13 Biodiversity as a Cornerstone of Agrosystems’ Sustainability in West Africa
Highlights box
- Agrobiodiversity is an asset for resilience and sustainability at different scales (species and intra-specific).
- Crop wild relatives and landraces extend the gene pool, providing a functional reservoir of genetic diversity.
- Approaches and methodologies in population genetics can help identify interesting diversity.
- Tapping genetic diversity toward the creation of large populations for breeding schemes is essential in breeding strategies.
- Agrobiodiversity management and use are central under erratic environments.
Abstract
Agrobiodiversity, encompassing crop diversity and the biodiversity that supports agricultural system functioning, plays a major role in agriculture in the context of climate change. This is all the more true in regions like West Africa, where population growth is strong and economic resources are limited. Global models predict average yield loss for major crops of 11% in West Africa by the 2050s. Moreover, climate niches of African crops are expected to shift significantly, reinforcing the need to adapt agricultural diversity and practices. A better use of agrobiodiversity, relying on improved understanding and knowledge of its characteristics, would support greater adaptation and resilience of African agrosystems and food security. Agrobiodiversity, from gene to landscape, is key to achieving food security in the face of the erratic effects of climate change. This chapter highlights novel strategies for agrobiodiversity management and use, while building sustainable and resilient agrosystems for the future of West Africa.
Keywords: Agrobiodiversity, agrosystems, crop wild relatives, resilience, climate change, food security
Introduction
Agriculture in West Africa, which relies primarily on rainfall, is highly vulnerable to climatic events that are particularly extreme and erratic under the influence of the African monsoon. The Sahel and West Africa are considered today as “climate change hotspots” (Intergovernmental Panel on Climate Change, p 1209, 2014). Data indicate long-term changes in the seasonality of rainfall (Nicholson et al., 2018) and increased variability in precipitation (Cornforth et al., 2019). Deep changes are expected in climate suitability, leading to geographic or temporal redistribution of cultivated species (Egbebiyi et al., 2020; Pironon et al., 2019).
Considering the high spatiotemporal variability of West Africa, it is essential to focus not only on agrosystems’ productivity but also on agrosystems’ resilience and sustainability. The concept of resilience, embedding ecological and social-ecological definitions, refers to the capacity of agrosystems to buffer, resist, adapt, or transform to continue feeding the population (Berkes, 2008; Holling, 1973; Walker et al., 2004).
Decades of research in empirical and theoretical ecology have shown that biodiversity, in a broad sense, drives systems resilience. Regarding agrosystems, biodiversity is key to establishing resilient and sustainable food production and improving livelihood (Félix et al., 2018; Lin, 2011). Crop intraspecific diversification or change in the crop portfolio is often the first adjustment chosen by smallholder farmers facing climate change impacts (Fahad & Wang, 2018; Kassie et al., 2013; Shinbrot et al., 2019). However, the nature of crop diversity changes is essential: In a recent review, Labeyrie et al. (2021) showed that farmers’ decisions in response to ongoing climatic events may not be adapted to long-term climate predictions. More research is needed to make the best possible use of biodiversity in agrosystems.
Until now, the valorization of diversity was based on a vision in which the environment was relatively controlled and stable, which made it possible to respond to each constraint by optimizing a few genes within varieties for a single objective. The plurality of values (socio-agro-ecological) and the plurality of environments due to their uncertainties, force researchers and farmers to think of biodiversity as an integrated response of agrosystems and not as a reservoir of solutions. To do this, it is important to consider diversity not as a static element of ecosystems but to understand its dynamics from genes to species, functions and ecosystems, and spatial and temporal scales.
This chapter explores why and how biodiversity in agrosystems (i.e., agrobiodiversity) can participate in resilience and sustainability in West Africa. First, researchers present the role of agrobiodiversity at the agrosystems’ scale and explain how it can help improve yield and stability. Second, researchers underline the importance of understanding the dynamics of diversity at its finest scale to make a better use of agrobiodiversity to face environmental constraints. Finally, researchers highlight that agrobiodiversity management and use is central to building sustainable and resilient agrosystems for the future of West Africa.
The Role of Agrobiodiversity in Agrosystems
1. How Does It Work?
Agrobiodiversity includes domesticated plants and animals, wild fauna, flora, and microbiota, as well as people’s knowledge that supports food production and agriculture (Qualset et al., 1995). It is a pillar of agroecology, ecological engineering, or “nature-based solution,” frameworks that all seek to promote and pilot ecological processes to produce food in a sustainable and resilient way.
The impact of biodiversity on ecosystem services was first studied in natural ecosystems (Gross et al., 2014; Isbell et al., 2015, Tilman & Downing, 1994). Different mechanisms have been identified and have been proven to function in agroecosystems: the insurance effect, the selection effect, and the complementary effect. The insurance effect principle states that in a diversified system, one species—or variety at the species level—may fail, but multiple failures are less likely (Yachi & Loreau, 1999). Different plants exhibit different responses to temperature, precipitation, pests, and diseases. Fluctuations in yield among plants can compensate for each other and stabilize the total biomass production through time. The selection effect principle outlines that the more species or varieties there are in an ecosystem, the greater the chance that the best performing crop will be included. Because one species will find the environmental conditions to be favorable, total biomass production stays stable from year to year. However, an important condition for the selection effect to happen is that the best-performing species or variety in the given year must be dominant. The complementary effect entails direct ecological interactions between plants. Functional differences between species (Hector, 1998; Loreau & Hector, 2001) can lead to reduced interspecific competition, facilitative interactions, and exploitation of different resources (i.e., soil nutrients). Plant height differences, for example, can buffer the impact of extreme climate events (Philpott et al., 2008) or create microclimates that can help control diseases and pests (Zhu et al., 2000).
2. Agrobiodiversity to Improve Yields
Increasing agrobiodiversity through the addition of crops varieties, species, or functional groups (e.g., cereals versus legumes) can be achieved at multiple spatial and temporal scales. Within the same fields, practices include rotations of crops within 1 year (with no temporal overlap) or year after year; inter cropping (i.e., cultivation of more than one crop species); and cultivar mixtures (i.e., cultivation of more than one cultivar of the same species; Smith & McSorley, 2000). Agrobiodiversity also entails management practices promoting noncrop species—including habitat within or around fields (e.g., hedgerows)—or soil biodiversity (i.e., inoculation of beneficial organisms, organic amendments, etc.).
Recently, research on the benefits of diversification practices bloomed worldwide (Beillouin et al., 2019), showing that promoting agrobiodiversity can create multiple benefits simultaneously. In one of the largest-scale field trials across Malawi. Snapp et al. (2010) showed that rotations involving maize and long-lived legumes, at moderate levels of nitrogen fertilizer, had similar yet more stable yields, compared with monoculture maize. In addition, these rotations provided substantial nutritional benefits (i.e., increase in protein yield), greater fertilizer-use efficiency (e.g., >100% more grain yield with the same amount of nitrogen input), and acceptability to farmers. Himmelstein et al. (2017) found that intercropping practices across Africa benefitted both yields (e.g., increase of 23% on average) and growers’ incomes compared with monocropping systems. However, authors noted that the management of leguminous intercrop systems could still be optimized to reach their full potential. Until now, cultivar mixtures have been used mainly for disease and weed management (Beillouin et al., 2019). However, meta-analyses have demonstrated that mixtures significantly improved yield compared with monoculture (Reiss & Drinkwater, 2018; Smithson & Lenné 1996). They also found that yield benefits were much greater in tropical areas and where plants grow in soil stressful conditions (i.e., low soil organic content and in acidic soils).
3. Agrobiodiversity to Face Climate Variability
The potential for agrobiodiversity to buffer agricultural impacts of climate shocks is less researched. Among the few empirical studies assessing yield stability, results supported the positive effect of the diversity of crop species (Gaudin et al., 2015; Smith et al., 2008) on the temporal stability of yield and income (Auffhammer & Carleton, 2018; Di Falco & Chavas, 2008). To date, only two meta-analyses synthesized results on the stabilizing effect of diversity. Research showed that yield stability of cereal-grain legume intercropping systems in tropical regions is greater than both cereal and legume cultivated as sole crops (Raseduzzaman & Jensen, 2017; Reiss & Drinkwater, 2018) Facilitation mechanisms leading to greater productivity and stability within fields may be stronger under more stressful conditions (Maestre et al., 2009; Prieto et al., 2015).
Renard & Tilman (2019) investigated whether crops’ diversity increases year-to-year stability of crops production in the context of climate change at the national scale over 5 decades (19612011) and 91 nations. Results showed that greater crop species and functional group diversity increased the stability of food supply and resilience to interannual variability of precipitation at the national level. Although agrobiodiversity can contribute to sustainable agriculture in diverse ways, how to pilot it for greater resilience deserves more research, notably in West Africa with its strong climate constraints.
From Landscapes to Genes
1. A New Perspective on Selection Schemes
At the scale of varieties, the same principles may apply. The insurance strategy guides farmers’ choices in regions with high variability: They prefer to have varieties that produce a lower yield under a multitude of conditions than varieties that perform extremely well only under very specific conditions (Van Oosterom et al., 1996; Weltzien et al., 1998). At the agrosystems level, more research is needed to identify combinations of species and traits that can optimize yield stability through an insurance or a complementary effect. Achieving production stability under all climatic conditions could also rely on the valorization of intraspecific diversity. As Haussmann et al. (2012) stated, the scale of varieties should consider two levels: individual buffering and population buffering. Individual buffering is the variance of the individual’s phenotype, which is expressed as a function of environmental conditions. This buffering may be accentuated by the presence of different alleles for the locus of interest. Population buffering is the variance across all individuals in the population and therefore of the intra-population diversity (see Figure 1).
Figure 1
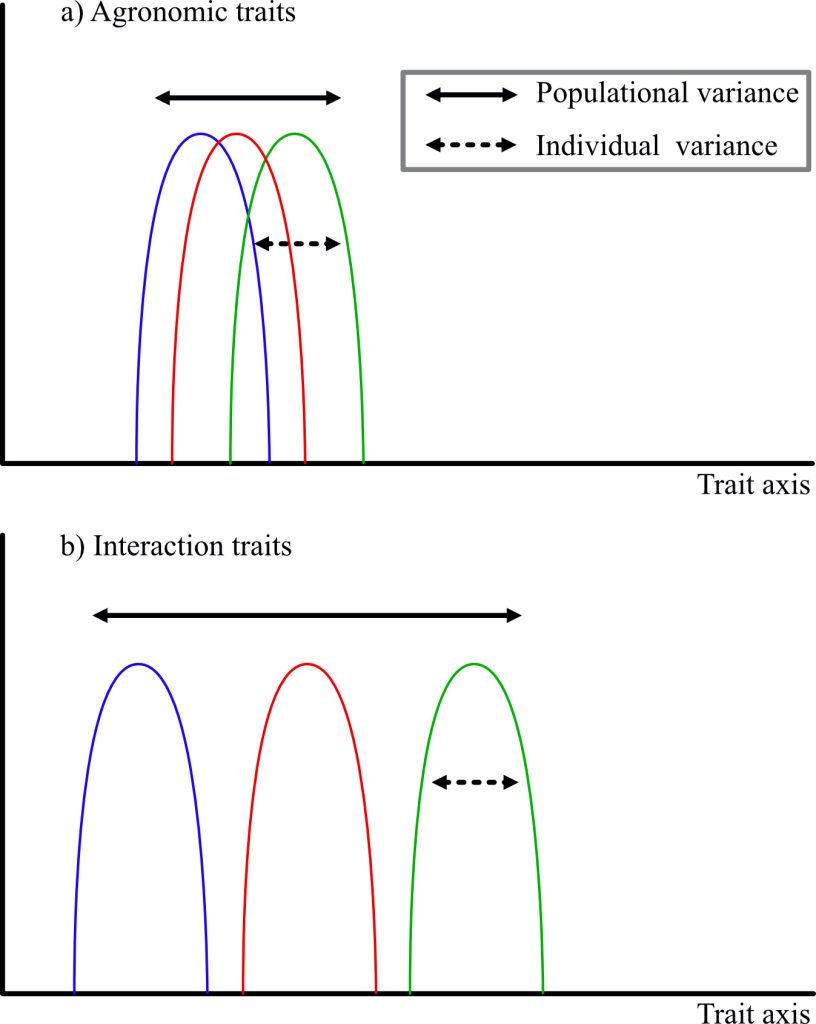
The idea of highlighting variances within individuals and populations to improve agrosystems’ performance is recognized in ecology for natural ecosystems (Violle et al., 2012) and has been further developed for agrosystems by rethinking selection schemes (Litrico & Violle, 2015). The authors break functional traits into two types: traits of agronomic interest, often related to productivity (i.e., grain number and weight) and interaction traits (i.e., rooting depth, vegetative architecture, and phenology). Thus, Litrico and Violle (2015) proposed that the variance be considered according to the two types of traits. Agronomic traits should have a very small variance of the trait at the individual level and the population level, so that all individuals tend toward the same optimum. Conversely, interaction traits should minimize the individual variance and maximize the population variance, so that the population covers a wide range of conditions. Thus, it is rather a question of thinking of an “ideomix” than an ideotype.
Variance, at the individual and population scales, is governed by genes involved in the trait under consideration. Flowering earliness, for instance, is highly regarded as adaptive to drought. It allows escape from the midseason rainfall breaks which are characteristic of Sahelian Africa. Many studies seek to identify the major genes involved in earliness. Fewer studies focus on the variance within the cycle, but a variance of a few weeks may allow some plants to survive while others do not. In pearl millet, PhyC (PHYTOCRHOME C) has been identified as a major gene involved in precocity (Saïdou et al., 2014; Vigouroux et al., 2011). Nevertheless, a gap of more than 15 days may exist between the first plant to flower and the last. Such gaps are even observed within full-sib families. Faye et al. (2022) confirmed the importance of the PgPhyC gene with the T allele of the SNP (Single Nucleotide Polymorphism) chr2_11155563 almost fixed in early varieties and the alternative allele in late varieties. In addition, they identified a second gene, FRS12 (FAR1-RELATED SEQUENCE 12) for which four SNPs were identified, some with more subtle frequency differences between the two varieties. In addition to these two genes, which seem to have major effects, a number of other genes have also been detected and present a great interest in breeding to target individual or population buffering in breeding programs.
2. Sources of Interesting Genetic Diversity
The described example on flowering earliness highlights the necessity to identify reservoirs of genetic diversity, the importance of understanding genes’ involvement in traits, interactions, and the relationship between genes and environment. This study presents functional reservoirs of diversity and describes why this diversity could be interesting in an integrated way of valorization. Neglected crops will not be included here but rather detailed in a companion paper.
2.1 Wild Relatives as a Source of “Ready-to-Use” Genes for Crop Adaptation
Since the first domestication of wild plants some 12,000 years ago, cultivated crops have shared a complex and long history with humans, the environment, and their wild relatives. Despite the reduction of habitats because of evolving environmental conditions and human spread, Crop Wild Relatives (CWR) remain interesting reservoirs of diversity and may conceal useful alleles in view of crop improvement and adaptation (Guarino & Lobell, 2011). Wild relatives still exchanging genes with the cultivated pool refuels the cultivated diversity. In yam, for example, constant inclusion of wild individuals in the cultivated pool in Benin is a factor of enrichment of the cultivated diversity (Scarcelli et al., 2006). A genomic study on pearl millet provided evidence that wild-to-crop gene flow increased cultivated genetic diversity leading to adaptive introgression (Burgarella et al., 2018). Such cases may be an effective strategy for informing new breeding directions and for uncovering ready-to-use wild diversity relevant for crop adaptation to current environmental changes (Burgarella et al., 2019).
Populations of African CWR are also found outside the geographical range of their cultivated counterparts, and therefore they experience different environmental conditions. For instance, the wild rice Oryza barthii shows a much wider distribution than the domesticated species it gave birth to, the cultivated African rice O. glaberrima (Lu et al., 2010). The wild ancestor of pearl millet is found in more extreme northern latitudes than the cultivated populations (i.e., in drier environments, see Figure 2). In these populations, Berthouly-Salazar et al. (2016) identified genetic variation linked to abiotic and biotic stress responses, and Ousseini et al. (2017) revealed a gene implicated in the number of flowers, both covarying with the gradient of rainfall. This pristine natural variation linked to local adaptation can be an asset for crop improvement.
Figure 2
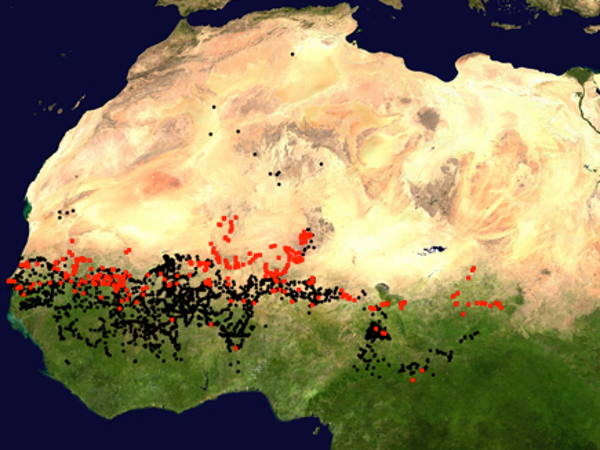
2.2 Landraces, Genetic Load, Local Adaptation, and Cryptic Variation
Despite the well-known bottleneck effect that makes the wild compartment of landraces a complementary source of diversity, a lesser-known effect of the domestication process is the existence of a deleterious load within cultivated populations (Moyers et al., 2018). This possible “cost of domestication” refers to the increase in frequency of deleterious alleles segregating in the domesticated species as compared to the wild compartment. The existence of this genetic load implies an extended linkage and reduced genetic diversity. Intensive breeding programs would also strongly increase the number of deleterious mutations in domesticated species (Frantz et al., 2020). The study of these deleterious alleles, and of their frequency in different populations, may help orientate breeding strategies and diversity valorization, as their existence may limit the efficacy of selection and impact breeding efficacy.
From their domestication, each crop settled in new locations and colonized new environments through farmers or seed exchanges. These landraces now significantly contribute to the important genetic resources at the disposal of farmers and breeders. Two specific kinds of genetic diversity (i.e., allelic variations) may be of particular interest for crop adaptation and sustainable use in breeding while ensuring diversity conservation.
First, the variability of environmental conditions across a species range drives differential adaptation of populations, resulting in local adaptation. Local adaptation is the difference in relative fitness between a native genotype in a given environment and a nonnative genotype in the same environment (Kawecki & Ebert, 2004). A meta-analysis of 74 studies revealed that local adaptation is common among species and that a local population has on average 45% greater fitness than a foreign population (Hereford, 2009). This statement might not stand when population environments are drastically different or when selective factors are different at opposite ends of the gradient (Hereford, 2009; Popovic & Lowry, 2020). Local adaptations are critical for species survival in diversified and variable environments and thus may enable crop populations, if incorporated in their genetic background, to withstand impacts of climate changes (Razgour et al., 2019; Vargas et al., 2017).
Cryptic variations are a second source that can be just as important in coping with environmental changes, especially when environmental changes are highly contrasted and rapid (Bitter et al., 2019). Cryptic genetic variation has been reported in wild progenitors of crop species and landraces (Lauter & Doebley, 2002; Von Wettberg et al., 2018). Cryptic genetic variation is defined as standing genetic variation that does not contribute to the normal range of phenotypes observed in a population but that can bring forth phenotypic variation after environmental change (Gibson & Dworkin, 2004). Cryptic variation allows large phenotypic shifts outside the normal boundaries of phenotypic variation of a population, (i.e., when a variation in environmental conditions substantially changes the optimal phenotype). These shifts require important genetic stepping-stones that would be possible to reach only if cryptic variation precedes the change in environmental conditions. (Zheng et al., 2019). Given the current pace of environmental changes, exploring and conserving cryptic diversity should be of primary focus.
3. Population Genomics Can Identify Interesting Diversity: Approaches and Methodologies
The potential of this available diversity to improve agriculture in the face of climate change is huge. However, its use is far from being obvious to valorize. Population genetics is the study of allele frequency changes in a group of individuals exchanging genes (i.e., a population). Its concepts and tools are used to study microevolution, population dynamics, and conservation genetics. While broadly applied in natural conditions, population genetics approaches are also relevant in agriculture. When accessing the genetic composition of a population, several parameters can be studied: genotype frequencies, allelic frequencies, gene flows, heritability, genetic correlations, heterozygosity, and other indicators that allow an understanding of the genetic dynamics of the population under study. Using such approaches may help identify the historical effects of evolutionary forces that have shaped current diversity and reveal interesting alleles directly related to the environmental conditions to which they respond. This knowledge and understanding are essential to make an integrated use of agrobiodiversity and to ensure that it is a factor of resilience in agrosystems.
3.1 Detecting Footprints of Selection
The identification of selection, phenotype-genotype links, and candidate genes is possible through the study of allelic frequencies at the whole genome scale. In this paragraph, a synthetic and theoretical way the genomic footprints left by selection in the case of a strong and recent selection by an environmental pressure is presented. The cases of polygenic selection or soft selection are more complex and harder to detect, although surely more frequent and often linked to local adaptation and rapid evolution (Hermisson & Pennings, 2017).
In case an ideal unique population at equilibrium and a recent mutation under strong positive selection is considered, the haplotype(s) carrying a beneficial mutation will increase rapidly in frequency in the population. This selection of a small number of haplotypes will lead to a reduction in diversity around the adaptive allele because polymorphisms physically linked to it will be swept along (referred to as hitchhiking effect). This binding strength will depend on the rate of recombination and the time it takes for it to act. In other words, the lower the recombination rate, the greater the size of the haplotype will be, with a loss of diversity that will expand far away from the causative allele. Similarly, the older the selection pressure is and the more time the recombination has had to break the linkage, the smaller the region affected by the loss of diversity. Because it is this difference in diversity relative to the rest of the genome that can be used to identify the presence of adaptive alleles, it is easy to understand that it will be easier to identify adaptive alleles that have been selected recently or in regions/genomes with low recombination rates.
The loss of diversity around the adaptive allele can be measured either by classical diversity tests, such as Tajima’s D, and across the spectrum of allelic frequencies, also called site frequencies spectrum (SFS). In case of neutrality, this spectrum follows a distribution of the form y = 1/x. In case of selection, the intermediate classes are very little represented or even absent, and the extreme frequencies (i.e., very rare or very frequent alleles), increase in frequency. A third pattern that appears around the adaptive allele, in addition to the reduction of diversity and the SFS change, concerns the linkage disequilibrium (LD). Within a selected haplotype, the LD is high among polymorphisms located on the same side of the adaptive allele but low between SNPs located on either side of the allele. The w-statistic has been proposed to measure this pattern.
Analyses can be performed at the metapopulation level by comparing populations. If the adaptive allele is selected in a specific population, the difference in frequencies of the adaptive allele and those physically linked to it compared to their frequencies in the other populations will be very strong. This phenomenon creates a pattern along the genome where genomic zones involved in adaptation will exhibit more differentiation between populations than the rest of the genome. This differentiation is often measured by the fixation index (FST parameter), estimated from allelic frequencies. The study of FST values, along the chromosomes between two populations, allows the identification of particularly high values of FST, which highlight candidate regions of the genome for selection, including genes involved in adaptation in one of the compared populations.
Approaches based on diversity indices, SFS or LD, are highly dependent on the number and density of SNPs identified. Consequently, these methods are adapted to Whole Genome or capture sequencing technologies, allowing regions spanning a minimum of several dozens to several hundreds of kilobases. The GBS (Elshire et al., 2011), RADSeq (Hohenlohe et al., 2010), or small area capture approaches (Mariac et al., 2018) are more adapted for approaches based on population differentiation. When these types of methods based on FST/genetic differentiation are targeted, the choice of populations (i.e., spatial sampling protocol) is crucial (De Mita et al., 2013). Table 1 presents a synthesis of the different methods to detect selection and associated software.
Sequencing methodology leads to |
Number of populations needed |
Types of footprint |
Softwares |
References |
genomic regions > 50,000 kb |
1 |
Diversity indices |
ANGSD |
Korneliussen et al., 2014 |
( Tajima’s D) |
||||
SFS |
SweepFinder, SweeD |
Nielsen et al., 2005 ; Pavlidis et al., 2013 |
||
LD |
OmegaPlus (2012) |
Alachiotis et al., 2012 |
||
genomic regions < 1000 kb |
>2 |
Genetic differentiation |
PCAdapt, BayeScan |
Luu et al., 2017 ; Foll & Gaggiotti, 2008 |
3.2 Association Methods
Genomic scans looking for adaptive alleles can be complemented by approaches revealing significant correlations between allelic frequencies and phenotypic traits (genotype-phenotype association [GPA]) or environmental variables (genotype-environment association [GEA]). These methods are tailored to help understand the genetic basis of phenotypic variation (GPA) or adaptation to the environment (GEA). The association between phenotypic/environment and genomic variability can be examined through Genome Wide Association Studies (GWAS) and helps decipher metabolic pathways for a better understanding of functional processes. These methods are necessarily sensitive to the number of points to estimate the relationship (>100) and the kinship within the sampling. A review of tests and study of their limitations for GEA and GPA can be found in Rellstab et al. (2015) and Tam et al. (2019), respectively.
Studying adaptation to drought or other stresses on classical inbred lines or more complex ones such as RILS or NAMs is a good start but it is not enough. The diversity represented in these synthetic panels is reduced, and creating these resources is time consuming and costly—they generally require several generations of inbreeding of a rather large number of individuals. Whenever possible, CWRs and landraces should be included in adaptation studies because they are functional reservoirs of a genetic diversity that are essential for the resilience of varieties. Then, if researchers want to extract information from them, they must use population genomics methods that are adapted to heterogeneous populations and that allow the detection of local adaptations (Hoban et al., 2016).
3.3 Correlation Between Genetic Diversity and Environmental Variability
Another emerging set of methods seeks to infer the current populations’ vulnerabilities to future climatic conditions, including increased drought events. In contrast with well-known niche-modelling approaches that use the extent of species distribution to draw suitable areas at the species level, these approaches use the available genetic diversity to give a finer-scale view of the effect of future climates on local populations (i.e., landraces). These approaches largely rely on newly developed Machine Learning approaches to link the variations of genetic diversity and the climate that should have modelled it. If these approaches were initially developed and applied to natural populations of plants or animals (Bay et al., 2018; Fitzpatrick & Keller, 2015), there is a growing interest in applying them to cultivated plants (Capblancq et al., 2020; Rhoné et al., 2020). A detailed example is reported in Box 1.
Altogether, population genetics theory and its application to the study of agrobiodiversity’s history and contemporary evolution in the face of current environmental constraints is a powerful tool to better understand populations’ dynamics and identify how to make a reasonable and integrated use of their genetic diversity.
Box 1: A study of genomic vulnerability
A recent study has focused on estimating the local adaptation of traditional landraces of pearl millet to environmental variations in West Africa (Rhoné et al, 2020). Using a fine-scale climatic dataset and climatic variables calculated to match the growing season of the crop (Sultan et al, 2019), researchers have investigated how genetic diversity has been shaped by variation in climate conditions. Using a machine learning algorithm that monitors shifts in allele frequencies toward environmental gradients, this first step of the study determined that the impact of climate on genetic structure is important. Using the relationships established between climate variables and allelic variations, a second step of the study consisted in predicting the allelic composition that will be required to fit future
climate conditions estimated under a climate change model. By calculating a genetic distance between the current allelic composition of a landrace and the future one at the same location under climate change, researchers computed a so-called genomic vulnerability (GV, aka genetic offset). This GV informs on the magnitude of evolution needed to cope with future climate for a given landrace. For pearl millet, researchers observed a particular pattern of GV that was subsequently linked to a variation in flowering time using a GWAS approach.
To validate that the GV can efficiently predict a biological impact of climate change, researchers used a common garden experiment. Using a field in Niger as a common environment for several landraces coming from all West Africa, researchers computed for these test landraces a GV between their original location and the common garden. They evaluated performance of the landraces by monitoring the growth and several key phenotypic traits linked to the yield. They then tested if a greater GV ended up with a lower yield. The obtained negative correlation between GV and yield estimators was highly significant, with a Pearson’s r ranging from -0.31 to -0.42 depending on the considered parameter, validating the predictive power of the GV approach for the first time in a crop plant.
A last work performed under this study was to evaluate if material exchange throughout West Africa could locally reduce GV, using a newly developed measure of the remaining GV after a migration event. This investigation led to the outcome that within-country migrations would not be efficient in significantly reducing the GV but larger, regional-scale migrations should help reduce the risk of yield loss due to adverse climatic conditions.
Management and Uses of Agrobiodiversity
1. Conservation Strategies
The importance of agrobiodiversity, defended in this chapter, brings another perspective on the conservation of genetic resources. While it is recognized that in situ conservation—in addition to ex-situ collections of diversity—is crucial to maintain evolutionary forces under work in populations, the question of the genetic erosion arises in debates on climate change. The pace imposed by this environmental perturbation begs the question: Can populations adapt quickly enough while not losing all important genetic variation if the selection pressure is too strong? In the face of climatic change, it becomes important to maintain evolutionary forces at work and to ensure that these evolutionary forces will be able to maintain a dynamic state that continues creating diversity. Evolution models must therefore be developed to thoroughly understand how diversity will behave in the face of climate change and to make recommendations on practices that would promote a dynamic in favor of maintaining diversity, and against undesired genetic erosion. In that, the role of so-called de-facto conservation, through agricultural practices oriented toward more inclusion of agrobiodiversity is primordial, local actors are guarantors of the genetic richness present on their territory.
2. Genetic Resources Access
Despite the key role that agrobiodiversity is expected to play to build a sustainable agriculture resilient to climate change, a paradoxical situation prevails today. On the one hand, new genomics, phenotyping, and information technologies promote a more rapid development of knowledge and an increased and accelerated exploitation of genetic resources in the public and private sectors. On the other hand, international regulations tend to make access to and exchange of genetic resources more complex, although underpinned by the laudable goal of equity. These instruments (the International Treaty on Plant Genetic Resources [ITPGRFA] and the Nagoya Protocol [NP] of the Convention on Biological Diversity [CBD]) aim to guarantee access to genetic resources and the fair and equitable sharing of the benefits stemming from their use. Better uses of this diversity will require improved mechanisms for exchanging information and resources rapidly and effectively, considering the diversity in value systems at work among stakeholders who often have different visions and objectives for the exchange of genetic resources (Jankowski et al., 2020).
These challenges will require coordinated transdisciplinary projects across research disciplines (e.g., agronomy, anthropology, sociology, economics, breeding and genetics) involving actors beyond research. Access to this biodiversity must be preserved in itself for the functions and services rendered within agro-ecosystems, which does not prohibit the use of the genetic resources offered (i.e., genetic improvement). It must be done in a sustainable manner and in a logic of common goodness at different scales and for multiple actors. To meet the current challenges of crop adaptation and improvement under dry environment, the authors advocate for a broad understanding of innovation, which requires the involvement of new diversity and new actors in the knowledge production process.
Perspectives
The identification of genes or genomic regions involved in adaptation or in the past evolution of populations significantly enriched the knowledge of the variability existing in populations and its potential usefulness, in particular through the study of the links with the environment. It also appears that a classical view, where one crop improvement need can be addressed by one appropriate allele, does not respond properly to the specific constraints imposed by the local context of West Africa or to the perspective of the ongoing rapidly changing climate. Moreover, such approaches would fail in establishing long-term sustainable and resilient agrosystems. On the contrary, the maintenance of numerous alleles in a diversity of crops seems more likely to ensure agrosystems’ stability at the level of crops populations. Similarly, maintaining a diversity of crop species within and outside fields holds the potential to promote stability of food production and livelihoods.
CWR and landraces are thus potentially valuable sources of alleles of interest, especially in the context of climatic unpredictability and of needed resilient strategies. A classical view regarding biodiversity valorization relies on a triptych conservation-characterization-use in prebreeding. However, this approach is far from simple. The characterization and use of genetic resources requires tremendous investments and precedent knowledge regarding the genetic complexity of adaptive traits. As a result, biodiversity valorization in breeding programs has been recognized as essential for a long time but scarcely led to concrete successful examples, regarding the huge potential offered by the total conserved diversity. In addition, it remains a tit-for-tat strategy that may encounter limits when it comes to adapting populations to important and erratic changes in the environment.
Conclusion
Agrobiodiversity should be an integrated input at several scales—landscapes, species, individual genotypes, and genes—to contribute to more stable food systems in the face of erratic effects of climate and global changes. Allowing a broader and more efficient use of agrobiodiversity, (i.e., the study of the finest level of diversity in its dynamic state, as population genetics and genomics approaches), is essential for a better understanding and knowledge of the characters of interest to significantly improve agrosystems’ functioning. Through their role, breeders and farmers are the guarantors of this agrobiodiversity. They can greatly influence its conservation by integrating the concept of diversity into their strategies. If improvement is still considered a factor of erosion in intensive agricultural systems, an inverted paradigm is possible through an approach based on an objective of stability and resilience of agrosystems.
References
Auffhammer, M., & Carleton, T. A. (2018). Regional crop diversity and weather shocks in India. Asian Development Review, 35(2), 113-130.
Bay, R. A., Harrigan, R. J., Le Underwood, V., Lisle Gibbs, H., Smith, T. B., & Ruegg, K. (2018). 359(6371), 83-86.
Beillouin, D., Ben-Ari, T., & Makowski, D. (2019). Evidence map of crop diversification strategies at the global scale. Environmental Research Letters, 14(12), Article 123001.
Berthouly‐Salazar, C., Thuillet, A.-C., Rhoné, B., Mariac, C., Ousseini, I. S., Couderc, M., Tenaillon, M. I., & Vigouroux, Y. (2016). Genome scan reveals selection acting on genes linked to stress response in wild pearl millet. Molecular ecology, 25(21), 5500-5512.
Berkes, F., Colding, J., & Folke, C. (Eds.). (2008). Navigating social-ecological systems: building resilience for complexity and change. Cambridge University Press.
Bitter, M. C., Kapsenberg, L., Gattuso, J.-P., & Pfister, C. A. (2019). Standing genetic variation fuels rapid adaptation to ocean acidification. Nature communications, 10(1), Article 5821.
Burgarella, C., Barnaud, A., Kane, N. A., Jankowski, F., Scarcelli, N., Billot, C., Vigouroux, Y., & Berthouly-Salazar, C. (2019). Adaptive introgression: an untapped evolutionary mechanism for crop adaptation. Frontiers in Plant Science, 10, 4.
Burgarella, C., Cubry, P., Kane, N. A., Varshney, R. K., Mariac, C., Liu, X., Shi, C., Thudi, M., Couderc, M., Xu, X., Chitkineni, A., Scarcelli, N., Barnaud, A., Rhoné, B., Dupuy, C., François, O., Berthouly-Salazar, C., & Vigouroux, Y. (2018). A western Sahara centre of domestication inferred from pearl millet genomes. Nature ecology & evolution, 2(9), 1377-1380.
Capblancq, T., Fitzpatrick, M. C., Bay, R. A., Exposito-Alonso, M., & Keller, S. R. (2020). Genomic prediction of (mal)adaptation across current and future climatic landscapes. Annual Review of Ecology, Evolution, and Systematics, 51(1), 245-269.
Cornforth, R., Parker, D. J., Diop-Kane, M., Fink, A. H., Lafore, J. P., Laing, A., Afiesimama, E., Caughey, J., Diongue-Niang, A., Hamza, I., Harou, A., Kassimou, A., Lamb., P., Lampety, B., Mumba, Z., Nnodu., I., Omotosho, J., Palmer., S., Parrish, P., … Tompkins, A. (2019). The first Forecasters’ Handbook for West Africa. Bulletin of the American Meteorological Society, 100(11), 2343-2351.
De Mita, S., Thuillet, A.-C., Gay, L., Ahmadi, N., Manel, S., Ronfort, J., & Vigouroux, Y. (2013). Detecting selection along environmental gradients: analysis of eight methods and their effectiveness for outbreeding and selfing populations. Molecular ecology, 22(5), 1383-1399.
Di Falco, S., & Chavas, J.-P. (2008). Rainfall shocks, resilience, and the effects of crop biodiversity on agroecosystem productivity. Land Economics, 84(1), 83-96.
Egbebiyi, T. S., Crespo, O., Lennard, C., Zaroug, M., Nikulin, G., Harris, I., Price, J., Forstenhäusler, N., & Warren, R. (2020). Investigating the potential impact of 1.5, 2 and 3 °C global warming levels on crop suitability and planting season over West Africa. PeerJ, 8, Article e8851.
Elshire, R. J., Glaubitz, J. C., Sun, Q., Poland, J. A., Kawamoto, K., Buckler, E. S., & Mitchell, S. E. (2011). A robust, simple genotyping-by-sequencing (GBS) approach for high diversity species. PLOS ONE, 6(5), Article e19379.
Fahad, S., & Wang, J. (2018). Farmers’ risk perception, vulnerability, and adaptation to climate change in rural Pakistan. Land Use Policy, 79, 301-309.
Faye, A., Barnaud, A., Kane, N. A., Cubry, P., Mariac, C., Burgarella, C., Rhoné., B., Faye, A., Olodo, K., Cisse, A., Couderc, M., Dequincey, A., Zekraouï, L., Moussa, D., Tidjani, M., Vigouroux, Y., & Berthouly-Salazar, C. (2022). Genomic footprints of selection in early- and late-flowering pearl millet landraces. [Manuscript submitted for publication].
Félix, G. F., Diedhiou, I., Le Garff, M., Timmermann, C., Clermont-Dauphin, C., Cournac, L., Groot, J. C. J., & Tittonell, P. (2018). Use and management of biodiversity by smallholder farmers in semi-arid West Africa. Global Food Security, 18, 76–85.
Fitzpatrick, M. C., & Keller, S. R. (2015). Ecological genomics meets community‐level modelling of biodiversity: mapping the genomic landscape of current and future environmental adaptation. Ecology Letters, 18(1), 1-16.
Frantz, L. A. F., Bradley, D. G., Larson, G., & Orlando, L. (2020). Animal domestication in the era of ancient genomics. Nature Reviews Genetics, 21(8), 449-460.
Gaudin, A. C. M., Tolhurst, T. N., Ker, A. P., Janovicek, K., Tortora, C., Martin, R. C., & Deen, W. (2015). Increasing crop diversity mitigates weather variations and improves yield stability. PLOS ONE, 10(2), Article e0113261.
Gibson, G., & Dworkin, I. (2004). Uncovering cryptic genetic variation. Nature Reviews Genetics, 5(9), 681-690.
Gross, K., Cardinale, B. J., Fox, J. W., Gonzalez, A., Loreau, M., Wayne Polley, H., Reich, P. B., & van Ruijven, J. (2014). Species richness and the temporal stability of biomass production: a new analysis of recent biodiversity experiments. The American Naturalist, 183(1), 1-12.
Guarino, L., & Lobell, D. B. (2011). A walk on the wild side. Nature Climate Change, 1(8), 374-375.
Haussmann, B. I. G., Fred Rattunde, H., Weltzien‐Rattunde, E., Traoré, P. S. C, vom Brocke, K., & Parzies, H. K. (2012). Breeding strategies for adaptation of pearl millet and sorghum to climate variability and change in West Africa. Journal of Agronomy and Crop Science, 198(5), 327-339.
Hector, A. (1998). The effect of diversity on productivity: detecting the role of species complementarity. Oikos, 82(3), 597-599.
Hereford, J. (2009). A quantitative survey of local adaptation and fitness trade-offs. The American Naturalist, 173(5), 579-588.
Hermisson, J., & Pennings, P. S. (2017). Soft sweeps and beyond: understanding the patterns and probabilities of selection footprints under rapid adaptation. Methods in Ecology and Evolution, 8(6), 700-716.
Himmelstein, J., Ares, A., Gallagher, D., & Myers, J. (2017). A meta-analysis of intercropping in Africa: impacts on crop yield, farmer income, and integrated pest management effects. International Journal of Agricultural Sustainability, 15(1), 1-10.
Hoban, S., Kelley, J. L., Lotterhos, K., Antolin, M. F., Bradburd, G., Lowry, D. B., Poss, M. L., Reed, L. K., Storfer, A., & Whitlock, M. C. (2016). Finding the genomic basis of local adaptation: pitfalls, practical solutions, and future directions. The American Naturalist, 188(4), 379-397.
Hohenlohe, P. A., Bassham, S., Etter, P. D., Stiffler, N., Johnson, E. A., & Cresko, W. A. (2010). Population genomics of parallel adaptation in three spine stickleback using sequenced RAD tags. PLOS Genetics, 6(2), Article e1000862.
Holling, C. S. (1973). Resilience and stability of ecological systems. Annual Review of Ecology and Systematics, 4(1973), 1-23.
Intergovernmental Panel on Climate Change. (2014). Climate Change 2014 – impacts, adaptation, and vulnerability: Part B: regional aspects. Working Group II contribution to the IPCC Fifth Assessment Report. Cambridge University Press.
Isbell, F., Craven, D., Connolly, J., Loreau, M., Schmid, B., Beierkuhnlein, C., Martijn Bezemer, T., Bonin, C., Bruelheide, H., de Luca, E., Ebeling, A., Griffin, J. N., Guo, Q., Hautier, Y., Hector, A., Jentsch, A., Kreyling, J., Lanta, V., … & Eisenhauer, N. (2015). Biodiversity increases the resistance of ecosystem productivity to climate extremes. Nature, 526(7574), 574-577.
Jankowski, F., Louafi, S., Kane, N. A., Diol, M., Camara, A. D., Pham, J.-L., Berthouly, C., & Barnaud, A. (2020). From texts to enacting practices: defining fair and equitable research principles for plant genetic resources in West Africa. Agriculture and Human Values, 37(4), 1083-1094.
Kassie, B. T., Hengsdijk, H., Rötter, R. P., Kahiluoto, H., Asseng, S., & van Ittersum, M. K. (2013). Adapting to climate variability and change: experiences from cereal-based farming in the Central Rift and Kobo Valleys, Ethiopia. Journal of Environmental Management, 52(5), 1115-1131.
Kawecki, T. J., & Ebert, D. (2004). Conceptual issues in local adaptation. Ecology Letters, 7(12), 1225-1241.
Labeyrie, V., Renard, D., Aumeeruddy-Thomas, Y., Benyei, P., Caillon, S., Calvet-Mir, L., Carrière, S. M., Demongeot, M., Descamps, E., Braga Junqueira, A., Li, X., Locqueville, J., Mattalia, G., Miñarro, S., Morel, A., Porcuna-Ferrer, A., Schlingmann, A., Vieira da Cunha Avila, J., & Reyes-García, V. (2021). The role of crop diversity in climate change adaptation: insights from local observations to inform decision making in agriculture. Current Opinion in Environmental Sustainability, 51, 15-23.
Lauter, N., & Doebley, J. (2002). Genetic variation for phenotypically invariant traits detected in teosinte: implications for the evolution of novel forms. Genetics, 160(1), 333-342.
Lin, B. B. (2011). Resilience in agriculture through crop diversification: adaptive management for environmental change. BioScience, 61(3), 183-193.
Litrico, I., & Violle, C. (2015). Diversity in plant breeding: a new conceptual framework. Trends in Plant Science, 20(10), 604-613.
Loreau, M., and Hector, A. (2001). Partitioning selection and complementarity in biodiversity experiments. Nature, 412(6842), 72-76. https://doi.org/10.1038/35083573
Lu, B.-R., Jackson, M., & Vaughn, D. (2010). Wild rice taxonomy. Rice Knowledge Bank.
Maestre, F. T., Callaway, R. M., Valladares, F., & Lortie, C. J. (2009). Refining the stress‐gradient hypothesis for competition and facilitation in plant communities. Journal of Ecology, 97(2), 199-205.
Mariac, C., Vigouroux, Y., Duponchelle, F., García-Dávila, C., Núñez, J., Desmarais, E., & Renno, J. F. (2018). Metabarcoding by capture using a single COI probe (MCSP) to identify and quantify fish species in ichthyoplankton swarms. PLOS ONE, 13(9), Article e0202976.
Moyers, B. T., Morrell, P. L., & McKay, J. K. (2018). Genetic costs of domestication and improvement. Journal of Heredity, 109(2), 103-116.
Nicholson, S. E., Funk, C., & Fink, A. H. (2018). Rainfall over the African continent from the 19th through the 21st century. Global and Planetary Change, 165, 114-127.
Ousseini, I. S., Bakasso, Y., Kane, N. A., Couderc, M., Zekraoui, L., Mariac, C., Manicacci, D., Rhoné, B., Barnaud, A., Berthouly-Salazar, C., Assoumane, A., Moussa, D., Moussa, T., & Vigouroux, Y. (2017). Myosin XI is associated with fitness and adaptation to aridity in wild pearl millet. Heredity, 119(2), 88-94.
Philpott, S. M., Lin, B. B., Jha, S., & Brines, S. J. (2008). A multi-scale assessment of hurricane impacts on agricultural landscapes based on land use and topographic features. Agriculture, Ecosystems & Environment, 128(1-2), 12-20.
Pironon, S., Etherington, T. R., Borrell, J. S., Kühn, N., Macias-Fauria, M., Ondo, I., Tovar, C., Wilkin, P., & Willis, K. J. (2019). Potential adaptive strategies for 29 sub-Saharan crops under future climate change. Nature Climate Change, 9(10), 758-763.
Popovic, D., & Lowry, D. B. (2020). Contrasting environmental factors drive local adaptation at opposite ends of an environmental gradient in the yellow monkeyflower (Mimulus guttatus). American Journal of Botany, 107(2), 298-307.
Prieto, I., Violle, C., Barre, P., Durand, J.-L., Ghesquiere, M., & Litrico, I. (2015). Complementary effects of species and genetic diversity on productivity and stability of sown grasslands. Nature Plants, 1(4), 1-5.
Qualset, C., Mcguire, P., & Warburton, M. (1995). In California: ‘Agrobiodiversity’ key to agricultural productivity. California Agriculture, 49(6), 45-49.
Raseduzzaman, M., & Jensen, E. S. (2017). Does intercropping enhance yield stability in arable crop production? A meta-analysis. European Journal of Agronomy, 91, 25-33.
Razgour, O., Forester, B., Taggart, J. B., Bekaert, M., Juste, J., Ibáñez, C., Puechmaille, S., Novella-Fernandez, R., Alberdi, A., & Manel, S. (2019). Considering adaptive genetic variation in climate change vulnerability assessment reduces species range loss projections. Proceedings of the National Academy of Sciences, 116(21), 10,418-10,423.
Reiss, E. R., & Drinkwater, L. E. (2018). Cultivar mixtures: a meta‐analysis of the effect of intraspecific diversity on crop yield. Ecological Applications, 28(1), 62-77.
Rellstab, C., Gugerli, F., Eckert, A. J., Hancock, A. M., & Holderegger, R. (2015). A practical guide to environmental association analysis in landscape genomics. Molecular Ecology, 24(17), 4348-4370.
Renard, D., & Tilman, D. (2019). National food production stabilized by crop diversity. Nature, 571(7764), 257-260.
Rhoné, B., Defrance, D., Berthouly-Salazar, C., Mariac, C., Cubry, P., Couderc, M., Dequincey, A., Assoumanne, A., Ardo Kane, N., Sultan, B., Barnaud, A., & Vigouroux, Y. (2020). Pearl millet genomic vulnerability to climate change in West Africa highlights the need for regional collaboration. Nature Communications, 11(1), 1-9.
Saïdou, A.-A., Clotault, J., Couderc, M., Mariac, C., Devos, K. M., Thuillet, A.-C., Amoukou, I. A., & Vigouroux, Y. (2014). Association mapping, patterns of linkage disequilibrium and selection in the vicinity of the PHYTOCHROME C gene in pearl millet. Theoretical and Applied Genetics, 127(1), 19-32.
Scarcelli, N., Tostain, S., Vigouroux, Y., Agbangla, C., Daïnou, O., & Pham, J.-L. (2006). Farmers’ use of wild relative and sexual reproduction in a vegetatively propagated crop. The case of yam in Benin. Molecular Ecology, 15(9), 2421-2431.
Shinbrot, X. A., Jones, K. W., Rivera-Castañeda, A., López-Báez, W., & Ojima, D. S. (2019). Smallholder farmer adoption of climate-related adaptation strategies: The importance of vulnerability context, livelihood assets, and climate perceptions. Environmental Management, 63(5), 583-595. https://doi.org/10.1007/s00267-019-01152-z
Smith, H. A., & McSorley, R. (2000). Intercropping and pest management: a review of major concepts. American Entomologist, 46(3), 154-161.
Smith, R. G., Gross, K. L., & Robertson, G. P. (2008). Effects of crop diversity on agroecosystem function: crop yield response. Ecosystems, 11(3), 355-366.
Smithson, J. B. & Lenné, J. M. (1996). Varietal mixtures: a viable strategy for sustainable productivity in subsistence agriculture. Annals of Applied Biology, 128(1), 127-158.
Snapp, S. S., Blackie, M. J., Gilbert, R. A., Bezner-Kerr, R., & Kanyama-Phiri, G. Y. (2010). Biodiversity can support a greener revolution in Africa. Proceedings of the National Academy of Sciences, 107(48), 20,840-20,845.
Sultan, B., Defrance, D., & Iizumi, T. (2019). Evidence of crop production losses in West Africa due to historical global warming in two crop models. Scientific Reports, 9(1), 1-15.
Tam, V., Patel, N., Turcotte, M., Bossé, Y., Paré, G., & Meyre, D. (2019). Benefits and limitations of genome-wide association studies. Nature Reviews Genetics, 20(8), 467-484.
Tilman, D., Downing, J. A., & Wedin, D. A. (1994). Does diversity beget stability? Nature, 371, 114. https://doi.org/10.1038/371114a0
Vargas, C. A., Lagos, N. A., Lardies, M. A., Duarte, C., Manríquez, P. H., Aguilera, V. M., Broitman, B., Widdicombe, S., & Dupont, S. (2017). Species-specific responses to ocean acidification should account for local adaptation and adaptive plasticity. Nature Ecology & Evolution, 1(4), 1-7.
Van Oosterom, E. J., Whitaker, M. L., & Weltzien, E. (1996). Integrating genotype by environment analysis, characterization of drought patterns, and village surveys to identify adaptive plant traits for pearl millet. In M. Cooper and G.L. Hammer (Eds.), Plant Adaptation and Crop Improvement (pp. 383-402). CAB International.
Vigouroux, Y., Mariac, C., De Mita, S., Pham, J.-L., Gérard, B., Kapran, I., Sagnard, F., Deu, M., Chantereau, J., Ali, A., Ndjeunga, J., Luong, V., Thuillet, A.-C., Saïdou, A.-A., & Bezançon, G. (2011). Selection for earlier flowering crop associated with climatic variations in the Sahel. PLOS ONE, 6(5), Article e19563.
Violle, C., Enquist, B. J., McGill, B. J., Jiang, L. C. H., Albert, C. H., Hulshof, C., Jung, V., & Messier, J. (2012). The return of the variance: intraspecific variability in community ecology. Trends in Ecology & Evolution, 27(4), 244-252.
von Wettberg, E. J., Chang, P. L., Başdemir, F., Carrasquila-Garcia, N., Korbu, L. B., Moenga, S. M., Bedada, G., Greenlon, A., Moriuchi, K. S., Singh, V., Cordeiro, M. A., Noujdina., N. V., Dinegde, K. N., Shah Sani, S. G. A., Getahun, T., Vance, L., Bergmann, E., Lindsay, D., Mamo, B. E., Warschefsky, E. J., … & Cook, D. R. (2018). Ecology and genomics of an important crop wild relative as a prelude to agricultural innovation. Nature Communications, 9(1), 1-13.
Walker, B., Holling, C. S., Carpenter, S. R., & Kinzig, A. (2004). Resilience, adaptability and transformability in social-ecological systems. Ecology and Society, 9(2).
Weltzien E., Whitaker, M. L., Rattunde, H. F. W., Dhamotharan, M., & Anders, M. M. (1998). Participatory approaches in pearl millet breeding. In J. R Witcombe, D. S. Virk and J. Farrington (Eds.), Seeds of Choice: Making The Most of New Varieties For Small Farmers (pp. 143-170). Oxford and IBH Publishing Co.
Yachi, S., & Loreau, M. (1999). Biodiversity and ecosystem productivity in a fluctuating environment: The insurance hypothesis. Proceedings of the National Academy of Sciences, 96, 1463-1468.
Zheng, J., Payne, J. L., & Wagner, A. (2019). Cryptic genetic variation accelerates evolution by opening access to diverse adaptive peaks. Science, 365(6451), 347-353.
Zhu, Y., Chen, H., Fan, J., Wang, Y., Li, Y., Chen, J., Fan, J., Yang, S., Hu, L., Leung, H., Mew, T. W., Teng, P. S., Wang, Z., & Mundt, C. C. (2000). Genetic diversity and disease control in rice. Nature, 406(6797), 718-722.