these parameters (minimum and maximum values) based on the minimum and maximum values observed during the experiments or on the range of values of the parameters derived from the genotype calibration is given in Table 3.
Parameter | Definition | Unit |
Range of variation
|
RootFrontMax | Maximum root length | mm | 200–2000* |
CoeffLeafDeath | Coefficient of sensitivity to leaf mortality | – | 0-0,03 |
CoeffInternodeMass | Structural mass of the internode | g.mm-1 | 0.005–0,1 |
InternodeLengthMax | Maximum length of the internode | mm | 80-500 |
CoeffPanicleMass | Structural mass of the panicle | fraction | 0,12–0,3 |
Note. *Range of variation given in the SAMARA model
2.4 Optimization Method to Define Dual-Purpose Sorghum Ideotypes
All three experimental sites were used as target environments for the optimization of dual-use sorghum ideotypes. For each environment, 67 years of site-specific daily weather conditions were used for the simulations: official data of the national meteorological division over the period 19502016 cleaned by ISRA-CERAAS and comprising average rainfall, maximum and minimum temperatures, solar radiation and insolation, minimum and maximum humidities, wind speed at 2 m from the ground, and potential evapotranspiration (FAO method). Each environment is also characterized by its soil type, its technical itinerary (resulting from our experiments), and by a sowing date based on the rainfall criterion used by Sambakhé (2018), for example, a cumulative rainfall of 30 mm over 3 consecutive days. This criterion is an adaptation of the 20 mm over 3 days criterion usually used in West Africa (Balme et al., 2005) to secure sorghum emergence. Indeed, in the target environments, and in general in West Africa, increasing instability in the start dates of the rainy seasons with dry sequences often necessitates reseeding.
Given its dual-use capabilities, the genotype Nieleni was considered as our reference and used to define the initial genotypic parameters required for the SAMARA model (Ndiaye et al., 2019). The values from the calibration were used. Then we optimized for each target environment the five genotypic parameters that were described in the previous section to define the typical ideotype of each of these environments. For this optimization, the minimum and maximum values (range of variation for optimization) were defined according to the minimum and maximum values observed in all our experiments for the Nieleni genotype (see Table 3).
For this purpose, the Rgenoud genetic optimization algorithm (Package R) was coupled to the SAMARA model (Sambakhé, 2018) to determine the optimal values of the genotypic parameters for the best performances (maximum grain and straw biomass yields) of sorghum ideotypes in a target environment. We optimized the five parameters chosen over the 67 years of climate in each target environment. This made it possible to define the ideotype, per target environment, with the highest average yield (grain and biomass) over 67 years and the lowest coefficient of variation. Thus, we obtain one ideotype per target environment with a high and stable grain and biomass yield. The P function to be optimized parameter was defined as follows:
P = max {Simulated average yield of 67 years for a Minimum coefficient of variation} (1)
2.5 Analysis of Ideotype Performance
A scatterplot was performed to compare the reference genotype Nieleni and the ideotype resulting from the model optimization across the target environments. For this, the reference genotype and the reference ideotype for each target environment over the 67 years were first simulated. Then, to compare the performance of Nieleni and the ideotype in terms of grain and straw biomass production, a potential index (IPO) for grain (or straw biomass) production for a given target environment was used
(2)
where IPO = potential index of a given genotype or ideotype i for the production of grain or straw biomass for a given target environment j; Yij = grain (or biomass) yield of a given genotype or ideotype i in a given environment j over the 67 years; Y̅ = overall average grain (or straw biomass) yield of the two genotypes over the same period across the different target environments.
Thus, for a given target environment j, a positive IPO (IPO>0) of a given genotype or ideotype i for grain (or for straw biomass) indicates a good aptitude of this genotype or ideotype i for grain (or for straw biomass) production for this target environment j (and conversely if the IPO is negative). A positive (or negative) IPO for both grain and straw biomass will therefore indicate a good (or poor) dual-production ability (grain and straw biomass) for the genotype or ideotype i for the given target environment j.
Results
1. Genotypic Parameters of Ideotypes for Dual Purpose
Simulation results for the identification of optimal genotypic parameters for the new ideotypes in the three target environments are presented in Table 4. To obtain a high and stable dual production, regardless of the target environment, the following traits are key: the stay-green of the leaves must be increased with a decreasing coefficient of leaf mortality value; the structural mass (stem reserve); and maximum stem length with increasing structural mass of the internodes and the maximum internode length and the grain yield potential with increasing structural mass of the panicle. An increase in leaf greenness, stem reserve, and structural mass of panicles is therefore necessary at Bambey, Sinthiou Malem, and Nioro du Rip to obtain high and stable yields.
Genotypic parameters optimized for the three ideotypes ranged from zero to 0.0001 for the sensitivity coefficient of leaf mortality (CoeffLeafDeath), between 0.078 g.mm-1 and 0.092 g. mm-1 for the structural mass of the internodes (CoeffInternodeMass), between 222.5 mm and 275.6 mm for the maximum internode length (InternodeLengthMax), and between 1300 mm and 1775 mm for the maximum root length (RootFrontMax). The structural mass of the panicle (CoeffPanicleMass) was 0.3 for all three ideotypes. And the coefficient of leaf mortality remained close to zero for all the ideotypes designed.
Importantly, to achieve high and stable double yields at Bambey—the environment receiving the least rainfall—it is necessary to maintain the stay-green character and maximum root length to minimize the effects of water stress. It is also important to note that the structural mass of the panicle has remained constant regardless of the environment and that the more unfavorable the environment, the thicker but shorter the stems are.
Root | Coeff | Coeff | Internode | Coeff | |
Parameter | Front | Leaf | Internode | Length | Panicle |
Max | Death | Mass | Max | Mass | |
Nieleni | 1500 | 0,0054 | 0,068 | 196,0 | 0,25 |
Id_Sinthiou_Malem | 1300 | 0,0001 | 0,078 | 275,6 | 0,3 |
Id_Nioro_du_Rip | 1402 | 0,0001 | 0,08 | 245,0 | 0,3 |
Id_Bambey | 1775 | 0 | 0,092 | 222,5 | 0,3 |
Note. Id = Ideotype
2. Agronomic Performance of Sorghum Ideotypes and Suitability for Dual Purpose
The average grain and straw biomass yields of the ideotypes and the reference genotype (Nieleni) are presented in Table 5. In general, ideotype yields are higher at Sinthiou Malem and Nioro du Rip compared to Bambey. Simulated grain yields were 4345 Kg.ha-1, 4282 Kg.ha-1, and 3339 Kg.ha-1 for the ideotype in Nioro du Rip, Sinthiou Malem, and Bambey, respectively compared to 2996 Kg.ha-1, 3173 Kg.ha-1, and 2292 Kg.ha-1 for the reference genotype. Thus, the optimized ideotype can potentially produce 29%, 45%, and 46% more grains respectively than the reference genotype in these different environments. For straw biomass, the simulated yields for the ideotype and the reference genotype were of the same order of magnitude in each target environment. It varied, depending on the environment, between 19725 Kg.ha-1 and 28670 Kg.ha-1 for the ideotype and between 19893 Kg.ha-1 and 28343 Kg.ha-1 for the reference genotype. The optimization of the ideotypes was mainly in the direction of grain improvement.
Figure 1 presents its indices of aptitude for grain and straw biomass production in the three target environments for each genotype. It can be noted that in Sinthiou Malem, the optimization was favorable for the double production (much improvement for grain and a little for biomass compared to Nieleni); in Nioro du Rip, it played on grain only (but very strongly); and in Bambey, it also strongly focused on grain yield but to the detriment of the biomass.
Environment | Genotype | Straw biomass yield (Kg.ha-1) | Grain yield (Kg.ha-1) |
Bambey | Ideotype | 19725 ± 3894 | 3339 ±1090 |
Bambey | Nieleni | 19893 ± 4369 | 2292 ± 1479 |
Nioro_du_Rip | Ideotype | 24858 ± 3391 | 4345 ± 526 |
Nioro_du_Rip | Nieleni | 24824 ± 3866 | 2996 ± 727 |
Sinthiou_Malem | Ideotype | 28670 ± 3242 | 4282 ± 288 |
Sinthiou_Malem | Nieleni | 28343 ± 3725 | 3173 ± 425 |
Figure 1
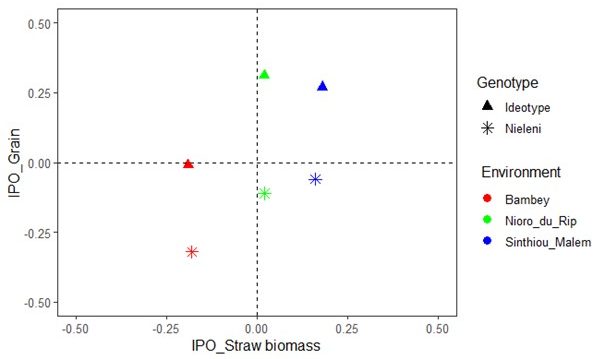
Discussion
Crop growth modeling can guide breeding line selection by studying in silico the performance of trait combinations and by identifying the best combinations and the optimal candidate genotypes for a target environment. Our results at Bambey, Sinthiou Malem, and Nioro du Rip using the SAMARA model show that a substantial increase in dual production, especially for grain, is possible for a given genotype and environment by optimizing its genotypic parameters through a combination of optimization rules and simulations of the crop model based on the historical data of this environment. The SAMARA model confirms that traits such as maximum root length, stay-green, internode length, stem structural mass, and panicle structural mass should be considered in the identification of dual-purpose ideotypes for the environments targeted. Additionally, the range of optimal values for each of these traits was determined for each environment.
1. Importance of the Genotypic Parameters of Optimized Ideotypes
This study showed that dual-purpose sorghum ideotypes should have a deeper maximum root length, especially in environments subject to water stress at the end of the growth cycle and/or with deep, sandy-dominated soils such as those of Bambey. In this study, the maximum root lengths of the designed ideotypes ranged from 1300 mm to 1775 mm. These values are greater than those reported by Chopart (1980) who showed, while studying the sorghum root system in Senegal, that the maximum root length varied between 1100 mm and 1300 mm. Such difference could be explained by the type of variety used, as dual-purpose varieties require more resources from the substrate, especially water, but also by the shortening of the rainy season observed in the past few years. This rainfall decrease that is accentuated by the climatic changes requires plants to develop more robust root systems (deep) for a better adaptation to dry environments. Chopart (1980) also emphasized that the new genotypes that are more efficient in terms of yield potential should not have root systems that are less well-developed than the existing varieties. Similarly, Sambakhé (2018), in defining grain sorghum ideotypes in Senegal, showed the importance of long roots for stabilizing yields in different target environments—Bambey in particular. In our environments affected by a low level of soil fertility and irregular rainfalls, the speed of progression of the root front and the degree of soil colonization by the roots are two important traits that also should be considered (Chopart, 1980).
It also appeared that dual-purpose sorghum ideotypes should have a high number of green leaves that are maintained as long as possible. This trait of interest depends on the total number of leaves—which is conditioned by the speed of leaf production (the phyllochrone)—and on the capacity of the plant to maintain its leaves green (stay-green character), especially during grain filling. Thus, the yield improvements observed in the ideotypes we have designed could be due to the improvement in the efficiency of light interception and conversion (because of the maintenance of the leaves in a green state), which makes it possible to maintain significant assimilate production for a longer period of time and consequently to cope more easily with water stress at the end of the season (Kamal et al., 2019), given that transpiration is well controlled. Consequently, to have sorghum with a good double production (grain and straw biomass) at Bambey, maintenance of a significant number of leaves in the stay-green state is essential (coefficient of leaf mortality equal to 0). The importance of keeping sorghum leaves photosynthetically active during the grain filling period has been reported as an adaptation factor to water-limiting environments (Borrell et al., 1999, 2000; Haussmann et al., 2002; Jordan et al., 2003). Additionally, all ideotypes developed had very high panicle structural mass (high potential kernel count) of 0.30 (see Table 4), which predicts a high yield potential as reported by Hassan and Mohammed (2015), who found that large panicles were associated with a high potential for grain number and grain yield. Hence, a high structural mass is advantageous in arid environments (Bambey) or in environments where fungal diseases are prevalent (Nioro du Rip). In addition, a long stem internode length should be favored, particularly in environments with good soil fertility (predominantly clay) and good rainfall distribution, such as those in Sinthiou Malem. Indeed, a positive association between plant height and grain yield has been reported for sorghum (Jordan et al., 2003), probably because of a greater efficiency in the use of radiation (George-Jaeggli et al., 2004). In addition, if the demand for grain assimilates exceeds the production through photosynthesis, an accelerated translocation of assimilates from stems and leaves occurs (Triboi & Triboi-Blondel, 2002).
2. Involvement for Adaptation in our Target Environments for Breeders and Agronomists
This study shows that the double production of sorghum (grain and fodder) could be significantly increased in these target environments if new ideotypes, superior to Nieleni (especially for grain production), are developed and implemented. Rötter et al. (2011) suggested that to cope with adaptation in the current environments, only new genotypes aimed at increasing yield potential and drought tolerance, combined with adapted agronomic practices, such as the modification of sowing dates, adequate management of nitrogen fertilizers, and plant protection, would allow yield levels to be maintained and losses avoided.
The use of new breeding methods—such as ideotype definition through modeling to create more appropriate genotypes—should allow better adaptation in the target environments (Dawson et al., 2015). This ideotyping requires an in silico approach to design ideotypes for specific uses in a given context. Thus, this study for the design of varietal ideotypes for the three environments targeted based on traits of interest for sorghum double production, historical meteorological data, and research recommendations (technical itinerary) is only one typical example that applies for our specific environments. This simulation approach can be pursued by varying other input variables (e.g., elements of the technical itinerary, soil, or climate) for the definition of varietal and crop ideotypes (technical combinations that affect the traits of the ideotypes). Thus, this study highlights an innovative approach that appears judicious to accelerate the collaboration between crop modelers, agronomists (ecophysiologists), and breeders. More comprehensive breeding programs that would combine the definition of ideotype by simulation and current selection methods could undoubtedly shorten the time required to create new sorghum genotypes better adapted to our different environments.
3. Limits of the Study
Limitations due to some uncertainties are noted, either in the structure of the model or in the approach used. Indeed, the target environments are considered as limited only by physical pedoclimatic conditions (climate, soil water reserve). We do not know the response of our ideotypes to other constraints, such as limited nitrogen (N) contents. In SAMARA, a module dedicated to nitrogen has not yet been integrated. Thus, we have been unable to consider the N limitation in the design of the ideotypes. N uptake and redistribution of N during the post-anthesis phase could be an important constraint to consider in order to achieve a higher sorghum yield potential. Indeed, as noted in this study, maintaining the green leaf biomass during grain filling can increase the grain yield of sorghum grown under water limiting conditions at the end of the cycle (Kamal et al., 2019). However, the effect of this stay-green trait can be limited by nitrogen deficiency (Borrell et al., 1999; Muchow & Sinclair, 1994; Peng et al., 1995).
In this study, we optimized our ideotypes using the genotype Nieleni as a reference. It is a caudatum type and a very efficient genotype in both grain and biomass production. Optimization could be done with a guinea-type genotype as a reference or guinea and caudatum genotypes simultaneously because the guinea types, although with low harvest indexes, are robust, generally tall, and better adapted to the constraints of the target environments compared to the caudatum types, which are rather sensitive (Ndiaye et al., 2019).
In this study, too, we have optimized our ideotypes based on the available history of meteorological data for the target environments, whereas it might be more judicious to use future climate data from climate scenarios for the design of ideotypes given the threats related to climate change in the target environments we considered. Future research also may use data from a shorter and particular climatic period, such as that from the dry period of 19701990 that affected the Sahel or from the recent period of gradual return of rainfall of 19902010 (Salack et al., 2011).
Finally, another limitation of the study lies in the technical itinerary used in the ideotype design. Indeed, this itinerary being the one recommended by the research, we did not take into account some yield reduction factors that producers face (e.g., constraint of sowing date, limited inputs, etc.). Thus, it might be interesting to conduct the study with an itinerary that considers constraints farmers face when designing ideotypes for target environments and contexts to increase efficiency.
All of these limitations reveal the importance of interdisciplinarity (crop modeling, agronomy, ecophysiology, and breeding) in the design of sorghum ideotypes that perfectly fit a defined use in a given context, and they show the importance of crop modeling as a powerful integrative tool for these explorations. This study is a proof of concept on the potentialities of crop modeling for ideotype explorations. Further simulations are required to cover the diversity of situations encountered in farmers’ fields.
Conclusion
This study aimed to use a crop model to design high-performance and stable dual-production (grain and straw biomass) sorghum ideotypes for the target environments of Bambey, Sinthiou Malem, and Nioro du Rip. The results revealed that under our current soil and climate conditions, sorghum ideotypes for dual purpose (grain and fodder) should have a thick stem, large panicles, and a high capacity for deep soil colonization by roots, especially in water-stressed environments such as in Bambey. This study also emphasized the importance of stay-green, especially in the least-watered areas such as Bambey, where the coefficient of leaf mortality is 0. Additionally, this study allowed us to quantify the optimal range of the traits considered for each environment targeted, which can greatly facilitate varietal choice.
Thus, our designed ideotypes can be a decision support tool for increasing productivity in these target environments. The conclusions of the study are potentially interesting for sorghum breeding programs, as they are part of the first step in the design of genotypes adapted to a target environment, namely the definition of the ideotypic traits to be favored. Thus, we recommend that the optimal range of values for these traits are taken into account when identifying appropriate genotypes in sorghum breeding programs for the target environments considered.
References
ANSD (Agence Nationale de la Statistique et de la Démographie). (2018). Bulletin Mensuel des Statistiques Economiques de 2018. Division des Statistiques Economiques, Ministère de l’Economie, des Finances et du Plan, Sénégal, 109 p.
Balme, M., Galle, S., & Lebel, T. (2005). Démarrage de la saison des pluies au Sahel: variabilité aux échelles hydrologique et agronomique, analysée à partir des données EPSAT-Niger. Sécheresse, 16 (1), 15-22.
Borrell, A. K., Bidinger, F. R., & Sunitha, K. (1999). Stay-green trait associated with yield in recombinant inbred sorghum lines varying in rate of leaf senescence. International Sorghum and Millets Newsletter, 40, 31–34.
Borrell, A. K., Hammer, G. L., & Henzell, R. G. (2000). Does maintaining green leaf area in sorghum improve yield under drought? II. Dry matter production and yield. Crop Science, 40(4), 1037–1048.
Chantereau, J., Nicou, R. (1994). Sorghum. The tropical Agriculturist Seres. CTA Wageningen, Netherlands; Macmillan, London Pmid: 24186186.
Chopart, J. -L. (1980). Etude au champ des systèmes racinaires des principales cultures pluviales du Sénégal (arachide-milsorghoriz pluvial) [Thèse de Doctorat d’Université, Institut National Polytechnique de Toulouse].
Dawson, I. K., Russell, J., Powell, W., Steffenson, B., Thomas, W. T. B., & Waugh, R. (2015). Barley: a translational model for adaptation to climate change. New Phytologist. 206(3), 913–931.
Dingkuhn, M., Pasco, R., Soulie, J. C. (2013). SAMARA V2.1: Model description and guidelines for calibration, 28 pp.
Donald, C. M. (1968). The breeding of crop ideotypes. Euphytica, 17, 385–403.
FAO, (2009). How to feed the World in 2050. FAO, Rome.
George-Jaeggli, B., Broad, I., Hammer, G. L., & Jordan, D. (2004). Is increased radiation use efficiency in sorghum related to increased height? In T. Fischer, N. Turner, J. Angus, L. McIntyre, M. Robertson, A. Borrell, & D. Lloyd (Eds.), New Directions for a Diverse Planet: Proceedings for the 4th International Crop Science Congress.
Gerstenmier, A., Choho, T. (2015). Fourniture d’intrants agricoles. Conference Feeding Africa: An action plan for African agricultural transformation, 21-23 October 2015, Dakar Senegal.
Hammer, G. L., Kropff, M. J., Sinclair, T. R., & Porter, J. R. (2002). Future contributions of crop modelling—from heuristics and supporting decision making to understanding genetic regulation and aiding crop improvement. European Journal of Agronomy, 18(1–2), 15–31.
Hammer, G., Cooper, M., Tardieu, F., Welch, S., Walsh, B., van Eeuwijk, F., Chapman, S., & Podlich, D. (2006). Models for navigating biological complexity in breeding improved crop plants. Trends in Plant Science, 11(12), 587–593.
Hammer, G. L., van Oosterom, E., McLean, G., Chapman, S. C., Broad, I. J., Harland, P., & Muchow, R. C. (2010). Adapting APSIM to model the physiology and genetics of complex adaptive traits in field crops. Journal of Experimental Botany, 61(8), 2185–2202.
Hansen, J. W. (2002). Realizing the potential benefits of climate prediction to agriculture: issues, approaches, challenges. Agric. Syst. 74, 309–330.
Hassan, S. A., & Mohammed, M. I. (2015). Breeding for dual purpose attributes in sorghum: Identification of materials and associations among fodder and grain yield and related traits. Journal of Plant Breeding and Crop Science, 7(4), 94–100.
Haussmann, B., Mahalakshmi, V., Reddy, B., Seetharama, N., Hash, C., & Geiger, H. (2002). QTL mapping of stay-green in two sorghum recombinant inbred populations. Theoretical and Applied Genetics, 106, 133–142. https://doi.org/10.1007/s00122-002-1012-3
ICRISAT: Sorghum [Internet]. 2009. Patancheru (AP): International Crops Research Institute for the Semi-Arid Tropics. https://www.icrisat.org/what-we-do/crops/sorghum/sorghum.htm
Jordan, D., Tao, Y., Godwin, I., Henzell, R., Cooper, M., & McIntyre, C. L. (2003). Prediction of hybrid performance in grain sorghum using RFLP markers. Theoretical and Applied Genetics, 106, 559–567.
Kamal, N. M., Gorafi, Y. S. A., Abdelrahman, M., Abdellatef, E., & Tsujimoto, H. (2019). Stay-green trait: a prospective approach for yield potential, and drought and heat stress adaptation in globally important cereals. International Journal of Molecular Sciences, 20(23), 5837. https://doi.org/10.3390/ijms20235837
Kouressy, M., Traoré, S., Vaksmann, M., Grum, M., Maikano, I., Soumaré, M., Traoré, P. S., Bazile, D., Dingkuhn, M., & Sidibé, A. (2008). Adaptation des sorghos du Mali à la variabilité climatique. Cahiers Agricultures, 17(2), 95–100.
Muchow, R. C., & Sinclair, T. R. (1994) Nitrogen response of leaf photosynthesis and canopy radiation use efficiency in field-grown maize and sorghum. Crop Science, 34(3), 721–727.
Ndiaye, M. (2019). Modélisation d’idéotypes variétaux de sorgho sur la base de la caractérisation du fonctionnement et potentiel de sorgho multi-usages au Sénégal [Thèse de Doctorat d’Université, Université Cheikh Anta Diop de Dakar].
Ndiaye, M., Adam, M., Ganyo, K. K., Guissé, A., Cissé, N., & Muller, B. (2019). Genotype-environment interaction: trade-offs between the agronomic performance and stability of dual-purpose sorghum (Sorghum bicolor L. Moench) genotypes in Senegal. Agronomy, 9(12), 867. https://doi.org/10.3390/agronomy9120867
Peng, S., Cassman, K. G., & Kropff, M. J. (1995). Relationship between leaf photosynthesis and nitrogen content of field-grown rice in tropics. Crop Science, 35(6), 1627–1630.
Passioura, J. B. (1996). Simulation models: science, snake oil, education, or engineering. Agronomy Journal, 88(5), 690–694.
Quilot-Turion, B., Ould-Sidi, M.-M., Kadrani, A., Hilgert, N., Génard, M., & Lescourret, F. (2012). Optimization of parameters of the “Virtual Fruit” model to design peach genotype for sustainable production systems. European Journal of Agronomy, 42, 34–48.
R Development Core Team. (2015). R: A Language and Environment for Statistical Computing. R Foundation for Statistical Computing.
Reynolds, M., Bonnett, D., Chapman, S. C., Furbank, R. T., Manès, Y., Mather, D. E., & Parry, M. A. J. (2011). Raising yield potential of wheat. I. Overview of a consortium approach and breeding strategies. Journal of Experimental Botany, 62(2), 439–452.
Rötter, R. P., Carter, T. R., Olesen, J. E., & Porter, J. R. (2011). Crop-climate models need an overhaul. Nature Climate Change, 1, 175–177.
Salack, S., Muller, B., Gaye, A. T. (2011). Rain-based factors of high agricultural impacts over Senegal. Part I: integration of local to sub-regional trends and variability. Theoretical and Applied Climatology 106, 1–22.
Sambakhé, D., (2018). Recherche de carte d’idéotypes de sorgho d’après un modèle de culture: optimisation conditionnelle à l’aide d’un métamodèle de krigeage. [Thèse de Doctorat d’Université, Université de Montpellier 1].
Semenov, M. A., & Halford, N. G. (2009). Identifying target traits and molecular mechanisms for wheat breeding under a changing climate. Journal of Experimental Botany, 60(10), 2791–2804.
Semenov, M. A., & Shewry, P. R. (2011). Modelling predicts that heat stress, not drought, will increase vulnerability of wheat in Europe. Scientific Reports, 1, 66. https://doi.org/10.1038/srep00066
Sinclair, T. R., & Seligman, N. G. (1996). Crop modelling: from infancy to maturity. Agronomy Journal, 88(5), 698–704.
Tardieu, F. (2003). Virtual plants: modelling as a tool for genomics of tolerance to water deficit. Trends in Plant Science, 8(1), 9–14.
Tardieu, F., & Tuberosa, R. (2010). Dissection and modelling of abiotic stress tolerance in plants. Current Opinion in Plant Biology. 13(2), 206–212.
Triboi, E., & Triboi-Blondel, A.-M. (2002). Productivity and grain or seed composition: a new approach to an old problem. European Journal of Agronomy, 16(3), 163–186.
Vanlauwe, B., Bationo, A., Chianu, J., Giller, K. E., Merckx, R., Mokwunye, U., Ohiokpehai, O., Pypers, P., Tabo, R., Shepherd, K. D., Smaling, E. M. A., Woomer, P. L., Sanginga, N. (2010). Integrated soil fertility management: Operational definition and consequences for implementation and dissemination. Outlook Agriculture, 39, 17–24.
White, J. W., Hoogenboom, G., Kimball, B. A., & Wall, G. W. (2011). Methodologies for simulating impacts of climate change on crop production. Field Crops Research, 124(3), 357–368.