4 Counterfeit Herbicides, Productivity and Family Labor Use on Farms in Mali: A Multivalued Treatment Approach
Abstract
Rapid growth in private sector herbicide imports has led to a dramatic rise in use of these commercial products by Malian smallholder farmers. Given weak regulatory capacity to monitor markets, the recent proliferation in herbicide products and brands has been accompanied by widespread sales of unregistered products. We test the effects of herbicides applied to Mali’s major dryland cereals, sorghum, and maize, on yield and labor use per ha, differentiated by gender and age. We employ a multivalued treatment model with data collected from 623 households and 1,273 plots. We find that registered herbicides provide significant productivity benefits to farmers. Registered herbicides reduce the intensity of weeding labor of adult males nearly twice as much as unregistered herbicides, also reducing the weeding labor of female and child household members. Unregistered herbicides negatively affect maize and sorghum yields, while registered herbicides control weed damage without reducing yield.
Keywords: herbicides, multivalued treatment, agricultural policy, Mali
Introduction
Raising the productivity of dryland cereal crops among smallholder farmers in Mali depends critically on their use of modern inputs combined with practices that protect soil and water resources. Yet, despite long-term investments in seed improvement and more recently, government subsidy programs for fertilizer, adoption rates for these inputs remain low on dryland cereals (Kelly et al., 2015; Theriault et al., 2018). In contrast, spurred entirely by commercial supply and farmer demand, herbicide use by smallholder farmers has proliferated. The number of herbicides imported has more than doubled since 2000, even though farmers pay the full commercial price (Haggblade et al., 2017b; INSTAT, 2016).
Rising rural wages, urbanization, and market access are thought to underpin these patterns across Sub-Saharan Africa (Sheahan & Barrett, 2017; Tamru et al., 2017). Similar growth in herbicide use is apparent in other developing regions as well, driven by growing availability of generic products and falling prices (Haggblade et al., 2017a). In China, as in Africa, herbicide use has increased rapidly since the mid-2000s because of rising rural wage rates and falling herbicide prices (Huang et al., 2017).
In Mali, as is often the case elsewhere, not all of these herbicides are registered. While regulators effectively screen new herbicide products for efficacy and safety prior to registration, monitoring of the quality of herbicides actually sold in local markets following registration remains weak. As a result, a large volume of unregistered herbicides finds its way into Mali’s agricultural input markets. Recent market surveys indicate that roughly a quarter (24%) of herbicides sold in Mali are unregistered (Haggblade et al., 2019b). Moreover, laboratory analysis of the most widely used herbicide active ingredient suggests that unregistered herbicides are underdosed by about 8–10% compared to duly registered products (Haggblade et al. 2019a). Broadly speaking, counterfeit and low-quality agricultural inputs are thought to be widespread in Sub-Saharan Africa (Ashour et al., 2018; Bayoumi, 2021; Bold et al., 2017; Gharib et al., 2021; MirPlus, 2012; Yao, 2020).
The survey data from the Sudanian Savanna, an area of high productivity potential for sorghum and maize, demonstrates that most Malian farmers who apply herbicides do not know the difference between registered and non-registered products and that many of the herbicides they use are not registered. Clearly, a situation like this has important ethical considerations in addition to economic implications. Misuse of herbicides has consequences for on-farm yields and potential negative externalities for human and environmental health. Counterfeit inputs have caused considerable problems in fertilizer and seed use, but there has not been much analysis of this problem with respect to herbicides (Ashour et al., 2018).
Published analysis of herbicide use on farms in Sub-Saharan Africa also remains limited. A recent analysis by Haggblade et al. (2017b) examined the origins and consequences of the rapid growth in herbicide use in Mali, finding adoption rates that range from 25% of farmers in remote areas to 75% of farmers in areas better served by market and road infrastructure. The authors found that on average, herbicides costs 50% less per ha than hiring labor to weed and documented a strong relationship between adoption and rising wage rates for weeding labor. In Ethiopia, Tamru et al. (2017) found a similar rapid rise in herbicide use that was driven by the private sector, strongly correlated with access to all-weather roads, and contributed to significantly higher labor productivity on farms. Sheahan and Barrett (2017) documented overall levels of input use in several countries of Sub-Saharan Africa, including agro-chemicals (i.e., insecticides, herbicides, fungicides). Among the six countries examined, Ethiopia and Nigeria showed substantial use of herbicides in particular. Sheahan et al. (2017) demonstrated the positive relationship of agro-chemical use with value of agricultural output, along with its negative consequences for indicators of human health. They analyzed nationally representative datasets from Ethiopia, Tanzania, Uganda, and Nigeria, finding the rate of use of agro-chemicals surpassed 10% of growers.
This chapter contributes to an emerging, still sparse knowledge about the use of herbicides by smallholder farmers in Sub-Saharan Africa, and in particular, to information about counterfeit herbicides. We contribute to the body of research cited above in several ways. First, we tested the effect of herbicide product quality on yield and labor productivity on Malian farms. In the absence of accredited testing laboratories in Mali, we use registered as compared to non-registered herbicides as a proxy for herbicide quality. Via registration of the product, we also tested the effects of regulations.
Second, we are able to disaggregate labor intensity, which we measured as weeding labor per ha, by gender and age (i.e., male, female, and child labor). The heavy demand for female labor generated by a combination of domestic and farm work has long been considered an impediment to their productivity in Sub-Saharan Africa, although Palacios-Lopez et al. (2017) recently questioned women’s share of farm labor.
Third, to test our hypotheses, we applied a multivalued treatment effects model to observational data collected in 58 villages of Mali’s Sudanian Savanna zone from 2014 to 2015. Many treatment effects in agricultural development involve binary assignment (e.g., program participation or not). In the case of binary treatment when employing observations in cross-sectional data, matching has become a popular approach (Imbens & Wooldridge, 2009), especially given the challenges of identifying appropriate instruments for two-stage least squares analysis. To our knowledge, propensity score matching methods have not yet been fully extended to multivalued treatments. Multivalued models have been described by Wooldridge (2010) and Cattaneo (2010), but so far, we have found only one application in agriculture (Esposti, 2017). Here, we tested three multivalued effect estimators with regression methods and, for completeness, used propensity score matching to test the overall effects of herbicide use.
Methods
1. Data
The sampling frame is a baseline census of all 2,430 sorghum-growing households in 58 villages located in the Cercles of Kati, Dioila, and Koutiala, regions of Koulikoro and Sikasso. Sikasso and Koulikoro regions lie largely within the Sudan Savanna zone, which has the greatest yield potential in Mali (Dicko et al., 2017). These two regions constitute the “sorghum belt” of the nation in terms of area cultivated and total production; as of the 2012–2013 season, the year before our survey, the two regions represented more than 51% of total sorghum area planted in the country (Cellule de Planification Statistique du Secteur du Développement Rural [CPS-SDR]). The zone is also favorable for maize production, an increasingly popular food and cash crop.
The enumeration unit in the baseline census, and generally in Mali, is the Exploitation Agricole Familiale (EAF), also known as the family farm enterprise. According to the national agricultural policy act (Loi d’Orientation Agricole), the EAF is a production unit composed of several members who are related and who use production factors collectively to generate resources under the supervision of the head of household. The head can be a female or male household member, but female headship remains rare. The head’s primary economic activity is to encourage the optimal use of production factors, as these are defined by the extended family. For the EAFs we studied, the first priority was universally food security. The head represents the EAF in all civil acts, including representation and participation in government programs. They may designate a team leader (chef de travaux) to supervise field work and manage the EAF on behalf or to assist the head when they have physical or other limitations.
The family farm enterprise is a complex organization that consists of numerous plots on which multiple crops are grown. Plots are managed collectively and individually by various family members. Members generally include the head, their spouse and children, married sons and their wives and children, unmarried daughters and brothers of the head, and other relatives. Collective plots belonging to the whole EAF are managed by the household head or the team leader on behalf of the EAF. Individual plots belong to the EAF but are planted and managed by individual members, including both men and women. The production from these plots is not managed collectively. At each cropping season, the head distributes individual plots based on the needs of the family.
Sorghum and maize are the major dryland cereals in this region of Mali, although some millet is also grown. For more detailed analysis of input use in sorghum and maize production, we drew a sample of EAFs with simple random sampling. The final sample size was 623 EAFs, with an overall sampling fraction of 25%. Enumerators inventoried all plots operated by each sampled EAF, grouping them by crop and plot management type. Considering sorghum and maize plots only (because of budget constraints), one plot was randomly sampled per management type per EAF. The total sample of sorghum and maize plots analyzed, including those collectively and individually managed, was 1,273. We controlled for plot manager in the analysis, and included all sorghum and maize plots, having obtained more detailed production data compared to other crops.
The multi-visit sample survey was conducted in four rounds from August 2014 through June 2015, with a combination of paper questionnaires and computer-assisted personal interviews, by a team of experienced enumerators employed by the Institut d’Economie Rurale. Modules included (a) inventories of plots, livestock, agricultural equipment, and household assets, utilizing the harvest from the previous season; (b) input use and labor use on sorghum and maize plots; (c) measurement of area and production on sorghum and maize plots; (d) consumption expenditures and migration remittances.
2. Econometric Strategy
Our aim was to test the impacts of counterfeit and registered herbicides, as compared to use of neither, on farm productivity (output per ha) and labor intensity (weeding labor per ha). We further differentiated labor intensity by gender and age.
Herbicide use has recently expanded in rural Mali, in the absence of any deliberate program or policy intervention. We expected the decision to use herbicides to be non-random in farming communities given that the input was novel and it was introduced entirely by local traders or other farmers. We imagined that at this early stage of adoption, users and non-users would be systematically different. Users may “self-select,” leading to potential bias in estimates of productivity impacts.
The Randomized Controlled Trial (RCT) is often seen as the “gold standard” of evaluation approaches because it eliminates selection bias (Imbens & Wooldridge, 2009). Bias due to non-random selection can occur because of program placement, participation criteria, or through processes of self-selection. Various approaches have been recommended to address the challenge of establishing a counterfactual with non-experimental data, including the class of treatment effect models, which we employed here. These models make treatment and outcome independent by conditioning on covariates or controls.
Various methods were used to address the question of establishing a counterfactual with non-experimental observations, including the class of treatment effect models, most of which involved a single treatment level represented by a binary (0,1) variable. Our interest in distinguishing the effects of herbicide quality (no herbicides at all, counterfeit herbicides, registered herbicides, or 0,1,2 respectively) led us to apply a multivalued treatment effects model. The case of multivalued treatment effects was developed by Wooldridge (2010), Cattaneo (2010), and Cattaneo et al. (2013). Wooldridge (2010) presented the example of participation in a training program that occurred at different levels (part-time or full-time), or in different forms. Cattaneo (2010) developed a more general theory for semiparametric estimators and applied it to estimate quantile treatment effects. The Wooldridge (2010) conceptualization best represents our situation, where 0 represents the control group and higher integer values indicate different treatment options.
To our knowledge, propensity score matching methods have not been fully developed for more than a single value of treatment (Cattaneo et al., 2013). As in the binary case, multivalued treatment effects can be estimated by applying other regression approaches. Wooldridge (2010) recommended regression adjustment (RA) as a baseline approach and a simple extension of the binary case to the multivalued case. RA estimators model the potential outcome of treatment without any assumptions about the treatment model. Augmented, inverse-probability weighted (AIPW) and inverse-probability weighted, regression adjustment (IPWRA) estimators address the outcome model Yi = f ( Xi, β) + εi, as well as the treatment model for 1,…G treatments, Pr (Ti=1,…G) = h (Zi; λ) + ωi.
X is a vector of covariates that influence the outcome Y and Z is a set of covariates explaining treatment assignment T, which may overlap. By exploiting inverse-probability weights derived from propensity scores estimated with multinomial, nested, or ordered logit, these estimators control for selection on observables. We utilized multinomial logit.
When either the outcome model or the treatment model or both are specified correctly, AIPW and IPWRA furnish consistent estimators of the treatment model parameters. Reflecting this property, the AIPW and IPWRA have been termed “doubly robust” (Wooldridge, 2010, p. 930). Cattaneo (2010) referred to the AIPW as the “efficient influence function”. AIPW and IPWRA estimators can be more efficient than RA (Cattaneo, 2010; Cattaneo et al., 2013; Glynn & Quinn, 2010). Use of these approaches in agricultural analyses appears to be infrequent. Esposti (2017) applied the multivalued Cattaneo (2010) model to evaluate the impact of the 2005 reform of the Common Agricultural Policy on farm production choices using treatment intervals.
Identification of the treatment effect relies on satisfying the properties of conditional mean independence, which stipulates that the treatment does not affect the mean of the outcome variable. Apparently, the multivalued case relies on a weaker assumption than in the binary case (Esposti, 2017; Cattaneo, 2010). Among our estimators, weighting by the inverse of the estimated propensity score achieved covariate balance and created a sample in which the distribution of covariates was independent of treatment status (Karamba & Winters, 2015).
Potential bias generated by unobservable characteristics remained. We applied the bounds approach proposed by Rosenbaum (2002) to examine the robustness of our results to these unobserved characteristics. The Rosenbaum bounds approach determines how strongly an unobserved factor must affect the outcome variables to undermine the matching estimates. The technique calculates the confidence intervals for different level of Γ based on the following equation
where Pi and Pj are odds for two individuals i and j. γ is the effect of unobserved factor on the treatment selection
Γ=exp(γ)
The smallest value of Γ for which the confidence interval contains zero is the critical odds ratio for which we questioned the estimated impact. If the value of Γ is small (less than two), we considered that the effect of the estimated treatment was sensitive to unobservable factors (Duvendack & Palmer-Jones, 2012).
We also improved the covariate balance by introducing plot management type and education into the treatment model. Plot management type is highly correlated with observed and intrinsic characteristics of the manager (relationship to head, gender, age) that affect access to resources within the extended family household (EAF). We also examined the common support condition.
In addition to the multivalued models, we estimated a binary model with propensity score matching to test the effects of use vs. non-use of herbicides. All models were estimated in STATA 14 using plot-level data.
3. Model Specification
The objective of the econometric strategy was to quantify the potential outcomes that expressed changes in the output per ha and labor use in weeding per ha. For both outcomes, we used the same econometric specification
Y =α+β’outcomecovar+ Ө’treatmentcovar +µ,
where Y is grain yield in kg/ha or weeding labor/ha and outcomecovar is a vector of agricultural inputs applied on dryland cereals plots, which covary with the outcome. Corresponding to a notional yield response function, we included input quantities per ha (seed, fertilizer, adult male labor, adult female labor, children’s labor, machinery use, manure), as well as plot characteristics (time in minutes to travel from homestead to the plot, whether any structure was built on the plot to offset soil and water erosion). These were the same covariates we expected to influence the intensity of labor use.
Treatmentcovar is a vector of plot manager, household, and market covariates that influence incentives for use (covarying with treatment), including the cost per ha of hiring weeding labor, the total household labor supply, and whether or not the EAF received a fertilizer subsidy. In Mali, access to formalized extension structures (encadrement) substitutes to some extent for commercial markets, influencing farmer access to inputs and services of various kinds, including subsidized fertilizer. The estimate of weeding costs per ha is derived from responses to a question asking how much weeding labor costs if herbicides were not applied. Since market infrastructure extends to weekly fairs conducted in villages, we included a dummy variable for the presence of a weekly fair in the village of the EAF. Finally, as described above, we recognized the social organization of production in this region of Mali, and added the characteristics of the plot manager (education of the manager, whether the plot is managed by the head or another individual member of the household) among our explanatory variables.
Following the potential outcomes framework, we expressed the observed outcome Y as
Y=D0Y0+D1Y1+D2Y2
where D0, D1, and D2 were dummy variables indicating the level of treatment. Dt={0,1,2} equals to one if the plot received treatment level t and zero otherwise. Y0, Y1, and Y2 were the corresponding potential outcomes.
Letting t and s act as two treatment levels, the average treatment effects were given by the following equation:
ATE = E[Yt-Ys]
If we were in a randomized experiment setting, we could estimate the average treatment effect (ATE) of level t relative to level s by the sample means of observed outcomes. Given the observational nature of our data, to estimate ATE we needed to fulfil the weak unconfoundedness or conditional independence assumption, which required independence of each treatment level with the corresponding potential outcomes knowing X. Hence, formally
Dt ⊥Yt|X, ∀t∈ {0,1,2}
where X= outcomecovar + treatmentcovar, ⊥ denotes independence and t treatment level.
In addition, the overlap or common support assumption, which implies positive probability of being in any treatment level, must be satisfied. The overlap assumption in multivalued treatment can be assessed using generalized propensity score (GPS) proposed by Imbens (2000) and computed with the following equation:
r(x,t) = P [T = t|X=x] = E[Dt|X = x]>0
The GPS is used in the empirical implementation because it overcomes the problem of high dimensionality that might arise by conditioning directly on vector X.
Under these assumptions, we estimated the mean of the potential outcome for the treatment level t knowing the vector of covariates X by taking the average of the conditional expectation of the potential outcome or by weighting the observed outcomes with the generalized propensity score through the below equation:
or
The ATEs were then determined by pairwise comparison of these means of the potential outcome.
Model control variables are defined in Table 1. Outcome variable definitions are shown in Table 2, along with differences in the means of each outcome variable by treatment. Without controlling for other factors, lower mean yields were associated with unregistered herbicide use compared with no herbicide use, but the highest mean yields were associated with registered herbicide use. The lowest labor use per ha for adult males, adult females, or children was on plots receiving registered herbicides. In interpreting our labor outcome variables, it was important to recognize that hired labor use on farms surveyed was negligible. Absence of labor markets, and dependence on the extended family or labor sharing arrangements with neighbors, was a prominent feature of the dryland cereals farming system in our zone of study. These were the hypotheses we carried to the estimation.
Control variable | Definition |
Treatment covariates
|
|
individually-managed |
plot managed individually by male or female who is not the EAF head or designate=1, else 0
|
manager |
head=1; individual male not head=2; individual female not head=3
|
education |
plot manager attended primary school=1, 0 else
|
labor supply |
number of adults in EAF between 12 and 55 years of age (inclusive)/total area operated by EAF
|
weeding cost |
cost of hiring weeding labor per ha
|
subsidy |
EAF benefited from fertilizer subsidy=1, 0 else
|
market |
weekly market fair in village=1, 0 else
|
village |
village fixed effect
|
Outcome covariates
|
|
seed |
quantity of seed in kgs used per ha
|
fertilizer |
kgs of fertilizer applied per ha
|
male labor |
number of adult male person-days (14 years and above) per ha
|
female labor |
number of adults female person-days (14 years and above) per ha
|
child labor |
number of children person-days (under 14 years) per ha
|
machinery use |
hours of equipment use her ha
|
manure |
manure use on plot=1, 0 else
|
location |
time in minutes to travel from home to the plot
|
erosion control |
any anti-erosion structure built on plot=1, 0 else
|
Outcomes | Definition | No herbicide | Unregistered herbicide |
Registered herbicide
|
crop productivity | yield=grain kgs harvested/ha | 1183 | 890 | 1346 |
male labor intensity | days weeding labor per ha for adult males; | 21.1 | 20.5 | 17.9 |
female labor intensity | days weeding labor per ha for adult females | 6.13 | 8.01 | 3.77 |
child labor intensity | days weeding labor per ha for children (12 years and under) | 3.38 | 4.18 | 2.83 |
N=1273 plots, 623 EAFs. |
Results
1. Descriptives
Glyphosate, a broad-spectrum, non-selective herbicide which was developed by Monsanto under the trademark name of Roundup, is the world’s most popular herbicide. Importers and input vendors also report that glyphosate is their top-selling herbicide in Mali (Diarra, 2016). Our survey data indicated that glyphosate accounted for about two-thirds of herbicide volumes applied by farmers to their sorghum and maize plots. Selective herbicides, intended for use on cotton, maize, and rice, represented the other third. Since many households surveyed belonged to the cotton growers’ associations, use of selective herbicides may have reflected their experience and access to inputs via their membership. Based on Table 3, only slightly more than half of sorghum and maize plots (53%) or volume of total herbicides used by farmers surveyed (55%) were registered. The remainder were of unregistered and of uncertain quality, meaning either “knock-offs” or counterfeit.
Herbicide type | Herbicide registration | |||
registered | unregistered | total | ||
% of plots | 53 | 47 | 100 | |
% of volume | 55 | 45 | 100 |
Aside from the practice of full soil inversion (plowing) during land preparation, farmers in this region of Mali rely on hand and hoe to control weeds, and generally on the labor of family members or shared labor with friends. When asked what it would cost to hire labor to accomplish this task rather than apply herbicides, surveyed farmers indicated that they would have spent $52. In comparison, those who used herbicides instead would spend an average of only $23 per hectare.
Among households surveyed, female household members (primary wives of the household head, but also daughters-in-law) managed about one-quarter of sorghum plots, but none of the maize plots. Sorghum and maize plots managed by male family members who were not heads of household were relatively few in number, although these men often managed plots of cotton or other cash crops. Larger plots managed by the household head and worked collectively by members constituted over 80% of all sorghum plots and 95% of maize plots.
The data in Table 4 indicates that individual plot managers were more likely to apply herbicides on their plots than household heads were to use the input on family fields. According to social norms, the head reserves the right to demand labor on these plots, since the output benefits the family as a group; individuals must meet labor needs on their own fields after satisfying their duties—potentially, a powerful incentive for using herbicides. Female plot managers applied herbicides on their individual fields at nearly twice the rate applied on collective fields (2.6 liters per hectare compared to 1.1 liters). Male-managed individual plots received doses that were even higher per hectare.
Crop grown | ||||
Plot manager | Plot type | Sorghum | Maize | All |
Percent of plots using herbicides | ||||
Household head | collective | 47 | 69 | 58 |
Female | individual | 79 | 79 | |
Male | individual | 90 | 60 | 80 |
All | 56 | 69 | 61 | |
Herbicide application rate (liters/ha) | ||||
Household head | collective | 1.1 | 1.7 | 1.4 |
Female | individual | 2.6 | 2.6 | |
Male | individual | 3.3 | 2.5 | 3.1 |
All | 1.6 | 1.7 | 1.6 |
2. Econometric Findings
Before discussing the ATEs, it is important to examine the quality of the matching process. Conditional probabilities of herbicide use by treatment level are shown in Figure 1. Across all three treatment levels, the density distribution of the estimated probabilities confirmed that there was not much high-density mass near the values 0 or 1. Thus the common support condition was satisfied: there was substantial overlap in the distribution of the probability for non-users of herbicides, users of unregistered herbicides, and users of registered herbicides.
Figure 1
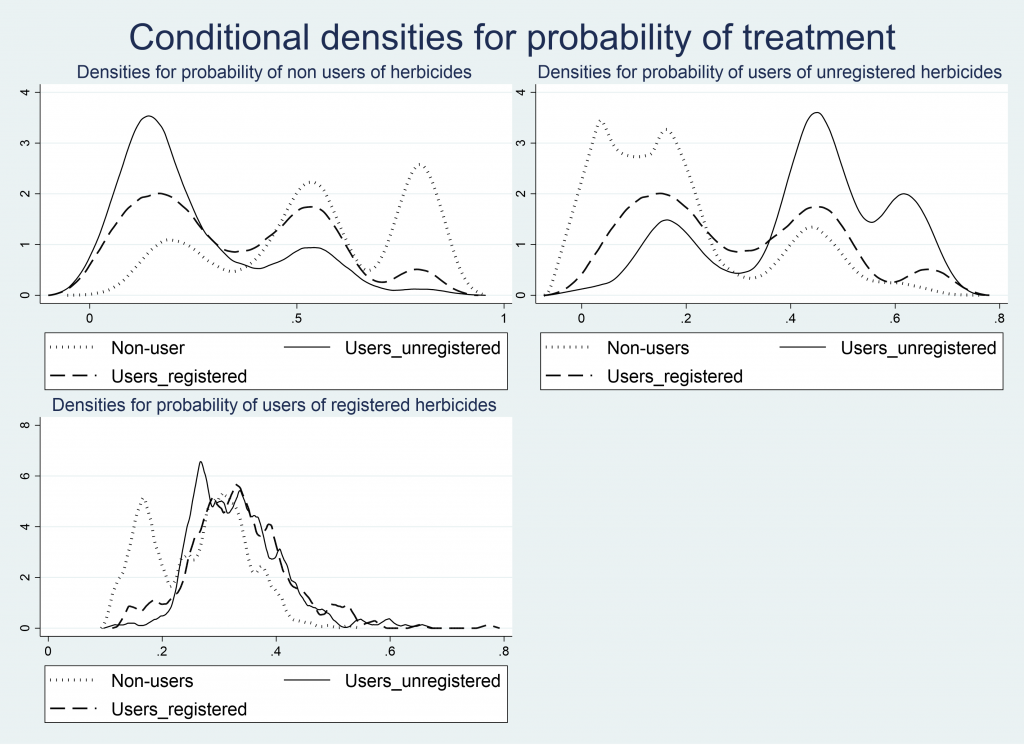
In addition, Figure 2 presents the probability distribution of propensity scores (lowest to highest) and the region of common support for users and non-users. The data indicated that the common support condition was satisfied as there was overlap in the distribution of the propensity scores between the two groups. This is reflected in Figure 3, which shows the probability density functions of users and non-users. The results of the covariate balancing tests in Table 5 show that good balance was achieved after matching by reducing the percentage of balance bias up to more than 70% overall.
Figure 2
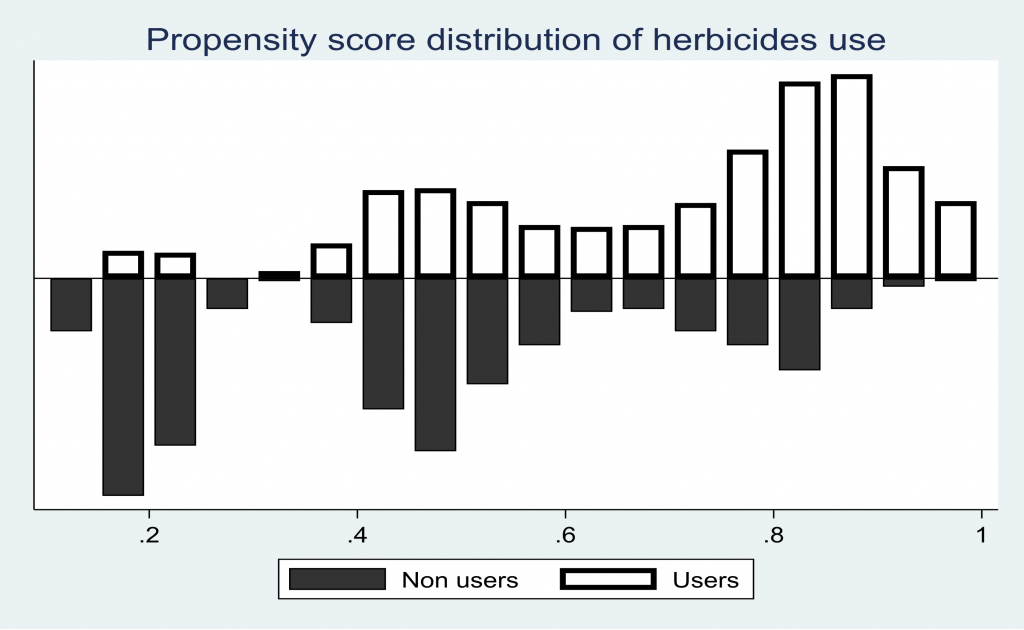
Figure 3
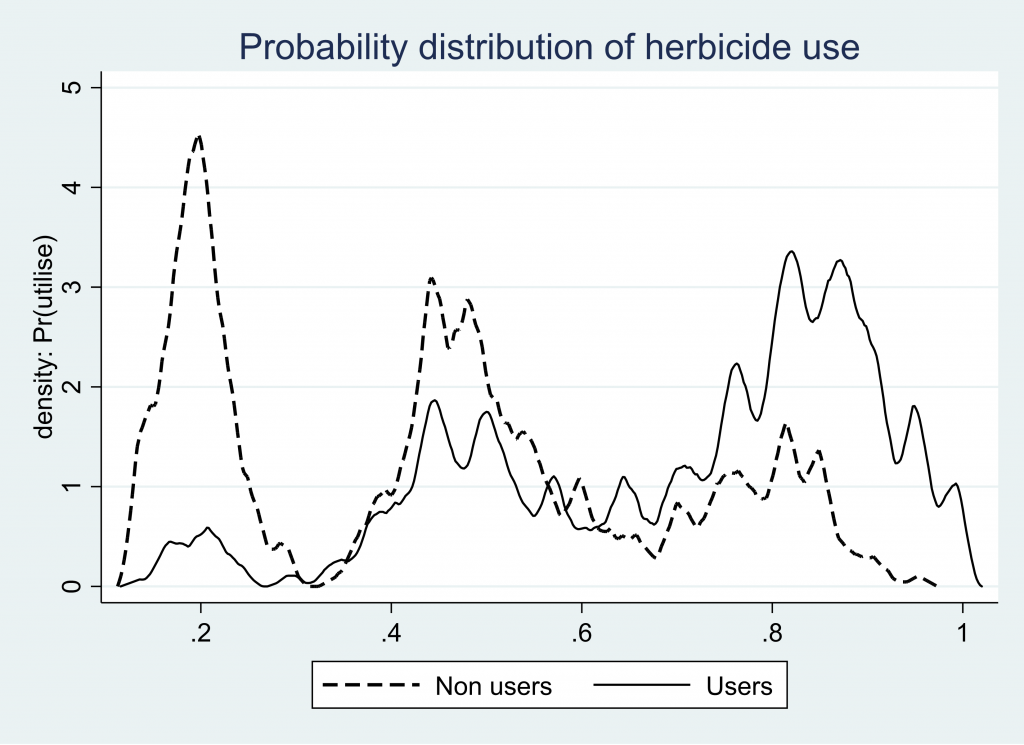
Standardized | Differences | Variance | Ratio | ||
Raw | Weighted | Raw | Weighted | ||
Not registered herbicides
|
|||||
manager | 0.538 | 0.538 | 2.662 | 2.660 | |
village | -1.401 | -1.401 | 0.451 | 0.451 | |
subsidy | -0.283 | -0.283 | 1.158 | 1.157 | |
age | -0.192 | -0.192 | 1.012 | 1.011 | |
education | 0.278 | 0.278 | 1.647 | 1.645 | |
market | -0.301 | -0.301 | 0.654 | 0.654 | |
labor supply | 0.334 | 0.334 | 1.216 | 1.215 | |
weeding cost | 0.732 | 0.732 | 12.777 | 12.768 | |
individually-managed | 0.559 | 0.559 | 2.745 | 2.743 | |
seed | -0.069 | -0.069 | 2.048 | 2.046 | |
fertilizer | 0.031 | 0.031 | 0.891 | 0.890 | |
male labor | -0.141 | -0.141 | 1.043 | 1.043 | |
female labor | -0.192 | -0.192 | 0.569 | 0.568 | |
child labor | 0.194 | 0.194 | 1.742 | 1.740 | |
location | -0.033 | -0.033 | 1.108 | 1.107 | |
erosion control | -0.070 | -0.070 | 0.999 | 0.999 | |
machinery use | -0.313 | -0.313 | 0.667 | 0.666 | |
manure | 0.020 | 0.020 | 1.016 | 1.015 | |
Registered herbicides
|
|||||
manager | 0.189 | 0.189 | 1.600 | 1.599 | |
village | -0.824 | -0.824 | 0.716 | 0.716 | |
subsidy | -0.140 | -0.140 | 1.099 | 1.098 | |
age | -0.072 | -0.072 | 1.022 | 1.021 | |
education | 0.180 | 0.180 | 1.427 | 1.426 | |
market | -0.222 | -0.222 | 0.751 | 0.750 | |
labor supply | 0.318 | 0.318 | 1.156 | 1.155 | |
weeding cost | 0.535 | 0.535 | 21.569 | 21.555 | |
individually-managed | 0.198 | 0.198 | 1.643 | 1.642 | |
seed | 0.180 | 0.180 | 1.887 | 1.886 | |
fertilizer | 0.521 | 0.521 | 2.954 | 2.952 | |
male labor | 0.146 | 0.146 | 1.423 | 1.422 | |
female labor | -0.185 | -0.185 | 0.710 | 0.709 | |
child labor | 0.154 | 0.154 | 1.518 | 1.517 | |
location | -0.172 | -0.172 | 1.092 | 1.092 | |
erosion control | -0.251 | -0.251 | 0.797 | 0.796 | |
machinery use | -0.095 | -0.095 | 0.967 | 0.966 | |
manure | 0.324 | 0.324 | 1.138 | 1.137 |
Furthermore, Table 6 presents the critical values of gamma (Γ) for each of the four outcome variables. The critical gamma values indicated that yield and male labor were sensitive to unobserved characteristics at Γ = 1.4 and Γ = 1.6, respectively. These values suggested that the unobserved characteristic would need to increase the odds ratio by 40% for yield and 60% for male labor before we started questioning the estimated effects of treatment. The degree of vulnerability to unobserved factors for these two outcome variables was within an acceptable range and was not a critical issue (Rosenbaum, 2005). The critical gamma values for female and children labor were greater than two. This result implies that the treatment effects are more robust to the hidden biases arising from unobserved characteristics for these two outcome variables. Our estimates of treatment effects were overall robust to the influence of unobserved factors.
Outcome variables | Gamma | CI+ | CI- |
Yield | 1 | -401.395 | -189.458 |
1.2 | -512.604 | -77.024 | |
1.4 | -609.629 | 16.257 | |
Male labor | 1 | -7.902 | -4.134 |
1.2 | -10.132 | -2.312 | |
1.4 | -12.194 | -0.802 | |
1.6 | -14.034 | 0.524 | |
Female labor | 1 | -0.003 | -0.003 |
1.2 | -0.003 | -0.003 | |
1.4 | -0.004 | -0.003 | |
1.6 | -0.004 | -0.004 | |
1.8 | -2.013 | -0.004 | |
2 | -2.885 | -0.004 | |
2.2 | -3.962 | -0.004 | |
2.4 | -4.878 | 0.553 | |
Children labor | 1 | -0.003 | -0.004 |
1.2 | -0.003 | -0.004 | |
1.4 | -0.704 | -0.004 | |
1.6 | -1.676 | -0.003 | |
1.8 | -2.102 | -0.002 | |
2 | -3.106 | -0.003 | |
2.2 | -3.484 | -0.003 | |
2.4 | -3.896 | 0.549 |
As described in the econometrics section, we applied RA, AIPW, and IPWRA to estimate the impacts of herbicide use on yield and intensity of adult male, adult female, and child labor with a multivalued treatment model (non-use, use of unregistered, and use of registered herbicides). For completeness, we used overall propensity score matching (PSM) to estimate the binary impact of herbicide use compared to non-use.
The results reported in Table 7 showed no significant impact of registered herbicide use on yield. In fact, herbicides reduced damage from weeds rather than enhancing the yield potential of the crop (Lichtenberg & Zilberman, 1986). Still, ceteris paribus, we might have expected a larger harvested output per acre if damages had been offset by use. On the contrary, the regression indicates that the use of unregistered herbicide has a significantly negative effect on harvested yields, controlling for other covariates. Household members who managed plots where they applied unregistered herbicides expected an average yield loss of 218 kg/ha. Considering that the overall mean yield was only 1,141 ha, this amount is important in terms of magnitude. Overall, the binary PSM model confirms that the overall impact of herbicide use on crop yields is not significantly different from zero.
RA | AIPW | IPWRA | PSM | |
ATE on Yield | ||||
not registered | -219.4* | -204.0** | -152.1 | |
registered | 66.1 | 108.2 | 137.3 | |
use herbicide | 1.876 | |||
ATE on men weeding labor
|
||||
not registered | -4.686** | -5.441** | -6.284*** | |
registered | -7.973*** | -8.674*** | -9.327*** | |
use herbicide | -8.061*** | |||
ATE on women weeding labor
|
||||
not registered | -1.055 | -0.92 | -1.318 | |
registered | -1.397 | -1.788* | -2.144** | |
use herbicide | -1.599 | |||
ATE on children weeding labor
|
||||
not registered | -1.62 | -1.785 | -2.279* | |
registered | -1.913* | -2.241** | -2.706** | |
use herbicide | -1.342 | |||
N | 1136 | 1136 | 1136 | 1136 |
* p < 0.10, ** p < 0.05, *** p < 0.01
Impacts on weeding labor appear to be stronger, as might be expected given that labor bottlenecks are the primary incentive for using this input. Among the households surveyed, adult male labor was by far the largest category. The labor of adult male household members was heavily demanded on the collective plots, particularly in land preparation and weeding. The labor of adult female household members was deployed across a broad range of household and farming activities; their individual plots were small in size, and generally intercropped with legumes. The data shows low overall levels of female labor use in weeding. Child labor efforts were also relatively minor compared to those of adult males.
Consequently, the impacts of all categories of herbicide use on the labor use intensity of adult males is strong and positive (corresponding to a negative sign since the variable was labor days per hectare). Registered herbicides had the greatest impact, almost twice as large as unregistered herbicides (an average decrease of 7.9 vs. 4.6 days). The use of registered herbicides was associated with lower amounts of weeding labor by women and children in the household, reducing it by an average of 1.7 and 2.2 days, respectively. This implies that children in households who use registered herbicides are less likely to be asked to weed, which has important ethical and, possibly, health implications.
Conclusions and Policy Implications
This study contributes in general to a sparse, emerging knowledge about the use of herbicides by smallholder farmers in Sub-Saharan Africa and in particular to information about counterfeit herbicides. We found that the use of unregistered herbicides is associated with lower yields and that the use of all herbicides, and in particular, registered products, reduces the intensity of labor use (per ha) of male, female, and child labor in weeding.
Use of registered herbicides improves child welfare by reducing the demand for their labor. Policies and regulations to support the use of registered herbicides could be beneficial, but more research is needed on health and environmental implications. Future research might further test these hypotheses using techniques for quality directly through laboratory testing, and a sample of farmers from more regions of Mali.
Clearly, the large volume of unregistered herbicides currently in use poses a problem for farmers as well as potential problems for consumers and the environment. These findings suggest a need for improved regulatory practices. It is important to distinguish between the pre-registration review system, which works very well, and the post-registration market monitoring, which functions very poorly (Diarra & Haggblade, 2017; Haggblade et al., 2021). For over 20 years, Mali has participated in a regional regulatory review process. Since 1994, the Comité Permanent Inter-Etats de Lutte contre la Sécheresse dans le Sahel (CILSS) has operated a regional regulatory body, the Comité Sahelien des Pesticides (CSP), to review and certify all pesticide products sold throughout the Sahelian member countries, including Mali. Under these common rules, any pesticide passing CSP efficacy and safety reviews and registered for sale in one member country becomes automatically authorized for sale throughout all nine member countries. By centralizing this regulatory review process, the CSP provides a one-stop-shop for manufacturers and importers, facilitating the review process and enabling suppliers to reduce bureaucratic costs by standardizing and centralizing review procedures. This model economizes on scarce technical manpower and laboratory facilities by pooling talent from across the member countries. Specialists at the Food and Agriculture Organization of the United Nations (FAO) and across West Africa consider the CSP regulatory model to be “probably the most successful example of regional harmonization in Sub-Saharan Africa” (Traoré et al., 2011, p.16) and one increasingly emulated in other sub-regions of Africa (Haggblade et al., 2021).
In contrast, post-regulatory monitoring remains very weak. The growing numbers of unregistered and counterfeit herbicide products available on the market lead to mounting farmer concerns about product quality and safety. Yet the environmental impacts of herbicide use remain largely unmonitored in Mali (Haggblade et al., 2017b).
Looking forward, policy makers in Mali will increasingly require better monitoring of pesticide product quality and environmental impact. The CILSS model of regional regulatory review, which economizes on scarce scientific personnel and laboratory facilities, has proven efficient in vetting herbicide products prior to release. Regional sampling and studies across common Sahelian agro-ecological zones can perhaps offer parallel economies in environmental monitoring.
Acknowledgements
The authors thank anonymous reviewers for their comments and suggestions, and gratefully acknowledge the financial support of the Bill & Melinda Gates Foundation under the project titled Guiding Investments in Sustainable Agriculture in Africa (GISAIA), and USAID/Mali under the project titled “Projet de recherche sur les politiques de sécurité alimentaire au Mali (PRePoSAM) awarded under the Food Security Innovation Lab’s Cooperative Agreement Number AID-688-A-16-00001.
References
Ashour, M., Gilligan, D. O., Hoel, J. B., & Karachiwalla, N. I. (2018). Do beliefs about herbicide quality correspond with actual quality in local markets? Evidence from Uganda. Journal of Development Studies, 55(6), 1285–1306. https://doi.org/10.1080/00220388.2018.1464143
Bayoumi, A. E. (2021). Counterfeit pesticides. ACS Chemical Health & Safety, 28(4), 232–237. https://doi.org/10.1021/acs.chas.1c00010
Bold, T., Kaizzi, K. C., Svensson, J., & Yanagizawa-Drott, D. (2017). Lemon technologies and adoption: Measurement, theory, and evidence from agricultural markets in Uganda. Quarterly Journal of Economics, 132(3), 1055–1100. https://doi.org/10.1093/qje/qjx009
Cattaneo, M. D. (2010). Efficient semiparametric estimation of multi-valued treatment effects under ignorability. Journal of Econometrics, 155(2), 138–154. https://doi.org/10.1016/j.jeconom.2009.09.023
Cattaneo, M. D., Drukker, D. M., & Holland, A. D. (2013). Estimation of multivalued treatment effects under conditional independence. Stata Journal, 13(3), 407–450. https://doi.org/10.1177/1536867×1301300301
Cellule de Planification et de Statistique du Secteur Développement Rural (CPS-SDR), Annuaire Statistique Du Secteur Développement Rural au Mali.
Diarra, A. (2016). Rapport de consultation: profil du marché des pesticides et mise en oeuvre des politiques agricoles dans l’espace CEDEAO: Etude de Cas au Mali. (mimeo). Michigan State University.
Diarra, A., & Haggblade, S. (2017). National implementation of regional pesticide policies in West Africa: Achievements, challenges and priorities. Feed the Future Innovation Lab for Food Security Research Paper 81. Michigan State University.
Dicko, M., Koné, M., Traoré, L., Diakité, C. H., Kamissoko, N., Sidibé, B., … Konaré, H. (2017). Optimizing fertilizer use within the context of integrated soil fertility management in Mali. Fertilizer Use Optimization in Sub-Saharan Africa. CABI, Nairobi, Kenya, 100–112.
Duvendack, M., & Palmer-Jones, R. (2012). High noon for microfinance impact evaluations: Re-investigating the evidence from Bangladesh. Journal of Development Studies, 48(12), 1864–1880. https://doi.org/10.1080/00220388.2011.646989
Esposti, R. (2017). The heterogeneous farm-level impact of the 2005 CAP-first pillar reform: A multivalued treatment effect estimation. Agricultural Economics, 48(3), 373–386. https://doi.org/10.1111/agec.12340
Gharib, M. H., Palm-Forster, L. H., Lybbert, T. J., & Messer, K. D. (2021). Fear of fraud and willingness to pay for hybrid maize seed in Kenya. Food Policy, 102, 102040. https://doi.org/10.1016/j.foodpol.2021.102040
Glynn, A. N., & Quinn, K. M. (2010). An introduction to the augmented inverse propensity weighted estimator. Political Analysis, 18(1), 36–56. https://doi.org/10.1093/pan/mpp036
Haggblade, S., Minten, B., Pray, C., Reardon, T., & Zilberman, D. (2017a). The herbicide revolution in developing countries: Patterns, causes, and implications. European Journal of Development Research, 29(3), 533–559. https://doi.org/10.1057/s41287-017-0090-7
Haggblade, S., Smale, M., Kergna, A., Theriault, V., & Assima, A. (2017b). Causes and consequences of increasing herbicide use in Mali. European Journal of Development Research, 29(3), 648–674. https://doi.org/10.1057/s41287-017-0087-2
Haggblade, S., Diarra, A., Jiang, W., Assima, A., Keita, N., Traore, A., & Traore, M. (2019a). Fraudulent pesticides in West Africa: A quality assessment of glyphosate products in Mali. International Journal of Pest Management, 67(1), 32–45. https://doi.org/10.1080/09670874.2019.1668076
Haggblade, S., Keita, N., Traoré, A., Traoré, P., Diarra, A., & Thériault, V. (2019b). A market survey of fraudulent pesticides sold in Mali. Food Security Innovation Lab Research Paper 157. Michigan State University.
Haggblade, S., Diarra, A., & Traoré, A. (2021). Regulating agricultural intensification: Lessons from West Africa’s rapidly growing pesticide markets. Development Policy Review, 40(1), e12545. https://doi.org/10.1111/dpr.12545
Huang, J., Wang, S., & Xiao, Z. (2017). Rising herbicide use and its driving forces in China. European Journal of Development Research, 29(3), 614–627. https://doi.org/10.1057/s41287-017-0081-8
Imbens, G. W. (2000). The role of the propensity score in estimating dose-response functions. Biometrika, 87(3), 706–710. https://doi.org/10.1093/biomet/87.3.706
Imbens, G. W., & Wooldridge, J. M. (2009). Recent developments in the econometrics of program evaluation. Journal of Economic Literature, 47(1), 5–86. https://doi.org/10.1257/jel.47.1.5
INSTAT, 2016. Annuaires statistiques du Mali.
Karamba, R. W., & Winters, P. C. (2015). Gender and agricultural productivity: Implications of the farm input subsidy program in Malawi. Agricultural Economics, 46(3), 357–374. https://doi.org/10.1111/agec.12169
Kelly, V., Kiakite, L., & Teme, B. (2015). Sorghum Productivity in Mali: Past, Present and Future. MSU International Development Working Paper, 138. https://doi.org/10.22004/AG.ECON.207024
Lichtenberg, E., & Zilberman, D. (1986). The econometrics of damage control: Why specification matters. American Journal of Agricultural Economics, 68(2), 261–273. https://doi.org/10.2307/1241427
MIRPlus. (2012). Evaluation de la qualité des pesticides commercialisés dans huit pays de l’espace CEDEAO. ECOWAS and UEMOA.
Palacios-Lopez, A., Christiaensen, L., & Kilic, T. (2017). How much of the labor in African agriculture is provided by women? Food Policy, 67, 52–63.
Rosenbaum, P. R. (2002). Covariance adjustment in randomized experiments and observational studies. Statistical Science, 17(3), 286–327. https://doi.org/10.1214/ss/1042727942
Rosenbaum, P. R. (2005). Heterogeneity and causality: Unit heterogeneity and design sensitivity in observational studies. The American Statistician, 59(2), 147–152.
Sheahan, M., & Barrett, C. B. (2017). Ten striking facts about agricultural input use in Sub-Saharan Africa. Food Policy, 67, 12–25. https://doi.org/10.1016/j.foodpol.2016.09.010
Sheahan, M., Barrett, C. B., & Goldvale, C. (2017). Human health and pesticide use in Sub-Saharan Africa. Agricultural Economics, 48(S1), 27–41. https://doi.org/10.1111/agec.12384
Tamru, S., Minten, B., Alemu, D., & Bachewe, F. (2017). The rapid expansion of herbicide use in smallholder agriculture in Ethiopia: Patterns, drivers, and implications. European Journal of Development Research, 29(3), 628–647. https://doi.org/10.1057/s41287-017-0076-5
Theriault, V., Smale, M., & Assima, A. (2018). The Malian fertiliser value chain post-subsidy: An analysis of its structure and performance. Development in Practice, 28(2), 242–256. https://doi.org/10.1080/09614524.2018.1421145
Traoré, A. S., Dimithe, G., & Toe, A. M. (2011). perspectives des communautés économiques régionales en matière de gestion des pesticides. Gestion Des En Afrique de l’Ouest, (8), 14–19.
Wooldridge, J. M. (2010). Econometric Analysis of Cross Section and Panel Data (2nd ed.). The MIT Press.
Yao, B. O. (2020). Industry efforts to combat fraudulent pesticides in West Africa. Africa Agriculture Status Report 2020, Box 5.2. Alliance for a Green Revolution in Africa.