8 Leveraging Advanced Technologies to Enhance Food Production (Larson)
Student learning objectives
- Understand how challenges in the agricultural industry limit food production.
- Identify various strategies for improving food production
- Explain how the adoption of advanced technologies in the field of agriculture is demonstrating improvements in food production
Introduction
As the population of the world continues to increase at an annual rate of approximately 1.07% with no matched increase in available agricultural land, new ways to increase food production must be implemented (Khan et al., 2021). Given the fact that the world’s 150 million square kilometers of land surface will not be drastically changing in size, the productivity and efficiency by which food is produced must be improved. However, factors of climate, increased food demand, and limited water place limits on the amount of food traditional agricultural practices can supply. Therefore, advanced technologies are being implemented into food production systems to target inefficiencies in the system that could lead to increased food production to match the growing world population. Future technology to be implemented into agricultural practices can have a wide range of classifications, including digital agriculture, gene technology, intensified food production systems, and replacements of traditional foods/feed with novel ingredients. In a review of advanced technologies for use in agriculture (Khan et al., 2021), digital agriculture was found to have the most extraordinary breadth of impact across all aspects of the food supply chain (Table 8-1).
Table 8-1: Digital agriculture technologies with transformative potential in agriculture to cover the whole food production system.
Technology | Production | Processing | Packaging | Distribution | Consumption | Waste |
Drones | ✓ | ✓ | ✓ | ✓ | ||
Innovative sensors | ✓ | ✓ | ✓ | ✓ | ✓ | |
Big data | ✓ | ✓ | ✓ | ✓ | ✓ | ✓ |
Artificial intelligence | ✓ | ✓ | ✓ | ✓ | ✓ | ✓ |
Data integration | ✓ | |||||
Disease/pest early warning | ✓ | ✓ | ||||
Robotics | ✓ | ✓ | ✓ | ✓ | ✓ | |
On-field robots | ✓ | ✓ | ||||
Sensors for soil | ✓ | ✓ | ✓ | ✓ | ||
Tracking tech for livestock | ✓ | ✓ | ||||
Farm-to-farm virtual market | ✓ | ✓ | ✓ | ✓ | ✓ | |
Internet of Things | ✓ | ✓ | ✓ | ✓ | ✓ | ✓ |
Improved climate forecasts | ✓ | ✓ | ||||
Nano-drones | ✓ | ✓ | ✓ | ✓ | ||
SERS sensors | ✓ | ✓ | ✓ | ✓ | ||
Pest control robotics | ✓ | ✓ | ||||
Nanotechnology | ✓ | ✓ | ✓ | ✓ | ||
Intelligent food packaging | ✓ | ✓ | ✓ | ✓ | ||
Smartphone food diagnostics | ✓ | ✓ | ✓ | |||
Omics data use | ✓ | |||||
Traceability technologies | ✓ | ✓ | ✓ | ✓ |
Source: (Khan et al., 2021)
When efficiency in agriculture is targeted, the entire supply chain must be considered. However, production has often been the first segment identified for improvement because of ease of implementation. Because of this production segment focus, there have been significant improvements in the efficiency of global food production. For the remainder of this chapter, the discussion will focus on the production segment of the food system.
In 1940, the total agricultural land required to produce food for one individual human was 1.55 hectares (Roser, 2024). By 2020, improvement in the efficiency of food production has drastically changed that value to 0.6 hectares per 1 individual human. A greater than 60% reduction in land required per person is created in part due to the adoption of new technologies, but this pace of improved efficiency is not sustainable. Ortiz-Bobea et al. (2021) demonstrated through a series of 200 simulations that although agricultural advancements have improved the efficiency of food production, global farming productivity decreased by 21% compared to scenarios without global warming.
Challenges in Agriculture and Food Security
The growing number of humans on the planet continues to be a strong motivator for improved productivity in the agricultural industries. Despite the drive to improve, the industry is presented with various challenges that must be addressed in order to be successful in increasing global food production. Common challenges recognized globally for agriculture include (Devlet et al., 2021):
- Population growth
- Changes in consumer behavior
- Destruction of resources by pest infestation
- Shortage of water resources
- Climate change
- Labor costs/availability
Many of these challenges have plagued agriculture far before “modern times.” However, the advantage of modern agriculture is that technology development allows food producers to combat these challenges by strategically targeting various aspects of food production with specified advanced technological solutions. Throughout this chapter, these strategies, as well as corresponding examples of advanced technological solutions, will be presented.
Active strategies to improve agricultural productivity
Some of the strategies that have been identified to aid farmers in improving their agricultural practices include:
Soil health practices
When soil nutrients and water holding capacity are understood, practices for improving yield while minimizing unnecessary inputs like fertilizer or irrigation are possible.
Genetic Improvement
High-quality seed stock selected for specific performance and health traits can help farmers ensure that the species (whether plant or animal) selected for food production on their farm is best suited to the environment and goals of the operation.
Production Frontier
The production frontier is the production possibility for that producer’s operation. It represents the unique circumstances and inputs for that farming operation. Production frontiers are expected to differ according to the geographic region and agricultural potential of the land evaluated.
Mechanization and automation
The use of machines or automatic devices in farming practices presents the opportunity to combat the labor shortage challenges that exist in agriculture and the costs and inefficiencies associated with reliance on human labor.
Appropriate timing
In order for a crop or animal to grow appropriately in a way that increases food production, suitable materials must be available, the proper application/work must be conducted at the right time, and the appropriate nutrients/ingredients must be leveraged to support food growth and production. Timing can be influenced by a multitude of factors – labor, weather, supply chains, etc.
Irrigation systems
Water is one of the most valuable resources when it comes to food production, especially in certain parts of the world. Effective use of water resources ensures that food production can persist in agricultural regions for years to come and can contribute to improved yield outcomes.
Integrated pest management
Integrated pest management (IPM) is a proactive approach to avoid, identify, and treat disease-related events as they occur on both plants and animals. This could be either a pest presence or a disease outbreak. Minimizing the overuse of pesticides and drugs helps ensure pest resistance to the product doesn’t develop at an unexpected rate. It reduces input costs while still controlling the welfare and health of plants and animals used for food production.
Data Analytics
Data analytics often involves the use of an integrated platform or software that can interpret data inputs from the food production system in a way that generates meaningful insights in real-time. Data inputs can include sensors, scales, images, and other collected data pieces that, when integrated, provide the farmer with a big-picture overview of the operation and potential areas that need to be addressed within the farm.
Technical Efficiency
Technical efficiency is defined as the achievement of maximum productivity that the technology implemented can allow. Each of the strategies identified in this section has limitations for the final impact it can have on agricultural production – that is, the technical efficiency of that strategy.
For the remainder of this chapter, various case studies of applied advanced technologies will be presented as they support these strategies for the improvement of agricultural productivity.
What is digital agriculture?
Digital agriculture is defined as “the ongoing transformation of farming that includes digitalization and automation of farming tasks” (McFadden et al., 2023). This includes the adoption of yield maps and soil maps generated using sensor and satellite technologies, variable rate technologies, automated guidance for equipment, drone applicators and mounted sensors, and other advanced technologies. The field of digital agriculture is constantly changing and casts a broad perspective on the integration of various advanced technologies into agriculture for the improvement of efficiency in food production. In the remainder of this chapter, multiple highlights will be presented as examples of advanced technologies that have been integrated into agricultural food production systems. This is intended as an introduction to some of the unique advancements that have been made but is by no means an exhaustive list of all the cutting-edge technologies with potential applications for enhancing food production.
Sensor-generated maps for improved efficiency of natural resources
Knowledge is power. This age-old phrase especially holds with regard to how food producers interpret and examine the natural resources they have available for food production. Soil nutrient composition and water holding capacity are often evaluated to understand growing capacity and additional needs to maximize crop yields. Grass and forage samples are collected and analyzed for nutrient composition to know if that ingredient will meet nutrient requirements for grazing livestock’s health and growth. All these techniques provide food producers with knowledge. Traditionally, each data value has been analyzed independently, whether analyzed via in-hand sensors or sent off to a lab for testing. The expertise of food producers means they understand how to integrate these various data points into something meaningful. Recently, advanced technologies have been working to incorporate multiple sensors, lab measurements, and images into a centralized platform to help producers make real-time decisions via visual presentation of their resources. These generated maps allow ease of decision-making and lower the expertise requirement for entry-level food producers to effectively increase yields without many years of trial and error experienced by their senior food producers.
A recent survey of 800 commercial-scale corn and soybean production operations examined how farm data was collected and analyzed among large farms (DeLay et al., 2021). It was found that yield monitors, soil samples, and aerial imagery were commonly implemented practices, with over 40% of operations leveraging a form of ag-data software. The majority of the technology adopters were young professionals of all education levels. Over half (52%) of those food producers using data collection technology used Climate FieldViewTM (Figure 8-1), with John Deere’s Operations Center close behind at 44%.
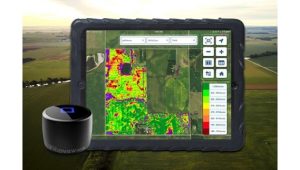
Both of these popular platforms allow for ease of decision-making and real-time data interpretations regarding available resources. John Deere Operations Center focuses on equipment function and operation and how that interrelates to the natural resources. Climate FieldViewTM works as a platform for information collection and data analysis. Multiple equipment makes are compatible with the platform to input collected data on crop performance, field zones for variable rate seedings, crop protection plans, and soil fertility. It intends to be a “one-stop-shop” that leverages machine learning for food production-related decision-making through data collection, storage, and analysis.
Often, when it comes to raising livestock, food producers are not privileged to the same level of knowledge that crop producers are – simply due to the complexity of the variables influencing animal protein production. An area of livestock production that has historically struggled with knowledge/insights for improving the efficiency of food production is animal grazing pastures or native ranges. Grazing animals are classified as prey species, which means that behaviorally, they can be challenging to study when allowed to roam freely in their native environment (which consists of large grass or forage pastures). Recent research has shown that advanced technologies implemented by cattle grazing producers allow for increased knowledge of animal behavior patterns, which can result in improved efficiency in animal protein production and natural resource use. One such example of this was research conducted by Sangjan et al. (2022), which compared satellite and unmanned aerial system (UAS) imagery of forage pastures as an indicator of pasture productivity when grazed by cattle. Vegetation index data was determined using remote sensing and wavelength measurements to measure the green color of vegetation in satellite and UAS images (Figure 8-2). The study concluded that both satellite and UAS images could be leveraged to improve pasture productivity and understand the grazing intensity patterns of cattle.
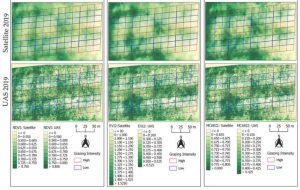
Using advanced technologies to reduce agriculture-affiliated pests
Pests and weeds often present themselves to food producers as a grand challenge, often with a moving target. Pesticides and herbicides have been used historically to eliminate the presence of unwanted organisms that may sequester nutrients away from the plant or animal of interest, creating an inefficiency in the food production system. Pesticides and herbicides were popularized in agriculture around WWII (1939-1945) due to the urgency of increasing food production and controlling insect-borne diseases. It has been estimated that without pesticide use, food production would decrease dramatically (78% loss in fruit production, 54% loss in vegetable production, and 32% loss in cereal production) (Tudi et al., 2021). However, pesticides can be easily overused when spread universally across a field at a consistent application rate. Variable-rate spray technology was developed for integration into precision farming using maps or sensors to detect weeds or soil properties, which then feeds information to AI-driven technology that guides either a smart tractor or drone system to apply a variable amount of pesticide product based on soil and plant needs.
Despite the advancements in variable rate technology applications, pesticides are still viewed negatively for their label indication to kill an organism. Although intended (and registered) as a product to kill, the lack of verified safety of other environmental organisms is often a concern for environmentalists. This has led to continued improvements in pest mitigation for the agricultural industry, mainly through mechanical weed control. Recent technologies have been released that use mounted lasers and autonomous vehicles to eliminate weeds from cropland, eliminating the need for pesticides without increasing labor (Figure 8-3; Andreasen et al., 2022). The laser beams use electricity rather than fossil fuels, and deep learning methods are employed to locate and positively identify weed vs. crop targets for the robot actuator to release laser energy toward. Laser types employed for weed control have been carbon dioxide lasers, diode lasers, and fiber lasers (Andreasen et al., 2022).
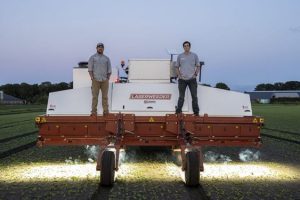
Role of artificial intelligence in pest identification in livestock
Similar to crop food production, the impact of pests represents an inefficiency in animal protein production. Strydom et al., 2023 summarize the impact of gastrointestinal nematodes, liver flukes, and ticks on efficiency in cattle products, stating that parasites not only cost producers money in protein production efficiency but also pose threats to animal wellbeing.
Nematodes are one of the most common parasites that infect cattle globally and are often treated with broad-spectrum parasiticides. However, globally, the overuse of broad-spectrum parasiticides has resulted in a growing resistance to parasiticides on the market by certain nematode species. This has presented new opportunities for diagnostic tools to identify active nematode infections via fecal egg count scores and field training tools such as the FecPakG2 (Figure 8-4). This field microscope has been demonstrated throughout the literature to positively identify when parasiticides are necessary or not within cattle herds (Francis and Slapeta et al., 2022). As it deploys into the hands of cattle producers, data collection has allowed Techion (FecPakG2 developers) to develop artificial intelligence tools for the automated identification of fecal egg counts on farms in real time. This would allow for real-time decisions of when to apply parasiticides, allowing for improved elimination of nematode parasites as well as decreased labor for commercial laboratories that currently identify fecal nematodes by hand via direct identification and enumeration by counting nematode eggs on prepared microscope slides. Corporate sponsors also claim that next-generation field microscope technology will have the ability to diagnose species of nematode infection for prescriptive parasiticide use in the near future.
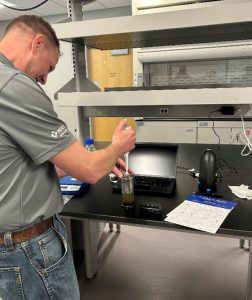
Advanced Technologies that provide alternative labor solutions
Labor continues to present itself as one of the most significant challenges for modern agriculture. In the United States, less than 2% of the population is involved in agriculture. This increases on a global scale, with approximately 27% of people employed in agricultural-affiliated positions. However, the reality remains that the majority of the world’s population is disconnected from food production. This disconnect has decreased the interest of many young professionals in beginning their careers in one of the labor-intensive roles traditionally associated with food production. In an effort to ease young professionals’ entry into the agricultural field, advanced technologies have been implemented explicitly targeting traditionally labor-intensive human tasks.
One of the most prominently recognized ways in which the labor challenge has been addressed with advanced technology is through automation. John Deere has led the market with its implementation of automation technology on various pieces of equipment and has even debuted concepts for completed autonomous tractors (Fig. 8-5). John Deere’s Operations Center software integrates yield monitors and other equipment-mounted sensors to provide feedback to the food producer on areas where efficiency can be improved to increase crop yield.
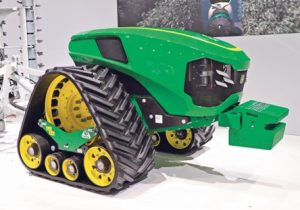
Because of the distance between the general population and food production practices, decision-making has become a common target for technology developers in the agricultural market. The critical importance of food production and the ability of the general population to engage in that process provides business justification for cross-utilization of technologies the general population is familiar with and food production applications for those same platforms. As Microsoft Copilot has continued to grow in popularity, the company has announced they are also developing parallel generative AI for agricultural food production use (Microsoft, 2024). The initial launch is simply for agronomy use but includes image identification and interactive AI decision-making to aid food producers in disease identification and crop yield improvements. Discussions have also begun for livestock production and veterinary medicine applications for Copilot.
Also working in the animal health space are integrated AI and camera technologies for disease and health event identification. OneCup AI was developed by cattle producers to improve the labor intensity of animal care in cattle operations. BETSY is a vision-based 360 Live identification platform that effectively identifies facial recognition and movement in animals for tracking and health event identification (Fig. 8-6; ABP, 2022). So far, BETSY has developed algorithms to identify lameness, coughing, feed intake, growth rate, and calving activities. The integrated cameras used for monitoring feed the AI with data, which then translates to text directly to the cattle producer with any updates they need to be aware of. Capabilities are currently being developed for voice-integrated commands and phone call interaction with BETSY in the near future.
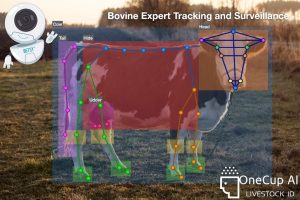
Integrating technologies: Smart aquaculture system example
Aquaculture is often overlooked in the field of agriculture as terrestrial food production is commonly considered the only one. However, with a growing world population and no increase in land mass for agriculture, aquatic food production offers a unique solution to our land-locked challenge of feeding the world. Like any food production system, aquaculture is not without limitations or faults. Traditional aquaculture practices are considered to pose threats to the environment and are very labor intensive – a universal challenge for all of agriculture. “Smart Aquaculture” offers food producers practices that enhance production more sustainably for the environment. Smart Aquaculture is defined by the use of IoT, big data, artificial intelligence, 5G, cloud computing, and robotics in a controlled or automated environment in which aquatic species are reared. These advanced technologies are leveraged to collect data for transmission and subsequent data processing so that it can be applied to critical concepts aquaculturists understand are essential for the health and performance of that species (Figure 8.7; Capetillo-Contreras et al., 2024).
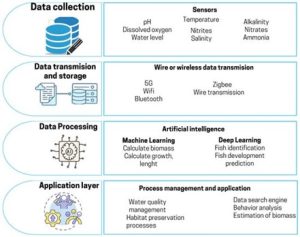
In particular, these intelligent practices have been applied to water quality, environment conditions for the aquatic species, hatchery of young stock, optimized feeding supply, appropriate feeding timing, and reduced need for labor. A conceptual depiction of how these advanced technologies can be incorporated to create an intelligent aquaculture system is shown in Figure 8-8 (Vo et al., 2021; Chrispin et al., 2020). These advanced technologies include the use of AI for feeding devices, AI drones in aquaculture (underwater) for monitoring animal behavior and health, routine checkup/screening techniques using AI technology, and smartphone integration of data analysis review.
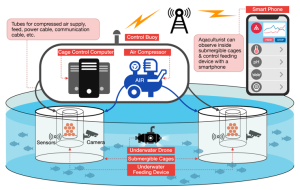
Conclusions
Integrating multiple advanced technology applications into one food production system appears to be a clear direction for new technology development to improve agricultural practices to feed a growing population. The possibilities of advanced technologies appear exponential. The progress made thus far with the various technologies discussed demonstrates success in implementing advanced technologies into food production systems. While the human population continues to increase, food production systems appear prepared to address the challenge by leveraging advanced technologies to improve production efficiency for both crops and livestock.
References
ABP. (2022). Prevision ranching with BETSY. Published by ABP Daily. Retrieved from: https://abpdaily.com/innovation-technology/precision-ranching-with-betsy/ [online resource]
Andreasen, C., Scholle, K., & Saberi, M. (2022). Laser Weeding with small autonomous vehicles: Friends or foes? Frontiers in Agronomy, 4, 841086.
Bayer. (2024). Intelligent Solutions for a Sustainable Future. Published on Bayer Global Digital Farming. Retrieved from: https://www.bayer.com/en/agriculture/digital-farming [online resource]
Becks, P. (2023) Weeding with a Laser, an observation in the field. Published on Future Farming as a weed/pest control article. Retrieved from: https://www.futurefarming.com/crop-solutions/weed-pest-control/weeding-with-a-laser/ [online resource]
Booker, R. (2019). Ag equipment giant unveils the future. Published by the Western Producer as crops, machinery article. Retrieved from: https://www.producer.com/crops/ag-equipment-giant-unveils-the-future/ [online resource]
Capetillo-Contreras, O., Pérez-Reynoso, F. D., Zamora-Antuñano, M. A., Álvarez-Alvarado, J. M., & Rodríguez-Reséndiz, J. (2024). Artificial Intelligence-Based Aquaculture System for Optimizing the Quality of Water: A Systematic Analysis. Journal of Marine Science and Engineering, 12(1), 161.
Chrispin, L. C., Jothiswaran, V. V., Velumani, T., Agnes, D. A. S., & Jayaraman, R. (2020). Application of artificial intelligence in fisheries and aquaculture. Biotica Res Today, 2(6), 499-502.
Codon., L. (2021). Nothing virtual about it: how digital tools are improving farmer’s lives. Published by 3BL CSR WIRE as a news report. Retrieved from: https://www.csrwire.com/press_releases/722681-nothing-virtual-about-it-how-digital-tools-are-improving-farmers-lives [Online resource]
DeLay, N., Mintert, J., & Thompson, N. (2021). Farm Data Collection and Software Adoption in Commercial Scale US Corn-Soybean Farms. In Western Economics Forum (Vol. 19, No. 2, pp. 12-19).
Devlet, A. (2021). Modern agriculture and challenges. Frontiers in Life Sciences and Related Technologies, 2(1), 21-29.
Francis, E. K., & Šlapeta, J. (2022). A new diagnostic approach to fast-track and increase the accessibility of gastrointestinal nematode identification from faeces: FECPAKG2 egg nemabiome metabarcoding. International journal for parasitology, 52(6), 331-342.
Khan, N., Ray, R. L., Kassem, H. S., Hussain, S., Zhang, S., Khayyam, M., Ihtisham., M., & Asongu, S. A. (2021). Potential role of technology innovation in transformation of sustainable food systems: A review. Agriculture, 11(10), 984.
Microsoft. (2024) Generative AI in Azure Data Manager for Agriculture. Published on the Microsoft Learn webpage. Retrieved from: https://learn.microsoft.com/en-us/azure/data-manager-for-agri/concepts-llm-apis [online resource]
McFadden, J., Njuki, E., & Griffin, T. (2023). Precision agriculture in the digital era: recent adoption on US farms.
Ortiz-Bobea, A., Ault, T.R., Carrillo, C.M., Chambers, R.G., & D.B. Lobell. Anthropogenic climate change has slowed global agricultural productivity growth. Nature Climate Change, 2021; DOI: 10.1038/s41558-021-01000-1
Roser, M., (2024). Why is improving agricultural productivity crucial to ending global hunger and protecting the world’s wildlife? Published online at OurWorldInData.org. Retrieved from: ‘https://ourworldindata.org/agricultural-productivity-crucial’ [Online Resource]
Sangjan, W., Carpenter-Boggs, L. A., Hudson, T. D., & Sankaran, S. (2022). Pasture productivity assessment under mob grazing and fertility management using satellite and UAS imagery. Drones, 6(9), 232.
Strydom, T., Lavan, R. P., Torres, S., & Heaney, K. (2023). The economic impact of parasitism from nematodes, trematodes, and ticks on beef cattle production. Animals, 13(10), 1599.
Tudi, M., Daniel Ruan, H., Wang, L., Lyu, J., Sadler, R., Connell, D., Chu, C., & Phung, D. T. (2021). Agriculture development, pesticide application, and its impact on the environment. International journal of environmental research and public health, 18(3), 1112.
Vo, T. T. E., Ko, H., Huh, J. H., & Kim, Y. (2021). Electronics, 10(22), 2882.