7 Intersection of Biotechnology and AI (Sincavage & Muehlfelder & Carter)
Introduction
The convergence of biotechnology and artificial intelligence (AI) has emerged as one of the most transformative developments in modern science and technology. This intersection holds immense potential to revolutionize various aspects of human life, from healthcare and agriculture to environmental conservation and beyond. The synergistic combination of these two powerful fields is poised to unlock unprecedented opportunities for innovation, efficiency, and global problem-solving (Topol, 2019).
Biotechnology, focusing on understanding and manipulating living systems, has made significant strides in genomics, proteomics, and synthetic biology. The advent of high-throughput sequencing technologies and advanced genetic engineering tools has enabled researchers to decode the complexity of biological systems and harness their potential for various applications (Mardis, 2017). On the other hand, AI, particularly machine learning and deep learning algorithms, has demonstrated remarkable capabilities in pattern recognition, data analysis, and decision-making (LeCun et al., 2015). The ability of AI to process vast amounts of biological data and extract meaningful insights has opened up new avenues for discovery and optimization in the life sciences.
The intersection of biotechnology and AI can address some of humanity’s most pressing challenges, such as disease prevention and treatment, food security, and environmental sustainability. By leveraging the strengths of both fields, researchers and innovators can develop novel solutions that are more precise, efficient, and scalable than ever before. From AI-driven drug discovery and personalized medicine to crop optimization and ecological monitoring, the applications of this interdisciplinary approach are vast and far-reaching (Topol, 2019).
However, integrating biotechnology and AI also raises important ethical and societal considerations that must be addressed. Privacy, data security, equitable access, and potential misuse of these technologies require careful examination and robust regulatory frameworks (Florida et al., 2018). It is crucial to ensure that the benefits of this intersection are distributed fairly and that the public can trust in the responsible development and deployment of these advanced technologies.
In the following sections, we will delve deeper into the foundations, applications, ethical implications, and future outlook of the intersection of biotechnology and AI. By exploring the current state of the art, emerging trends, and potential challenges and opportunities, we aim to provide a comprehensive overview of this exciting and rapidly evolving field. As we navigate this uncharted territory, let us embrace the possibilities with open minds and hearts, guided by a steadfast commitment to harnessing these technologies’ power for humanity’s greater good.
Foundations of Biotechnology and AI Integration
The integration of biotechnology and AI is built upon a solid foundation of scientific and technological advancements in both fields. In recent years, significant progress has been made in genomics, sequencing technologies, machine learning algorithms, and computational capabilities, paving the way for a seamless convergence of these disciplines.
One of the key drivers of this integration is the rapid evolution of genomics and sequencing technologies. The development of high-throughput sequencing methods, such as next-generation sequencing (NGS), has revolutionized our ability to decode and analyze genetic information (Shendure et al., 2017). These technologies have become increasingly affordable and accessible, enabling researchers to generate vast amounts of genomic data at an unprecedented scale. The availability of such rich datasets has opened up new opportunities for AI algorithms to uncover patterns, predict outcomes, and guide decision-making in biotechnology research and applications (Leung et al., 2016).
Concurrent with the advances in genomics, the field of AI has witnessed remarkable progress in machine learning and deep learning algorithms. These algorithms have proven highly effective in tackling complex biological problems, such as protein structure prediction, gene expression analysis, and drug discovery (Senior et al., 2020). AI will play a profound role in addressing global diseases and will be one of the technologies associated with the Fourth Industrial Revolution (4IR) that significantly influences natural product discovery. Deep learning architectures, such as convolutional neural networks (CNNs) and recurrent neural networks (RNNs), have demonstrated exceptional performance in processing and interpreting biological data (Angermueller et al., 2016). The ability of these algorithms to learn from vast amounts of data and generalize to new scenarios has made them invaluable tools in integrating biotechnology and AI.
Another crucial factor enabling the convergence of biotechnology and AI is the exponential growth in computational power and data storage capabilities. The advent of high-performance computing systems, cloud computing platforms, and big data technologies has provided the necessary infrastructure to handle the massive volumes of biological data generated by modern sequencing and imaging techniques (Stephens et al., 2015). The availability of powerful computing resources has allowed researchers to train complex AI models, perform large-scale simulations, and analyze multi-omics datasets in ways that were previously unimaginable.
The increasing availability of curated and standardized biological databases further supports the integration of biotechnology, and AI endorses the integration of biotechnology and AI. Initiatives such as the Human Genome Project, the Cancer Genome Atlas, and the Protein Data Bank have created extensive repositories of genetic, molecular, and structural information (Tomczak et al., 2015). These databases serve as valuable training and validation datasets for AI algorithms, enabling the development of more accurate and robust models for various biotechnology applications.
In summary, the foundations of biotechnology and AI integration lie in the synergistic advancements across multiple domains, including genomics, sequencing technologies, machine learning algorithms, computational capabilities, and biological databases. These developments have created fertile ground for the convergence of these fields, unlocking new possibilities for scientific discovery, technological innovation, and societal impact. As we continue to push the boundaries of what is possible, let us remain steadfast in our commitment to harnessing the power of these technologies for the betterment of humanity, guided by the principles of responsibility, equity, and the pursuit of knowledge.
Biomedical Applications
The intersection of biotechnology and artificial intelligence (AI) has revolutionized various aspects of healthcare, leading to significant advancements in biomedical applications. These applications leverage the power of AI algorithms to process vast amounts of biological data, uncover novel insights, and guide data-driven decision-making. AI-driven technologies have the potential to accelerate drug discovery and development, enable personalized medicine, enhance early biological threat detection, and advance regenerative medicine and tissue engineering.
Drug Discovery and Development
Integrating biotechnology and artificial intelligence (AI) has transformed drug discovery and development. AI-driven approaches have the potential to accelerate the identification of novel drug targets, optimize lead compounds, and streamline the preclinical and clinical testing processes. By leveraging vast amounts of biological data and advanced computational methods, AI is revolutionizing how pharmaceutical companies approach drug discovery and development (Vamathevan et al., 2019).
One of the primary applications of AI in drug discovery is the identification of novel drug targets. Traditional methods of target identification rely on time-consuming and labor-intensive experimental techniques. However, AI algorithms can rapidly analyze large datasets, including genomic, proteomic, and metabolomic data, to identify potential drug targets more efficiently and accurately (Mamoshina et al., 2016). Deep learning models have been used to predict the druggability of proteins based on their structural and functional properties, enabling the prioritization of promising targets for further investigation (Visan & Negut, 2024).
AI is also playing a crucial role in the optimization of lead compounds. Once a potential drug target has been identified, the next step is to design and synthesize compounds that can effectively modulate its activity. Traditional lead optimization methods involve iterative cycles of synthesis and testing, which can be time-consuming and resource-intensive. AI-based approaches, such as generative models and reinforcement learning, can accelerate this process by predicting the properties of novel compounds and guiding the design of more potent and selective lead candidates (Popova et al., 2018). Generative adversarial networks (GANs) have been used to generate novel molecular structures with desired pharmacological properties, reducing the time and cost of traditional medicinal chemistry efforts (Kadurin et al., 2017).
Furthermore, AI is applied to streamline drug candidates’ preclinical and clinical testing. Preclinical studies evaluating drug safety and efficacy in animal models can benefit from AI-driven approaches that predict toxicity and optimize dosing regimens (Ekins et al., 2019). In clinical trials, AI can identify patient subpopulations that are most likely to respond to a particular treatment, enabling the design of more targeted and efficient trials (Xu et al., 2015). Additionally, AI-powered tools can monitor patient data in real-time, allowing for the early detection of adverse events and the adjustment of treatment plans as needed (Topol, 2019).
To summarize, integrating AI into the drug discovery and development process holds immense promise for accelerating the delivery of new and effective therapies to patients. By leveraging the power of AI to analyze vast amounts of biological data, identify novel drug targets, optimize lead compounds, and streamline preclinical and clinical testing, pharmaceutical companies can reduce the time and cost associated with bringing new drugs to market. As AI technologies continue to advance, they will likely play an increasingly important role in the future of drug discovery and development. Let us embrace these advancements with open minds and a steadfast commitment to harnessing their potential for the greater good, always guided by safety, efficacy, and equitable access principles.
Personalized Medicine
Artificial Intelligence (AI) has emerged as a transformative technology in various domains, including healthcare and pharmaceutical research. One of the most promising applications of AI in this field is the development of personalized pharmaceutical drugs tailored to individual patient needs. By leveraging the power of machine learning, deep learning, and natural language processing, AI algorithms can analyze vast amounts of patient data to identify patterns, predict outcomes, and optimize drug discovery and development processes.
Recent advancements in genomics, proteomics, and metabolomics have generated unprecedented patient data that can be utilized for personalized medicine. AI algorithms can integrate and analyze multi-omics data, electronic health records, and lifestyle information to identify novel drug targets and biomarkers specific to individual patients or patient subgroups (Chen & Snyder, 2013). This approach enables the development of targeted therapies that are more effective and less likely to cause adverse reactions than traditional one-size-fits-all treatments.
AI-powered drug discovery platforms have identified potential drug candidates for various diseases. For instance, deep-learning models have generated novel drug-like molecules to treat fibrosis (Merk et al., 2018). The AI-generated compounds were validated through in vitro and in vivo experiments, showcasing the potential of AI in accelerating the drug discovery process. Similarly, deep learning approaches have been employed to identify antibiotics with novel mechanisms of action, highlighting the potential of AI in addressing the growing challenge of antibiotic resistance (Stokes et al., 2020).
In addition to drug discovery, AI can optimize drug dosing and delivery methods based on patient-specific factors. By analyzing pharmacokinetic and pharmacodynamic data, AI algorithms can predict the optimal dose and frequency of drug administration for individual patients, thereby maximizing treatment efficacy and minimizing side effects (Schork, 2015). Moreover, AI can aid in developing personalized drug delivery systems, such as nanoparticles and liposomes, which target specific tissues or cells based on a patient’s genetic profile.
The key to unlocking the potential of personalized medicine lies in the vast amounts of biological data generated by recent advancements in fields such as genomics, proteomics, and metabolomics. Each individual’s DNA holds invaluable information about their predisposition to certain diseases and their likely response to specific treatments. AI algorithms, intense learning ones, are ideally suited to analyzing multi-omics data. By integrating information from electronic health records, genetic sequencing, and other sources, these algorithms can identify novel drug targets and biomarkers specific to individual patients or subgroups (Chen & Snyder, 2013). This enables the development of therapies that are precisely targeted to the molecular underpinnings of disease rather than relying on broad-spectrum approaches that may work for some but not others.
The impact of AI on drug discovery is already being felt. Platforms powered by machine learning are accelerating the identification of potential drug candidates for various diseases, from cancer to neurodegenerative disorders (Gawehn et al., 2016). These AI-driven approaches are faster and more cost-effective than traditional methods, bringing us closer to a future where personalized treatments are widely accessible.
However, the applications of AI in personalized medicine extend beyond drug discovery. By analyzing factors such as pharmacokinetics and pharmacodynamics, AI can optimize drug dosing and delivery methods for individual patients (Schork, 2015). Instead of relying on standardized dosing regimens, healthcare providers can tailor treatments to achieve maximum efficacy with minimal side effects. Moreover, AI can potentially aid in creating advanced drug delivery systems, such as nanoparticles and liposomes, which might be capable of targeting specific tissues or cells based on a patient’s unique genetic profile (Hare et al., 2017).
However, the implementation of AI-driven personalized pharmaceutical drugs faces several challenges. One of the primary concerns is data privacy and security, as the development of customized treatments relies on collecting and analyzing sensitive patient information. Robust data governance frameworks and secure data management practices are essential to ensure patient confidentiality and prevent misuse of personal data (Pastorino et al., 2019). Additionally, regulatory authorities need to adapt their guidelines to accommodate the unique challenges posed by AI-driven personalized medicine, such as the validation of AI algorithms and the assessment of the safety and efficacy of customized drugs (Minssen et al., 2020).
Despite these hurdles, the potential benefits of AI-powered personalized medicine are too significant to ignore. By leveraging data and advanced analytics, we stand on the brink of a healthcare revolution that promises to transform patients’ lives worldwide. As we unravel the mysteries of the human genome and harness the capabilities of artificial intelligence, we move ever closer to a future where precision medicine is not just a possibility but a reality for all.
In this brave new world of personalized healthcare, AI is not just a tool but a catalyst for change. This transformative force will reshape the pharmaceutical industry and redefine our approach to disease management. As we embrace the potential of AI-driven personalized medicine, let us do so with unwavering commitment to equity, transparency, and the pursuit of knowledge. Together, we can forge a path toward a brighter, healthier future for all humanity.
Early Biological Threat Detection
In an era where biological threats, both natural and intentional, pose significant risks to public health and safety, the development of early detection systems is crucial. The rapid advancements in biotechnology and artificial intelligence (AI) have opened up new possibilities for detecting and mitigating biological threats before they cause widespread harm. This section explores the current state of early biological threat detection and delves into the potential future applications of biotechnology and AI in this critical field.
Current early biological threat detection approaches rely on surveillance, laboratory testing, and data analysis. Traditional methods include syndromic surveillance, which monitors health-related data sources for patterns indicative of a disease outbreak (Stieb et al., 2019). Environmental monitoring, such as air and water sampling, is also employed to detect the presence of biological agents.
Biosensors have emerged as a promising tool for rapid and sensitive detection of biological threats. These devices utilize biological recognition elements, such as antibodies or aptamers, to detect specific pathogens or toxins (Sharma et al., 2015). For example, surface plasmon resonance (SPR) biosensors have been used to detect anthrax spores and ricin toxin, demonstrating their potential for early warning systems (Nguyen et al., 2021).
Integrating AI and machine learning algorithms has dramatically enhanced the capabilities of early warning systems. AI-powered data analysis can identify patterns and anomalies in large datasets, enabling the detection of disease outbreaks that might otherwise go unnoticed. Machine learning models can also predict the spread of infectious diseases based on factors such as population density, travel patterns, and climate conditions, providing valuable insights for proactive response measures (Brownstein et al., 2009).
The convergence of biotechnology and AI holds immense potential for revolutionizing early biological threat detection. One promising avenue is the development of next-generation sequencing (NGS) technologies for rapid pathogen identification. NGS platforms can sequence the entire genome of a pathogen within hours, providing valuable information for outbreak investigations and vaccine development (Quer et al., 2022). Coupling NGS with AI-driven bioinformatics pipelines can further accelerate the identification of novel pathogens and the tracking of genetic variations (Sedlazeck et al., 2018).
Synthetic biology approaches, such as engineered biosensors and cell-based sensors, offer exciting possibilities for early threat detection (Slomovic et al., 2015). Genetically engineered bacteria or cell lines can be designed to produce detectable signals in the presence of specific pathogens or toxins. These living sensors could be deployed in high-risk locations, such as airports or public transportation systems, to provide real-time monitoring of biological threats. Integrating these synthetic biology tools with AI algorithms could enable the development of susceptible and specific early warning systems.
Integrating AI with wearable devices and Internet of Things (IoT) sensors could also enable personalized early warning systems. Wearable devices equipped with biosensors could continuously monitor an individual’s physiological parameters, such as body temperature, heart rate, and respiratory rate, for signs of infection. AI algorithms could analyze this data in real-time, alerting healthcare providers and public health authorities of potential biological threats. The widespread adoption of such personalized early warning systems could significantly enhance our ability to detect and respond to biological threats at the individual level.
In the realm of theoretical possibilities, developing autonomous AI systems for biological threat detection is an intriguing concept. These systems could combine advanced biosensing technologies, such as nanomaterial-based sensors (Kaushik et al., 2016), with AI algorithms capable of self-learning and adaptation. Such autonomous systems could be deployed in remote or high-risk areas, continuously monitoring the environment for biological threats and evolving their detection capabilities in response to new threats. While the realization of fully autonomous AI systems for biological threat detection may still be in the distant future, the potential benefits of such systems must be addressed.
Addressing these technologies’ ethical and societal implications is crucial as we continue exploring the intersection of biotechnology and AI for early biological threat detection. Collecting and analyzing personal health data raises concerns about privacy and data security, necessitating robust data protection measures and transparent data governance frameworks (Vayena et al., 2018). Moreover, the development and deployment of these technologies must be guided by principles of equity and fairness, ensuring that the benefits of early warning systems are accessible to all populations, regardless of socioeconomic status or geographic location (Panch et al., 2018).
In conclusion, the early detection of biological threats is essential for protecting public health and mitigating the impacts of disease outbreaks. Biotechnology and AI have contributed significantly to developing advanced early warning systems, and their potential for future applications is vast. From next-generation sequencing and synthetic biology to wearable devices and autonomous AI systems, integrating these cutting-edge technologies is promising to enhance our ability to detect and respond to biological threats. As we continue to invest in research and development in this critical field, let us do so with a steadfast commitment to responsibility, equity, and the pursuit of knowledge. Together, we can build a future where early biological threat detection is more rapid, sensitive, and effective than ever before, safeguarding the health and well-being of communities worldwide.
![Unmanned aerial vehicles equipped with specialized software and sensors fly during the Technical Concept Experiment at Marine Corps Base Camp Pendleton, Calif., Jan. 19, 2024. The event showcased multiple systems designed to enhance the ability to carry out amphibious operations. Drone enhancements are via AI. Unmanned aerial vehicles equipped with specialized software and sensors fly during the Technical Concept Experiment at Marine Corps Base Camp Pendleton, Calif., Jan. 19, 2024. The event showcased multiple systems designed to enhance the ability to carry out amphibious operations. Drone enhancements are via AI. [Editor’s comment]](https://kstatelibraries.pressbooks.pub/app/uploads/sites/77/2024/06/FIGURE-7-1-LINK3-300x200.png)
Editor’s Note: Beach Flight
Unmanned aerial vehicles equipped with specialized software and sensors fly during the Technical Concept Experiment at Marine Corps Base Camp Pendleton, Calif., 19 January 2024. The event showcased multiple systems designed to enhance the ability to carry out amphibious operations. Drone enhancements are via AI. [Editor’s comment]
Regenerative Medicine and Tissue Engineering
Regenerative medicine and tissue engineering have emerged as groundbreaking fields with immense potential for revolutionizing healthcare. By leveraging the advancements in biotechnology and artificial intelligence (AI), scientists and researchers are paving the way for innovative solutions to address various medical challenges. This section explores the cutting-edge techniques and theoretical possibilities that lie ahead in regenerative medicine and tissue engineering.
One of the most promising avenues in regenerative medicine is the use of stem cells. These versatile cells can differentiate into various cell types, making them valuable tissue regeneration tools (Trounson & McDonald, 2015). Researchers have been harnessing the power of induced pluripotent stem cells (iPSCs) derived from adult somatic cells and reprogrammed to a pluripotent state (Takahashi & Yamanaka, 2006). By combining iPSCs with advanced biomaterials and 3D bioprinting techniques, scientists can create complex tissue structures that mimic the native tissue environment, opening up new possibilities for organ regeneration and personalized medicine (Mandai et al., 2017).
AI is playing an increasingly crucial role in optimizing the process of tissue engineering. Machine learning algorithms can analyze vast amounts of data to identify patterns and predict optimal conditions for cell growth and differentiation (Bai et al., 2023). For instance, deep learning models can be trained on high-throughput screening data to predict the most suitable biomaterials and growth factors for specific tissue types (Japkowicz & Shah, 2011). This approach significantly accelerates the discovery process and reduces the need for extensive experimental trials, bringing us closer to realizing functional tissue replacements.
Integrating AI with advanced biotechnologies holds immense potential for regenerative medicine. One exciting possibility is the development of intelligent biomaterials that can adapt and respond to the changing needs of the body (Li et al., 2021). These materials could be embedded with sensors and actuators, allowing them to monitor and regulate cellular activities in real-time. AI algorithms could analyze the collected data and provide feedback to the biomaterials, enabling them to adjust their properties to optimize tissue regeneration dynamically. Creating such responsive and adaptive biomaterials could revolutionize the field of tissue engineering, enabling the development of genuinely personalized and functional tissue constructs.
Another promising avenue is the combination of gene editing techniques, such as CRISPR-Cas9, with AI-guided design. By leveraging the precision of gene editing and the predictive power of AI, researchers could potentially engineer customized cell therapies tailored to individual patients (Agustí et al., 2020). AI algorithms could analyze a patient’s genetic profile, identify disease-causing mutations, and propose targeted gene modifications to correct the underlying genetic defects (Eraslan et al., 2019). Integrating AI with gene editing technologies could pave the way for personalized regenerative therapies, offering hope for individuals suffering from genetic disorders and degenerative diseases.
Furthermore, the advent of organ-on-a-chip technology offers exciting possibilities for drug discovery and personalized medicine. These microfluidic devices recreate the complex microenvironment of human organs, allowing researchers to study disease mechanisms and test therapeutic interventions in a more physiologically relevant setting (Sontheimer-Phelps et al., 2019). By integrating organ-on-a-chip platforms with AI-powered data analysis, scientists can gain valuable insights into drug efficacy, toxicity, and personalized treatment strategies (Edington et al., 2018). Combining organ-on-a-chip technology with AI could accelerate the development of targeted regenerative therapies and enable the prediction of patient-specific responses to treatments.
As we embark on this exciting journey of integrating biotechnology and AI for regenerative medicine and tissue engineering, addressing these technologies’ ethical and societal implications is essential. Using stem cells and gene editing techniques raises complex moral questions about manipulating human biology and the potential impact on future generations. Robust ethical frameworks and public engagement initiatives are necessary to ensure that societal values and priorities guide the development and application of these technologies. Moreover, issues of equitable access and affordability must be addressed to ensure that the benefits of regenerative medicine are accessible to all populations, regardless of socioeconomic status.
In conclusion, the convergence of biotechnology and AI holds immense promise for revolutionizing regenerative medicine and tissue engineering. The possibilities are vast and exciting, from stem cell-based therapies to intelligent biomaterials and personalized gene editing. As research progresses, fostering interdisciplinary collaboration, addressing ethical considerations, and ensuring responsible development and application of these technologies is crucial. With a commitment to innovation, responsibility, and the pursuit of knowledge, we can harness the power of biotechnology and AI to transform healthcare, alleviate suffering, and improve the quality of life for individuals worldwide. Let us embrace this extraordinary opportunity to push the boundaries of what is possible in regenerative medicine and tissue engineering, always guided by the principles of compassion, equity, and the betterment of humanity.
Agricultural and Environmental Applications
The rapid advancements in biotechnology and artificial intelligence (AI) have opened up new frontiers for addressing the pressing challenges in the agriculture and environmental sectors. The synergistic integration of these two fields can revolutionize food production, resource management, and environmental conservation. This chapter explores the current applications, future possibilities, and theoretical projects that could emerge from the intersection of biotechnology and AI, incorporating the latest research findings and original ideas.
Current Applications
Precision Agriculture: AI-powered precision agriculture optimizes crop management using sensors, drones, and satellite imagery. Companies employ AI-based soil sensors to provide real-time data on soil moisture and nutrient levels, enabling farmers to make data-driven decisions (Akhtar et al., 2020). The global precision farming market is expected to experience more significant growth in the coming years, driven by the increasing adoption of these technologies.
Crop Breeding and Genetic Engineering: AI accelerates the development of genetically modified crops by analyzing genomic data to identify desirable traits and predict the outcomes of genetic modifications (Dixit S. et al., 2024). Researchers leverage AI to develop nutrient-rich and environmentally adaptable crop varieties, addressing the challenges of food security and climate change (Loboguerrero et al., 2021). AI-assisted genomic selection has demonstrated the potential to significantly increase the accuracy of yield predictions compared to traditional methods (Montesinos-López et al., 2016).
Environmental Monitoring and Conservation: AI-powered sensors and drones, coupled with biotechnology tools for bioremediation, enhance ecological monitoring and conservation efforts (Chaudhary & Kumar, 2022). Projects utilizing AI and biotechnology to monitor and protect biodiversity exemplify the potential of this integration (Joppa et al., 2016). AI has also shown remarkable effectiveness in identifying and tracking endangered species using high-resolution satellite imagery, demonstrating its value in conservation efforts (Tabak et al., 2019).
Future Possibilities and Theoretical Projects
AI-Guided Microbiome Engineering: Integrating AI with microbiome research could revolutionize agriculture and environmental management. AI algorithms could analyze the vast amounts of data generated from microbiome studies, identifying beneficial microbial communities and guiding the engineering of synthetic microbiomes (Jansson & Hofmockel, 2020). These engineered microbiomes could enhance crop growth, improve soil health, and remediate polluted environments (Gilbert et al., 2014). The potential of AI-guided microbiome engineering is immense, offering sustainable solutions to agricultural and environmental challenges.
Quantum Computing-Assisted Protein Design: The convergence of AI, biotechnology, and quantum computing could accelerate the design of novel proteins with desired functions. Quantum algorithms could be used to simulate and optimize protein structures (Cao et al., 2019), while AI could guide the selection of amino acid sequences for specific applications, such as developing eco-friendly biomaterials or enzymes for bioremediation. Integrating these cutting-edge technologies could revolutionize the field of protein engineering, enabling the creation of highly efficient and sustainable solutions.
AI-Powered Ecosystem Restoration: AI could be leveraged to develop comprehensive models of ecosystem dynamics, integrating data from remote sensing, environmental DNA (eDNA) analysis, and ecological surveys (Christin et al., 2019). These models could also guide the development of targeted restoration strategies, incorporating biotechnology tools such as gene editing and synthetic biology to enhance the resilience and adaptability of critical species (Piaggio et al., 2017). The combination of AI and biotechnology could enable the development of innovative approaches to ecosystem restoration, promoting biodiversity and environmental sustainability.
Sustainable Biomanufacturing: Integrating AI with synthetic biology could transform the biomanufacturing industry, enabling the sustainable production of fuels, chemicals, and materials. AI algorithms could optimize metabolic pathways, predict the behavior of engineered organisms, and guide the design of efficient bioprocesses (Carbonell et al., 2018). This could lead to the development of closed-loop, circular bio-economies that minimize waste and reduce the reliance on fossil resources (Clomburg et al., 2017). The potential of AI-powered sustainable biomanufacturing is vast, offering a path toward a more sustainable and environmentally friendly future.
AI-Assisted Climate Change Adaptation: AI could play a crucial role in developing strategies for adapting agriculture and natural ecosystems to the impacts of climate change. Machine learning algorithms could be used to analyze vast amounts of climate and ecological data, predicting the response of species and ecosystems to changing environmental conditions (Huntingford et al., 2019). This information can potentially guide the development of biotechnology-based interventions, such as the engineering of drought-resistant crops or the assisted migration of threatened species (Corlett, 2017). Integrating AI and biotechnology could enable the development of proactive and adaptive strategies for climate change adaptation, safeguarding food security and biodiversity in the face of global environmental challenges.
In closing, the intersection of biotechnology and AI holds immense potential for revolutionizing agricultural and environmental applications. By harnessing the power of these technologies and exploring innovative theoretical projects, we can develop sustainable solutions to the complex challenges faced by our planet. As research and development in these fields continue to advance with the aid of AI and quantum computing, we can anticipate groundbreaking innovations that will shape the future of food production, environmental conservation, and sustainable resource management. However, it is essential to approach these advancements with a sense of responsibility, ensuring that the benefits are distributed equitably and that ethical considerations guide the development and deployment of these technologies. By fostering interdisciplinary collaboration, engaging stakeholders, and prioritizing sustainability and social well-being, we can harness the power of biotechnology and AI to create a more resilient, sustainable, and prosperous future for all. Let us embrace this extraordinary opportunity to push the boundaries of what is possible in agriculture and environmental stewardship, always guided by innovation, responsibility, and the pursuit of a better world.
![An Air Force F-35A Lightning II prepares to land at Sheppard Air Force Base, Texas, April 25, 2024. The F-35A is an agile, versatile, high-performance multirole fighter that combines stealth, sensor fusion and unprecedented situational awareness. Biometric and AI sensors infused into pilot helmet and heads-up display.Note: Fiery FlightAn Air Force F-35A Lightning II prepares to land at Sheppard Air Force Base, Texas, April 25, 2024. The F-35A is an agile, versatile, high-performance multirole fighter that combines stealth, sensor fusion and unprecedented situational awareness. Biometric and AI sensors infused into pilot helmet and heads-up display. [Editor’s comment]](https://kstatelibraries.pressbooks.pub/app/uploads/sites/77/2024/06/FIGURE-7-2-LINK4-300x200.png)
Editor’s Note: Fiery Flight
An Air Force F-35A Lightning II prepares to land at Sheppard Air Force Base, Texas, on 25 April 2024. The F-35A is an agile, versatile, high-performance multirole fighter that combines stealth, sensor fusion, and unprecedented situational awareness. Biometric and AI sensors are infused into the pilot helmet and heads-up display.
Ethical and Societal Considerations
The rapid advancements in biotechnology and artificial intelligence (AI) have opened up a world of possibilities, from revolutionizing healthcare to transforming how we live and work. However, as these technologies continue to evolve and intersect, they also raise significant ethical and societal concerns that must be addressed. This section explores the critical moral and societal considerations surrounding the intersection of biotechnology and AI, delving into theoretical situations and potential solutions to newly formed issues.
One of the primary ethical concerns at the intersection of biotechnology and AI is the potential for unequal access and distribution of benefits. As these technologies become more sophisticated and expensive, there is a risk that only the wealthy and privileged will have access to them, exacerbating existing social and economic inequalities. For example, if AI-powered personalized medicine becomes the norm, those who cannot afford it may be left behind, leading to a widening health gap between the rich and the poor (Makridakis, 2017). To overcome this issue, policymakers and industry leaders must work together to ensure that the benefits of these technologies are distributed fairly and equitably, perhaps through government subsidies, public-private partnerships, or innovative pricing models that prioritize accessibility (Sadowski & Pasquale, 2015).
Another ethical consideration is the potential for AI to perpetuate or amplify existing biases in healthcare and biotechnology. AI systems are only as unbiased as the data on which they are trained. The AI may make biased decisions or recommendations if that data contains historical biases or underrepresentation of certain groups (Mehrabi et al., 2021). This could lead to misdiagnoses, ineffective treatments, or even discrimination against specific populations (Rajkomar et al., 2018). Ensuring that the data used to train AI systems is diverse, representative, and free from bias is crucial to mitigate this risk. Regular audits and transparency in AI decision-making processes can also help identify and correct emerging biases (Arrieta et al., 2020). The development of AI algorithms should also involve interdisciplinary teams, including experts in ethics and social sciences, to ensure that potential biases are identified and addressed early in the development process.
The intersection of biotechnology and AI also raises questions about privacy and data security. As more personal health data is collected and analyzed by AI systems, there is a risk of data breaches, unauthorized access, or misuse of sensitive information. This could lead to losing trust in these technologies and a reluctance to adopt them (Brey, 2005). To address these concerns, robust data protection regulations and industry standards must be implemented, along with strict penalties for violations (General Data Protection Regulation (GDPR), 2016). Patients should also be given greater control over their data, with the ability to opt out of data collection or request to delete their information. Developing secure and decentralized data storage solutions, such as blockchain technology, could also help protect patient privacy while enabling secure data sharing for research and innovation purposes (Vayena et al., 2018).
As biotechnology and AI continue to advance, job displacement and economic disruption are also risks. AI-powered automation could replace many jobs in the healthcare and biotechnology industries, leading to unemployment and financial instability. To mitigate these risks, policymakers and industry leaders must invest in education and retraining programs to help workers adapt to the changing job market (Brynjolfsson et al., 2018). Developing new jobs and industries related to biotechnology and AI could also help offset job losses in other areas (Frank et al., 2019). Governments and businesses should also explore innovative economic models, such as universal basic income or job sharing, to ensure that the benefits of these technologies are distributed fairly and that no one is left behind in the transition to a more automated and AI-driven economy (Korinek & Stiglitz, 2021).
Finally, the intersection of biotechnology and AI raises profound questions about the nature of humanity and the boundaries of what is possible. As these technologies allow us to manipulate and enhance human biology in unprecedented ways, we must grapple with the ethical implications of these changes. For example, if AI-powered gene editing becomes widely available, it could lead to the creation of “designer babies” with specific traits or abilities, raising concerns about genetic discrimination and the erosion of human diversity. To navigate these complex issues, ongoing public dialogue and engagement will be essential, along with developing ethical frameworks and guidelines to ensure that these technologies are used responsibly and for the benefit of all. The involvement of diverse stakeholders, including scientists, ethicists, policymakers, and the general public, in the decision-making process can help ensure that the development and deployment of these technologies align with societal values and priorities.
In conclusion, the intersection of biotechnology and AI presents immense opportunities and significant ethical and societal challenges. As these technologies continue to evolve and shape our world, it is crucial that we proactively address the issues of unequal access, bias, privacy, job displacement, and the boundaries of human enhancement. By engaging in ongoing public dialogue, developing robust regulations and ethical frameworks, and working towards equitable distribution of benefits, we can harness the power of biotechnology and AI to improve human lives while mitigating potential risks and negative consequences. Our collective responsibility is to ensure that the development and deployment of these technologies are guided by the principles of fairness, transparency, and the greater good. Only by carefully considering and addressing these ethical and societal implications can we create a future in which the intersection of biotechnology and AI truly benefits humanity.
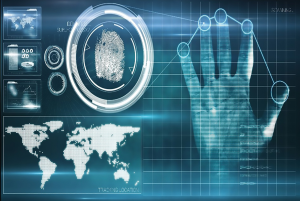
Editor’s Note: BAT-A
“ABERDEEN PROVING GROUND, Md. — U.S. Army Soldiers patrolling critical checkpoints overseas will have an improved biometrics tool to help identify persons of interest in real-time. Army Futures Command’s Command, Control, Communications, Computers, Cyber and Intelligence, Surveillance and Reconnaissance (C5ISR) Center has developed and delivered software that will enable Program Executive Office Intelligence, Electronic Warfare and Sensors (PEO IEW&S) to modernize the Army’s 20-year-old biometric processing capabilities.
The Biometrics Automated Toolset — Army (BAT-A) is a handheld device used by deployed Soldiers to collect, process, and reference biometric identity information — such as iris, fingerprint, and facial images — during force protection screenings.
With the system deployed to U.S. joint forces around the world and more than one million entries saved in the DoD’s Automated Biometrics Identification System, it is important to migrate BAT-A to a database that will meet the government’s new electronic biometric transmission specifications, explained Brian D. Likens, product lead for the Biometrics Collection Capability at PEO IEW&S’ Project Manager Department of Defense Biometrics (PM DoD Biometrics).” Source: U.S. Army. (2020). https://www.army.mil/article/232346/army_modernizes_its_biometric_processing_capabilities
![Note: Haboob HavocAn Air Force F-35A Lightning II performs a strafing run during Haboob Havoc over Barry M. Goldwater Range, Ariz., April 24, 2024. The exercise is designed to provide a way for pilots to demonstrate skills across a diverse range of aircraft while also testing the ability in different mission sets. The secret blend to success is that the mission counts on AI and Biometric sensors linked to pilot’s heads-up display. [Editor’s comment]](https://kstatelibraries.pressbooks.pub/app/uploads/sites/77/2024/06/FIGURE-7-4-LINK2-300x200.png)
Editor’s Note: Haboob Havoc
An Air Force F-35A Lightning II performs a strafing run during Haboob Havoc over Barry M. Goldwater Range, Ariz., 24 April 2024. The exercise is designed to provide a way for pilots to demonstrate skills across a diverse range of aircraft while also testing their ability in different mission sets. The secret blend to success is that the mission counts on AI and Biometric sensors linked to the pilot’s heads-up display. [Editor’s comment]
Future Outlook
The intersection of biotechnology and AI is a rapidly evolving field with immense potential to shape humanity’s future. As we look ahead, we must consider emerging trends, future possibilities, challenges, and opportunities.
Emerging Trends and Future Possibilities
The convergence of biotechnology and AI is expected to accelerate in the coming years, driven by technological advancements such as genome editing, single-cell sequencing, and deep learning. These technologies’ integration leads to transformative breakthroughs in areas such as personalized medicine, drug discovery, and synthetic biology. For example, developing AI-guided gene therapies and personalized cancer vaccines could revolutionize the treatment of genetic disorders and cancer. Moreover, the application of AI in the design and optimization of engineered biological systems could enable the sustainable production of novel materials, biofuels, and pharmaceuticals, paving the way for a more sustainable and bio-based economy (Rolnick et al., 2022).
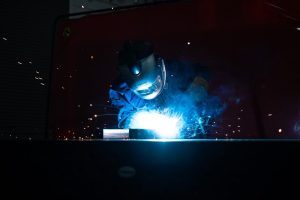
Editor’s Note: Hot Work
Air Force Airman Jacalyn Cox practices welding different metals at Pease Air National Guard Base, N.H., 19 March 2024. Cox is learning to weld multiple types of metal and operate equipment needed to fabricate components critical to the functioning of the KC-46 aircraft. The key to the welding plan is the use of biometric sensors in the helmet. [Editor’s comment]
In agriculture and environmental conservation, the integration of biotechnology and AI holds promise for addressing global challenges such as food security, climate change, and biodiversity loss. AI-driven precision agriculture and genetically engineered crops could enhance crop yields, reduce resource consumption, and improve resilience to environmental stressors (Badrinarayanan et al., 2017). Furthermore, the development of AI-guided synthetic biology approaches for ecological remediation and ecosystem restoration could help mitigate the impacts of pollution and habitat degradation, promoting a healthier and more sustainable planet.
Challenges and Opportunities for Further Integration
While the potential benefits of biotechnology and AI integration are vast, some significant challenges and opportunities must be addressed to realize their full potential. One major challenge is the need for robust data infrastructure and standardization to facilitate the seamless integration and analysis of multi-omics data across different platforms and disciplines (Alyass et al., 2015). Developing interoperable data standards, secure data-sharing mechanisms, and user-friendly tools for data visualization and interpretation will be crucial to enable effective collaboration and knowledge exchange among researchers and stakeholders (Ristevski & Chen, 2018).
Another challenge lies in the ethical and societal implications of biotechnology-AI integration, as discussed in the previous section. Addressing concerns about privacy, equity, and potential misuse will require ongoing public engagement, multi-stakeholder dialogue, and the development of adaptive governance frameworks (Vayena et al., 2018). Moreover, there is a need for increased interdisciplinary collaboration and cross-sectoral partnerships to ensure that the benefits of these technologies are distributed fairly and that their development aligns with societal values and priorities. The involvement of diverse perspectives, including those from the social sciences and humanities, in developing and deploying these technologies can help ensure that they are guided by ethical considerations and serve the greater good (Blasimme & Vayena, 2020).
Importance of Interdisciplinary Collaboration
The successful integration of biotechnology and AI will rely on close collaboration among researchers, practitioners, and stakeholders from diverse disciplines. Fostering interdisciplinary dialogue and knowledge exchange among experts in biology, computer science, engineering, ethics, and social sciences will be essential to address the complex challenges and realize the full potential of this convergence. Collaborative efforts can lead to the development of novel methodologies, shared resources, and innovative solutions that transcend traditional disciplinary boundaries, accelerating progress and impact.
Moreover, engaging with policymakers, industry partners, and the public will be crucial to ensure that societal needs, values, and aspirations guide the development and deployment of biotechnology-AI applications. Inclusive and participatory approaches to research and innovation can help build trust, facilitate knowledge co-creation, and ensure that the benefits of these technologies are equitably distributed. The establishment of public-private partnerships, citizen science initiatives, and stakeholder engagement platforms can foster a more inclusive and democratic approach to the development and governance of these technologies.
The future of biotechnology and AI integration holds immense promise for addressing some of humanity’s most pressing challenges. By harnessing the power of these technologies and fostering interdisciplinary collaboration, we can unlock new frontiers in scientific discovery, technological innovation, and societal progress. However, realizing this potential will require concerted efforts to address the technical, ethical, and societal challenges that lie ahead. We must approach these challenges with a sense of responsibility, ensuring that the development and deployment of these technologies are guided by the principles of fairness, transparency, and the greater good (Stilgoe et al., 2013).
As we move forward, investing in education and public outreach is crucial to foster a better understanding of the potential benefits and risks of biotechnology and AI integration. Engaging the public in meaningful dialogue and decision-making processes can help build trust and ensure that the development of these technologies aligns with societal values and priorities. Moreover, supporting interdisciplinary research and training programs can help develop the next generation of scientists, engineers, and policymakers with the skills and knowledge to navigate the complex landscape of biotechnology and AI integration.
In conclusion, the future outlook for the intersection of biotechnology and AI is exciting and challenging. As we stand at the threshold of a new era of scientific and technological advancement, it is our collective responsibility to ensure that we harness the power of these technologies for the benefit of all humanity. By fostering interdisciplinary collaboration, engaging in public dialogue, and prioritizing ethical considerations, we can create a future in which the integration of biotechnology and AI leads to a more healthy, sustainable, and equitable world. Let us embrace this extraordinary opportunity with a purpose guided by innovation, responsibility, and the pursuit of knowledge for the greater good.
Conclusions
As we stand at the precipice of a new era, the intersection of biotechnology and artificial intelligence beckons us to embrace its transformative potential. The convergence of these two powerful fields promises to revolutionize how we approach humanity’s most pressing challenges, from healthcare and agriculture to environmental conservation and beyond (Topol, 2019).
The advancements in biomedical applications, such as AI-driven drug discovery, personalized medicine, and regenerative medicine, offer hope for millions suffering from diseases that have long eluded effective treatments (Vamathevan et al., 2019). Integrating AI and biotechnology in agriculture and environmental management presents a path towards sustainable food production and ecosystem restoration, ensuring a healthier planet for future generations (Akhtar et al., 2020).
However, as we embrace the possibilities offered by this intersection, we must navigate the ethical and societal considerations that arise. We must strive for equitable access to these transformative technologies, remain vigilant against biases and privacy erosion, and engage in ongoing public dialogue to shape our desired future (Mehrabi et al., 2021).
The journey ahead is filled with challenges and boundless opportunities. By fostering interdisciplinary collaboration and engaging diverse stakeholders, we can unlock the full potential of biotechnology and AI integration. Through cooperation, innovation, and responsibility, we can harness the power of these technologies to improve the human condition and create a brighter future for all (Stilgoe et al., 2013).
As we embark on this exhilarating journey, let us embrace the possibilities with open minds and hearts, guided by a steadfast commitment to the greater good. The intersection of biotechnology and AI represents a watershed moment in human history, a chance to rewrite the narrative of our species and shape a future that surpasses our wildest dreams.
In the words of Carl Sagan, “Somewhere, something incredible is waiting to be known.” The intersection of biotechnology and artificial intelligence is “something incredible,” a frontier of boundless potential waiting to be explored and harnessed for the betterment of all. Let us summon the courage, compassion, and wisdom to navigate the challenges and opportunities that lie ahead, guided by innovation, responsibility, and the pursuit of knowledge.
The convergence of biotechnology and AI is a call to action, an invitation to dream big and imagine a world where the most intractable problems yield to the power of human ingenuity and collaboration. It is a reminder that, despite our differences, we are united by a common purpose: to leave this world better than we found it.
So, let us answer this call with enthusiasm and determination, forging ahead toward a future where the miracles of science and technology are harnessed for the benefit of all humanity. Let us always remember that the accurate measure of our success will be the depth of our compassion, the strength of our unity, and the enduring legacy we leave for generations to come.
References
Agustí, A., Montserrat, J. M., Zubrow, M., Gozal, D., & Farre, R. (2020). Deep learning and sleep apnea: We are not there yet. Journal of Clinical Sleep Medicine, 16(10), 1613-1614.
Akhtar, M., Sultana, S., Awais, M., Akram, W., Owusu-Dabo, E., & Hogarh, J. N. (2020). Artificial intelligence in precision agriculture: Techniques, applications, challenges, and prospects. In C. Raju (Ed.), Precision Environmental Agriculture (pp. 217–246). Springer, Cham.
Alyass, A., Turcotte, M., & Meyre, D. (2015). From big data analysis to personalized medicine for all: Challenges and opportunities. BMC Medical Genomics, 8(1), Article 33. https://doi.org/10.1186/s12920-015-0108-y
Angermueller, C., Pärnamaa, T., Parts, L., & Stegle, O. (2016). Deep learning for computational biology. Molecular Systems Biology, 12(7), Article 878. https://doi.org/10.15252/msb.20156651
Arrieta, A. B., Díaz-Rodríguez, N., Del Ser, J., Bennetot, A., Tabik, S., Barbado, A., García, S., Gil-López, S., Molina, D., Benjamins, R., Chatila, R., & Herrera, F. (2020). Explainable Artificial Intelligence (XAI): Concepts, taxonomies, opportunities, and challenges toward responsible AI. Information Fusion, 58, 82–115. https://doi.org/10.1016/j.inffus.2019.12.012
Badrinarayanan, V., Kendall, A., & Cipolla, R. (2017). Segnet: A deep convolutional encoder-decoder architecture for image segmentation. IEEE Transactions on Pattern Analysis and Machine Intelligence, 39(12), 2481-2495. https://doi.org/10.1109/TPAMI.2016.2644615
Bai, L., Wu, Y., Li, G., Zhang, W., Zhang, H., & Su, J. (2023). AI-enabled organoids: Construction, analysis, and application. Bioactive materials, pp. 31, 525–548. https://doi.org/10.1016/j.bioactmat.2023.09.005
Blasimme, A., & Vayena, E. (2020). The ethics of AI in biomedical research, patient care, and public health. In M. D. Dubber, F. Pasquale, & S. Das (Eds.), The Oxford Handbook of Ethics of AI (pp. 703-718). Oxford University Press. https://doi.org/10.1093/oxfordhb/9780190067397.013.43
Brey, P. (2005). Freedom and privacy in ambient intelligence. Ethics and Information Technology, 7(3), 157–166. https://doi.org/10.1007/s10676-006-0005-3
Brownstein, J. S., Freifeld, C. C., & Madoff, L. C. (2009). Digital disease detection—harnessing the Web for public health surveillance. New England Journal of Medicine, 360(21), 2153–2157. https://doi.org/10.1056/NEJMp0900702
Brynjolfsson, E., Mitchell, T., & Rock, D. (2018). What can machines learn, and what does it mean for occupations and the economy? AEA Papers and Proceedings, 108, 43-47. https://doi.org/10.1257/pandp.20181019
Cao, Y., Romero, J., Olson, J. P., Degroote, M., Johnson, P. D., Kieferová, M., Kivlichan, I. D., Menke, T., Peropadre, B., Sawaya, N. P. D., Sim, S., Veis, L., & Aspuru-Guzik, A. (2019). Quantum chemistry in the age of quantum computing. Chemical Reviews, 119(19), 10856-10915. https://doi.org/10.1021/acs.chemrev.8b00803
Carbonell, P., Jervis, A. J., Robinson, C. J., Yan, C., Dunstan, M., Swainston, N., Vinaixa, M., Hollywood, K. A., Currin, A., Rattray, N. J. W., Taylor, S., Spiess, R., Sung, R., Williams, A. R., Fellows, D., Stanford, N. J., Mulherin, P., Le Feuvre, R., Barran, P., . . . Scrutton, N. S. (2018). An automated Design-Build-Test-Learn pipeline for enhanced microbial production of fine chemicals. Communications Biology, 1(1), Article 66. https://doi.org/10.1038/s42003-018-0076-9
Chaudhary, B., & Kumar, V. (2022). Emerging technological frameworks for sustainable agriculture and environmental management. Sustainable Horizons, p. 3, 100026. https://www.sciencedirect.com/science/article/pii/S2772737822000219
Chen, R., & Snyder, M. (2013). The promise of personalized omics to precision medicine. Wiley Interdisciplinary Reviews: Systems Biology and Medicine, 5(1), 73-82. https://doi.org/10.1002/wsbm.1198
Christin, S., Hervet, É., & Lecomte, N. (2019). Applications for deep learning in ecology. Methods in Ecology and Evolution, 10(10), 1632-1644. https://doi.org/10.1111/2041-210X.13256
Clomburg, J. M., Crumbley, A. M., & Gonzalez, R. (2017). Industrial biomanufacturing: The future of chemical production. Science, 355(6320), Article aag0804. https://doi.org/10.1126/science.aag0804
Corlett, R. T. (2017). A bigger toolbox: Biotechnology in biodiversity conservation. Trends in Biotechnology, 35(1), 55-65. https://doi.org/10.1016/j.tibtech.2016.06.009
Dixit, S., Kumar, A., Srinivasan, K., Vincent, P. M. D. R., & Ramu Krishnan, N. (2024). Advancing genome editing with artificial intelligence: opportunities, challenges, and future directions. Frontiers in bioengineering and biotechnology, p. 11, 1335901. https://doi.org/10.3389/fbioe.2023.1335901
Edington, C. D., Chen, W. L., Geishecker, E., Kassis, T., Soenksen, L. R., Bhushan, B. M., Freake, D., Kirschner, J., Maass, C., Tsamandouras, N., Valdez, J., Cook, C. D., Parent, T., Snyder, S., Yu, J., Suter, E., Shockley, M., Velazquez, J., Velazquez, J. J., . . . Ingber, D. E. (2018). Interconnected microphysiological systems for quantitative biology and pharmacology studies. Scientific Reports, 8(1), Article 4530. https://doi.org/10.1038/s41598-018-22749-0
Ekins, S., Puhl, A. C., Zorn, K. M., Lane, T. R., Russo, D. P., Klein, J. J., Hickey, A. J., & Clark, A. M. (2019). Exploiting machine learning for end-to-end drug discovery and development. Nature Materials, 18(5), 435-441. https://doi.org/10.1038/s41563-019-0338-z
Eraslan, G., Avsec, Ž., Gagneur, J., & Theis, F. J. (2019). Deep learning: New computational modeling techniques for genomics. Nature Reviews Genetics, 20(7), 389-403. https://doi.org/10.1038/s41576-019-0122-6
Floridi, L., Cowls, J., Beltrametti, M., Chatila, R., Chazerand, P., Dignum, V., Luetge, C., Madelin, R., Pagallo, U., Rossi, F., Schafer, B., Valcke, P., & Vayena, E. (2018). AI4People—An ethical framework for a good AI society: Opportunities, risks, principles, and recommendations. Minds and Machines, 28(4), 689-707. https://doi.org/10.1007/s11023-018-9482-5
Frank, M. R., Autor, D., Bessen, J. E., Brynjolfsson, E., Cebrian, M., Deming, D. J., Feldman, M., Groh, M., Lobo, J., Moro, E., Wang, D., Youn, H., & Rahwan, I. (2019). Toward understanding the impact of artificial intelligence on labor. Proceedings of the National Academy of Sciences, 116(14), 6531-6539. https://doi.org/10.1073/pnas.1900949116
Gawehn, E., Hiss, J. A., & Schneider, G. (2016). Deep learning in drug discovery. Molecular Informatics, 35(1), 3-14. https://doi.org/10.1002/minf.201501008
General Data Protection Regulation (GDPR). (2016). Regulation (EU) 2016/679 of the European Parliament and of the Council of 27 April 2016 on the protection of natural persons with regard to the processing of personal data and on the free movement of such data, and repealing Directive 95/46/EC (General Data Protection Regulation). Official Journal of the European Union, L119, pp. 1–88.
Gilbert, J. A., Jansson, J. K., & Knight, R. (2014). The Earth Microbiome Project: Successes and aspirations. BMC Biology, p. 12, Article 69. https://doi.org/10.1186/s12915-014-0069-1
Hare, J. I., Lammers, T., Ashford, M. B., Puri, S., Storm, G., & Barry, S. T. (2017). Challenges and strategies in anti-cancer nanomedicine development: An industry perspective. Advanced Drug Delivery Reviews, pp. 108, 25–38. https://doi.org/10.1016/j.addr.2016.04.025
Huntingford, C., Jeffers, E. S., Bonsall, M. B., Christensen, H. M., Lees, T., & Yang, H. (2019). Machine learning and artificial intelligence to aid climate change research and preparedness. Environmental Research Letters, 14(12), Article 124007. https://doi.org/10.1088/1748-9326/ab4e55
Jansson, J. K., & Hofmockel, K. S. (2020). Soil microbiomes and climate change. Nature Reviews Microbiology, 18(1), 35-46. https://doi.org/10.1038/s41579-019-0265-7
Japkowicz, N., & Shah, M. (2011). Evaluating learning algorithms: A classification perspective. Cambridge University Press. https://doi.org/10.1017/CBO9780511921803
Joppa, L. N., O’Connor, B., Visconti, P., Smith, C., Geldmann, J., Hoffmann, M., Watson, J. E. M., Butchart, S. H. M., Virah-Sawmy, M., Halpern, B. S., Ahmed, S. E., Balmford, A., Sutherland, W. J., Harfoot, M., Hilton-Taylor, C., Foden, W., Di Minin, E., Pagad, S., Genovesi, P., . . . Burgess, N. D. (2016). Filling in biodiversity threat gaps. Science, 352(6284), 416–418. https://doi.org/10.1126/science.aaf3565
Kadurin, A., Nikolenko, S., Khrabrov, K., Aliper, A., & Zhavoronkov, A. (2017). druGAN: An advanced generative adversarial autoencoder model for de novo generation of new molecules with desired molecular properties in silico. Molecular Pharmaceutics, 14(9), 3098-3104. https://doi.org/10.1021/acs.molpharmaceut.7b00346
Kaushik, A. K., Jayant, R. D., Tiwari, S., Vashist, A., & Nair, M. (2016). Nano-biosensors to detect beta-amyloid for Alzheimer’s disease management. Biosensors and Bioelectronics, 80, 273-287. https://doi.org/10.1016/j.bios.2016.01.065
Korinek, A., & Stiglitz, J. E. (2021). Artificial intelligence, globalization, and strategies for economic development (NBER Working Paper No. 28453). National Bureau of Economic Research. https://doi.org/10.3386/w28453
LeCun, Y., Bengio, Y., & Hinton, G. (2015). Deep learning. Nature, 521(7553), 436–444. https://doi.org/10.1038/nature14539
Leung, M. K., Delong, A., Alipanahi, B., & Frey, B. J. (2016). Machine learning in genomic medicine: A review of computational problems and data sets. Proceedings of the IEEE, 104(1), 176-197. https://doi.org/10.1109/JPROC.2015.2494198
Li, J., Pu, Y., Tang, J., Feng, B., Guo, W., & He, B. (2021). Machine learning in translational medicine: Bringing the future to the clinic. Trends in Biotechnology. Advanced online publication. https://doi.org/10.1016/j.tibtech.2021.08.005
Loboguerrero, A. M., Thornton, P., Wadsworth, J., Campbell, B. M., Herrero, M., Mason-D’Croz, D., Dinesh, D., Huyer, S., Jarvis, A., Millan, A., Wollenberg, E., & Zebiak, S. (2021). Perspective article: Actions to reconfigure food systems. Global Food Security, p. 27, Article 100432. https://doi.org/10.1016/j.gfs.2020.100432
Makridakis, S. (2017). The forthcoming Artificial Intelligence (AI) revolution: Its impact on society and firms. Futures, 90, 46-60. https://doi.org/10.1016/j.futures.2017.03.006
Mamoshina, P., Vieira, A., Putin, E., & Zhavoronkov, A. (2016). Applications of deep learning in biomedicine. Molecular Pharmaceutics, 13(5), 1445-1454. https://doi.org/10.1021/acs.molpharmaceut.5b00982
Mandai, M., Watanabe, A., Kurimoto, Y., Hirami, Y., Morinaga, C., Daimon, T., Fujihara, M., Akimaru, H., Sakai, N., Shibata, Y., Terada, M., Nomiya, Y., Tanishima, S., Nakamura, M., Kamao, H., Sugita, S., Onishi, A., Ito, T., Fujita, K., . . . Takahashi, M. (2017). Autologous induced stem-cell-derived retinal cells for macular degeneration. New England Journal of Medicine, 376(11), 1038-1046. https://doi.org/10.1056/NEJMoa1608368
Mardis, E. R. (2017). DNA sequencing technologies: 2006–2016. Nature Protocols, 12(2), 213-218. https://doi.org/10.1038/nprot.2016.182
Mehrabi, N., Morstatter, F., Saxena, N., Lerman, K., & Galstyan, A. (2021). A survey on bias and fairness in machine learning. ACM Computing Surveys, 54(6), Article 115. https://doi.org/10.1145/3457607
Merk, D., Friedrich, L., Grisoni, F., & Schneider, G. (2018). De novo design of bioactive small molecules by artificial intelligence. Molecular Informatics, 37(1-2), Article 1700153. https://doi.org/10.1002/minf.201700153
Montesinos-López, O. A., Montesinos-López, A., Crossa, J., Toledo, F. H., Pérez-Hernández, O., Eskridge, K. M., & Rutkoski, J. (2016). A genomic Bayesian multi-trait and multi-environment model. G3: Genes, Genomes, Genetics, 6(9), 2725-2744. https://doi.org/10.1534/g3.116.032359
Nguyen, P. Q., Soenksen, L. R., Donghia, N. M., Angenent-Mari, N. M., de Puig, H., Huang, A., Lee, R., Slomovic, S., Galbersanini, T., Lansberry, G., Sallum, H. M., Zhao, E. M., Niemi, J. B., & Collins, J. J. (2021). Wearable materials with embedded synthetic biology sensors for biomolecule detection. Nature Biotechnology, 39(11), 1366-1374. https://doi.org/10.1038/s41587-021-00950-3
Panch, T., Szolovits, P., & Atun, R. (2018). Artificial intelligence, machine learning, and health systems. Journal of Global Health, 8(2), Article 020303. https://doi.org/10.7189/jogh.08.020303
Pastorino, R., De Vito, C., Migliara, G., Glocker, K., Binenbaum, I., Ricciardi, W., & Boccia, S. (2019). Benefits and challenges of Big Data in healthcare: An overview of the European initiatives. European Journal of Public Health, 29(Suppl. 3), 23-27. https://doi.org/10.1093/eurpub/ckz168
Piaggio, A. J., Segelbacher, G., Seddon, P. J., Alphey, L., Bennett, E. L., Carlson, R. H., Friedman, R. M., Kanavy, D., Phelan, R., Redford, K. H., Rosales, M., Slobodian, L., & Wheeler, K. (2017). Is it time for synthetic biodiversity conservation? Trends in Ecology & Evolution, 32(2), 97–107. https://doi.org/10.1016/j.tree.2016.10.016
Popova, M., Isayev, O., & Tropsha, A. (2018). Deep reinforcement learning for de novo drug design. Science Advances, 4(7), Article eaap7885. https://doi.org/10.1126/sciadv.aap7885
Quer, J., Colomer-Castell, S., Campos, C., Andrés, C., Piñana, M., Cortese, M. F., González-Sánchez, A., Garcia-Cehic, D., Ibáñez, M., Pumarola, T., Rodríguez-Frías, F., Antón, A., & Tabernero, D. (2022). Next-Generation Sequencing for Confronting Virus Pandemics. Viruses, 14(3), 600. https://doi.org/10.3390/v14030600
Rajkomar, A., Hardt, M., Howell, M. D., Corrado, G., & Chin, M. H. (2018). Ensuring fairness in machine learning to advance health equity. Annals of Internal Medicine, 169(12), 866-872. https://doi.org/10.7326/M18-1990
Ristevski, B., & Chen, M. (2018). Big data analytics in medicine and healthcare. Journal of Integrative Bioinformatics, 15(3), Article 20170030. https://doi.org/10.1515/jib-2017-0030
Rolnick, D., Donti, P. L., Kaack, L. H., Kochanski, K., Lacoste, A., Sankaran, K., Ross, A. S., Milojevic-Dupont, N., Jaques, N., Waldman-Brown, A., Luccioni, A., Maharaj, T., Sherwin, E. D., Mukkavilli, S. K., Kording, K. P., Gomes, C., Ng, A. Y., Hassabis, D., Platt, J. C., . . . Bengio, Y. (2022). Tackling climate change with machine learning. ACM Computing Surveys, 55(2), Article 42. https://doi.org/10.1145/3485128
Sadowski, J., & Pasquale, F. (2015). The spectrum of control: A social theory of the smart city. First Monday, 20(7). https://doi.org/10.5210/fm.v20i7.5903
Schork, N. J. (2015). Personalized medicine: Time for one-person trials. Nature, 520(7549), 609-611. https://doi.org/10.1038/520609a
Sedlazeck, F. J., Lee, H., Darby, C. A., & Schatz, M. C. (2018). Piercing the dark matter: Bioinformatics of long-range sequencing and mapping. Nature Reviews Genetics, 19(6), 329-346. https://doi.org/10.1038/s41576-018-0003-4
Senior, A. W., Evans, R., Jumper, J., Kirkpatrick, J., Sifre, L., Green, T., Qin, C., Žídek, A., Nelson, A. W. R., Bridgland, A., Penedones, H., Petersen, S., Simonyan, K., Crossan, S., Kohli, P., Jones, D. T., Silver, D., Kavukcuoglu, K., & Hassabis, D. (2020). Improved protein structure prediction using potentials from deep learning. Nature, 577(7792), 706-710. https://doi.org/10.1038/s41586-019-1923-7
Sharma, T. K., Ramanathan, R., Rakwal, R., Agrawal, G. K., & Bansal, V. (2015). Moving forward in plant food safety and security through NanoBioSensors: Adopt or adapt biomedical technologies? Proteomics, 15(10), 1680-1692. https://doi.org/10.1002/pmic.201400503
Shendure, J., Balasubramanian, S., Church, G. M., Gilbert, W., Rogers, J., Schloss, J. A., & Waterston, R. H. (2017). DNA sequencing at 40: Past, present, and future. Nature, 550(7676), 345-353. https://doi.org/10.1038/nature24286
Slomovic, S., Pardee, K., & Collins, J. J. (2015). Synthetic biology devices for in vitro and in vivo diagnostics. Proceedings of the National Academy of Sciences, 112(47), 14429-14435. https://doi.org/10.1073/pnas.1508521112
Sontheimer-Phelps, A., Hassell, B. A., & Ingber, D. E. (2019). Modeling cancer in microfluidic human organs-on-chips. Nature Reviews Cancer, 19(2), 65-81. https://doi.org/10.1038/s41568-018-0104-6
Stephens, Z. D., Lee, S. Y., Faghri, F., Campbell, R. H., Zhai, C., Efron, M. J., Iyer, R., Schatz, M. C., Sinha, S., & Robinson, G. E. (2015). Big data: Astronomical or genomical? PLOS Biology, 13(7), Article e1002195. https://doi.org/10.1371/journal.pbio.1002195
Stieb, D. M., Huang, A., Hocking, R., Crouse, D. L., Osornio-Vargas, A. R., & Villeneuve, P. J. (2019). Using maps to communicate environmental exposures and health risks: Review and best-practice recommendations. Environmental Research, 176, Article 108518. https://doi.org/10.1016/j.envres.2019.05.049
Stilgoe, J., Owen, R., & Macnaghten, P. (2013). Developing a framework for responsible innovation. Research Policy, 42(9), 1568-1580. https://doi.org/10.1016/j.respol.2013.05.008
Stokes, J. M., Yang, K., Swanson, K., Jin, W., Cubillos-Ruiz, A., Donghia, N. M., MacNair, C. R., French, S., Carfrae, L. A., Bloom-Ackermann, Z., Tran, V. M., Chiappino-Pepe, A., Badran, A. H., Andrews, I. W., Chory, E. J., Church, G. M., Brown, E. D., Jaakkola, T. S.,
Tabak, M. A., Norouzzadeh, M. S., Wolfson, D. W., Sweeney, S. J., VerCauteren, K. C., Snow, N. P., Halseth, J. M., Di Salvo, P. A., Lewis, J. S., White, M. D., Teton, B., Beasley, J. C., Schlichting, P. E., Boughton, R. K., Wight, B., Newkirk, E. S., Ivan, J. S., Odell, E. A., Brook, R. K., . . . Miller, R. S. (2019). Machine learning to classify animal species in camera trap images: Applications in ecology. Methods in Ecology and Evolution, 10(4), 585–590. https://doi.org/10.1111/2041-210X.13120
Takahashi, K., & Yamanaka, S. (2006). Induction of pluripotent stem cells from mouse embryonic and adult fibroblast cultures by defined factors. Cell, 126(4), 663-676. https://doi.org/10.1016/j.cell.2006.07.024
Tomczak, K., Czerwińska, P., & Wiznerowicz, M. (2015). The Cancer Genome Atlas (TCGA): An immeasurable source of knowledge. Contemporary Oncology, 19(1A), A68-A77. https://doi.org/10.5114/wo.2014.47136
Topol, E. J. (2019). High-performance medicine: The convergence of human and artificial intelligence. Nature Medicine, 25(1), 44–56. https://doi.org/10.1038/s41591-018-0300-7
Trounson, A., & McDonald, C. (2015). Stem cell therapies in clinical trials: Progress and challenges. Cell Stem Cell, 17(1), 11–22. https://doi.org/10.1016/j.stem.2015.06.007
Vamathevan, J., Clark, D., Czodrowski, P., Dunham, I., Ferran, E., Lee, G., Li, B., Madabhushi, A., Shah, P., Spitzer, M., & Zhao, S. (2019). Applications of machine learning in drug discovery and development. Nature Reviews Drug Discovery, 18(6), 463–477. https://doi.org/10.1038/s41573-019-0024-5
Vayena, E., Blasimme, A., & Cohen, I. G. (2018). Machine learning in medicine: Addressing ethical challenges. PLOS Medicine, 15(11), Article e1002689. https://doi.org/10.1371/journal.pmed.1002689
Vayena, E., Dzenowagis, J., Brownstein, J. S., & Sheikh, A. (2018). Policy implications of big data in the health sector. Bulletin of the World Health Organization, 96(1), 66–68. https://doi.org/10.2471/BLT.17.197426
Visan, A. I., & Negut, I. (2024). Integrating Artificial Intelligence for Drug Discovery in the Context of Revolutionizing Drug Delivery. Life (Basel, Switzerland), 14(2), 233. https://doi.org/10.3390/life14020233
Xu, Y., Dai, Z., Chen, F., Gao, S., Pei, J., & Lai, L. (2015). Deep learning for drug-induced liver injury. Journal of Chemical Information and Modeling, 55(10), 2085-2093. https://doi.org/10.1021/acs.jcim.5b00238
[1] Figure 7-1: (Beach Flight [Photograph]. U.S. Department of Defense. https://www.defense.gov/Multimedia/Photos/igphoto/2003379433/ )
[2] Figure 7-2: (Fiery Flight [Photograph]. U.S. Department of Defense. https://www.defense.gov/Multimedia/Photos/igphoto/2003458267/ )
[3] Figure 7-3: (https://www.army.mil/article/232346/army_modernizes_its_biometric_processing_capabilities )
[4] Figure 7-4: (Haboob Havoc [Photograph]. U.S. Department of Defense. https://www.defense.gov/Multimedia/Photos/igphoto/2003452285/ )
[5] Figure 7-5: (Hot Work [Photograph]. U.S. Department of Defense. https://www.defense.gov/Multimedia/Photos/igphoto/2003429492/ )